Introduction
Wildfire is a primary disturbance phenomenon with 200-500 million hectares burned annually across the globe (Amraoui et al., 2013). Fire affects large areas in almost all kinds of terrestrial ecosystems, so it is a more widespread phenomenon than any other natural disturbance (Ichoku et al., 2008 and Smith et al., 2016). In some cases, e.g. savannas and grasslands, fire plays an ecologically significant role in biogeochemical cycles and disturbance dynamics (Montealegre et al., 2014), influencing the temporal variability in carbon, water, and energy fluxes; however, in other cases, fire may lead to both long-term destruction of the vegetation cover and land degradation. In the European countries of the Mediterranean Basin, fire is a major hazard with an average of 45,000 fires per year which affect 0.5 million hectares (Montealegre et al., 2014 and Quintano et al., 2017).
Fire has a significant effect on both functioning and composition of ecosystems, and in landscape structure, it affects the quality of soil, air and water, and has important impacts on human health, lives and properties (San-Miguel-Ayanz et al., 2103). In Spain, during the last decade (2000-2010), there has been a clear decrease in the number of fires, along with an increase in the occurrence of large fires (>100 ha) (Montealegre et al., 2014 and Eleazar, 2013). Besides, in 2012, 64% of the total burned area corresponded to a single large fire (Montealegre et al., 2014). This increase in the number of large wildfires might be due to an increase in fuel continuity, which in turn is due to changes in land use during recent decades (Pausas et al., 2008). Even though many Mediterranean species exhibit different fire-adaptive strategies, human-induced changes in land use are making many landscapes more vulnerable to high-intensity wildfires (Collins et al., 2013). At a local scale, these effects vary across the landscape depending on factors such as fire intensity, fire and burn severity, fire recurrence and fire return interval (Cocke et al., 2005 and Viedma et al., 2015).
Information about fire effects on ecosystems is useful to ecologists and land managers who want to understand vegetation recovery and succession, and to plan future restoration works (Roy et al., 2006). Besides, fire-induced severity can help to reduce uncertainty in total gas emissions estimations from biomass burning (Holden et al., 2005), water quality determination, and radiation budget assessment (Quintano et al., 2017 and Keeley, 2009). Severity mapping can be useful for highlighting the most affected areas, and explore ecological responses before and after the fire (Chuvieco, 2009). Fire causes significant changes, as vegetation consumption and charring, destruction of leaf chlorophyll, exposure of soil, and alteration of both above- and below-ground moisture (White et al., 1996). Fortunately, most of these changes induce spectral variations that can be captured by some of the available satellite sensors (Chuvieco, 2009; White, 1996 and Lentile et al., 2006). In fact, satellite data has been used for decades to assess many factors related to fire (Lentile et al., 2006).
Within the remote sensing community, severity has been related to vegetation consumption, alteration of soil properties and long-term post-fire vegetation mortality and recovery, among others (Lentile et al., 2006). In particular, fire severity has been traditionally associated with active fire characteristics and immediate post-fire effects; whereas burn severity incorporates both short- and long-term effects (De Santis et al., 2007). For many authors, burn severity is related to the amount of time necessary to return to previous state and functionality (Lentile et al., 2006). In this paper, we focus on burn severity and define it as the way to identify the impact of fire on soil and plants once the fire is extinguished, consequently being related to what is left (post-fire phase) (De Santis et al., 2007).
There is a wide range of remote sensing platforms along with different methods for determining burn severity (Parsons et al., 2010), most of them including the use of post-fire field data (De Santis et al., 2007). Many studies have demonstrated the sensitivity of various spectral bands to significant changes in the radiance of burned vegetation. In particular, visible, near-infrared (NIR), and short-wave infrared (SWIR) channels have been profusely used in the study of fire effects on vegetated areas (White et al., 1996; Miller and Quayle, 2015 and Key and Benson, 2006). NIR reflectance is primarily sensitive to the spongy mesophyll layer at the leaf level (Knipling, 1970), while SWIR to water content, ash cover, and soil mineral content (Chuvieco, 2009 and Miller and Quayle, 2015). On the other hand, reflectance in the visible region depends on chlorophyll content. These bands have been used to study fire effects individually, or summarized in spectral indices of two mainly categories: vegetation or burned area indices. The Normalized Difference Vegetation Index (NDVI) has been related to field measurements of burn severity (Chafer et al., 2004), while the Normalized Burn Ratio (NBR), proposed by Key and Benson (Key and Benson, 2002), is the most effective NIR-SWIR index for burn severity available in the literature (De Santis et al., 2007). These two indices are defined as (Equation 1) (Equation 2):
Where ρNIR and ρRED are the reflectance of NIR and red bands respectively, while ρ4 and ρ7 are the reflectance of band 4 (NIR) and band 7 (SWIR) of Landsat TM.
Since burn severity depends on pre-fire conditions, estimation techniques should rely on a temporal evaluation of the vegetation cover. Consequently, some authors suggested the use of differential indices like the dNDVI (NDVI pre-fire minus NDVI post-fire) or the dNBR (NBR pre-fire minus NBR post-fire), proposed by Key and Benson (Key and Benson, 2002). Using this absolute change detection algorithm, barren areas unchanged by fire would not appear as high severity areas (Key and Benson, 2006). However, the use of differential indices does not solve one of the disadvantages of using continuous variables, which is the ambiguity in the definition of threshold values of severity classes. As shown in Table 1, different dNBR threshold values might correspond to the same field-based severity classes, leading to need of undergoing a calibration process for every new application of the procedure (Miller and Quayle, 2015 and Miller and Thode, 2007).
Table 1 Variability in the definition of the thresholds of burn severity through the dNBR spectral index
Study | Region | Severity level | |||
Unburned | Low | Moderate | High | ||
Parker et al. (2015) | Australia | <77 | 78-257 | 258-427 | >428 |
Miller and Thode (2007) | California, USA | <41 | 41-176 | 177-366 | >367 |
Key and Benson (2006) | South of Canada | <99 | 100-269 | 270-659 | >660 |
Hoscilo et al. (2013) | Indonesia | <53 | 54-213 | 214-550 | >550 |
Montealegre et al. (2014) | Spain | <81 | 82-198 | 199-545 | >545 |
With the objective of overcoming some of the mentioned disadvantages, Miller and Thode (Miller and Thode, 2007) presented a relativized version of dNBR, called the Relative dNBR (RdNBR). An index, as described by Parks et al. (2014), is a metric of relative change (rather than absolute change), thus it emphasizes change relative to the amount of pre-fire vegetation cover. Posteriorly, a new Landsat-based burn severity metric arose: the Relativized Burn Ratio (RBR), which provided an alternative to dNBR and RdNBR; this new index, developed by Parks et al. (2014), showed a higher capability for detection change, even where pre-fire vegetation cover was low. These two indices are defined as (Equation 3) (Equation 4):
Reliable estimations of burn severity are only possible using remotely sensed information in combination with field data. Field estimations of severity are usually based on visual observations of the effects of fire on soil and vegetation, and on the assessment of a set of related parameters (De Santis and Chuvieco, 2009). Among the available methods, the Composite Burn Index (CBI) developed by Key and Benson (Key and Benson, 2002) within the FIREMON (Fire Effects Monitoring and Inventory Protocol) project, and its modified version, the Geometrically structured CBI (GeoCBI) (De Santis and Chuvieco, 2009), have been used widely as operational tools. Both field indices present two main advantages: firstly, they visually assess the magnitude of change in five individual strata (substrate, grasslands/low shrubs, tall shrubs/saplings, understory trees, and canopy trees), and integrate them in a synoptic score (ranging from 0: unburned, to 3: highest severity) for the whole plot area (Key and Benson, 2006 and De Santis and Chuvieco, 2009). Secondly, these indices were designed to be used in conjunction with the satellite data. The GeoCBI index was preferred in this work since it is more consistently related to spectral reflectance than CBI for different ranges of burn severity (De Santis and Chuvieco, 2009).
Burn severity mapping techniques that use different calibration procedures between spectral indices (NDVI, dNDVI, NBR, dNBR, RdNBR, RBR) and field data (CBI, GeoCBI), have become more common in recent years (Miller and Quayle, 2015). Nonetheless, this is a rather difficult task since it is not always straightforward to relate field data to fire effects on satellite images (Miller and Quayle, 2015; Miller and Thode, 2007; Parks et al., 2014 and De Santis and Chuvieco, 2009). In particular, remotely sensed data cannot always explain field-based burn severity through regression models, since the latter also depend on factors such as ecosystem type, vegetation structure or spatial distribution, among others. For this reason, the identification of complex fire patterns and its understanding from satellite data is a key issue in burn severity mapping research programs; and in this regard, data-driven artificial intelligence and machine-learning techniques are being increasingly used to classify multispectral remotely sensed data for practical applications in wildfire monitoring (Meng et al., 2017 and Kern et al., 2017).
In this study, we propose and evaluate a new burn severity mapping algorithm from Landsat spectral indices and field-based data, for the assessment of two forest fires of different characteristics (size and level of damage) in Spain. Specifically, we present an enhanced methodological approach based on a Supervised Classification by Maximum Likelihood Estimation (MLE), strengthening this method with a proposal for the reduction of the adjust error (compared against traditional regression models) and the evaluation of how this affects the classification accuracy. Within this framework, the objectives of this research were: a) to determine the remote sensing indices with the best performance for burn severity mapping in two different forest fires in Mediterranean ecosystems, and b) to evaluate the accuracy of spectral index-based supervised classification and regression models for burn severity mapping. These objectives are conceived as developing methods for burn severity mapping by land management agencies.
Materials and Methods
Study Area
The two study areas were located in central Spain. The first one was located in the south-western part of the Province of Madrid (Pantano de San Juan area), while the second one was located in the north-eastern part of the Province of Guadalajara (Riba de Saelices area) (Figure 1). The Madrid fire burned a total area of 850 ha. The area was covered by pine forests (Pinus pinaster), and mixed forests of pine and an evergreen oak (Quercus ilex). The topography is abrupt and the altitudes range between 450 m and 1330 m. This region has a moderate-dry climate with annual precipitation of approximately 435 mm, and the average annual temperature is 13 °C (Ariza, 2017). The Guadalajara fire burned a total area of 13,000 ha. The fire started on July 16, 2005 and lasted 4 days. The area was covered by pine forests (Pinus pinaster), and mixed forests of pine with semi-deciduous oaks (Quercus pyrenaica and Quercus faginea). A large part of the burned area belonged to a protected area called the Alto Tajo Nature Park. The topography is rugged and the altitudes range between 1000 m and 1400 m. Rainfall in this region, which is evenly distributed through the year, averages 650 mm per year, and the average annual temperature is 10.2 °C (Viedma et al., 2015). Table 2 summarizes the main characteristics of the two wildfires.

Digital Elevation Models and Administrative Maps used as background, the fire perimeters were obtained from Landsat data processing as explained in Section 2.8.
Figure 1 Study area, fire perimeter, and field plots location: a) Madrid fire, and b) Guadalajara fire.
Table 2 Summary of the two wildfires analyzed in this study
Fire name | Location | Burning dates | Burned area (ha) | Dominance of species | Elevation (m) |
Madrid | Pantano de San Juan | 26-27/June/2003 | 850 | Pino pinea, Quercus ilex | 450-1330 |
Guadalajara | Riba de Saelices | 16-19/July/2005 | 13000 | Pino pinaster, Quercus pyrenaica, Quercus faginea | 1000-1400 |
Field data
Field-based assessment of burn severity was done according to the standard protocol of the Geometrically structured Composite Burn Index (GeoCBI), as proposed by De Santis and Chuvieco (2009). This index takes into account the fraction of coverage (FCOV) of each stratum used to compute the original CBI (Key and Benson, 2006), as well as the changes in the leaf area index (LAI) (De Santis and Chuvieco, 2009). GeoCBI is defined as (Equation 5):
Where m refers to each vegetation stratum and n is the number of strata. For the calculation of the GeoCBI each stratum is weighted by its FCOV value except for substrate, and the percentage of changes in the LAI is estimated for three strata (tall shrubs/saplings, understory trees, and canopy trees). Finally, the amount of new sprouts is added to the original estimation since it can significantly modify background reflectance; as a result, GeoCBI assigns a burn severity level (unburned, low, moderate, high) to each plot according to its final score (a number between 0.0 and 3.0), as stated in the protocol designed by De Santis and Chuvieco (2009).
Field plot locations were selected via stratified random sampling. Burn severity (derived from previous field trips), forest cover (derived from ancillary cartography and ortho-photographs), and accessibility determined the plot locations. GeoCBI field measurements were sampled in a maximal interval of twenty-five days after ignition date. And according to the requirement of fitting of Landsat spatial resolution (Key and Benson, 2006; Veraverbeke et al., 2010; Henry, 2008 and Arnett et al., 2015), the plots size was of 30 m by 30 m, and were located in fairly homogenous severity patches of 90 m by 90 m. Homogeneity assessment, which was performed on field by visual analysis of the surroundings, requires certain degree of personal training. Individual coordinates of the plot centers were captured with a hand-held Global Positioning System device (Garmin GPS 12); getting a planimetric accuracy of 5 to 15 meters. Additionally, we took digital photos from the center of each plot to the four cardinal directions in order to record vegetation structure, soil condition and additional information.
Field data consisted of 61 plots for the Madrid fire, and 129 plots for the Guadalajara fire. In the first case, 11 out of 61 were collected in unburned areas outside the limits of the fire perimeter. In the second case, 26 out of 129 plots belonged to the unburned category (figure 1 shows plot locations). Afterwards, we divided field data into two groups: 80% of the plots for training purposes, and the remaining 20% for validation. Selection for training and validation was random within each of the four burn severity levels (unburned, low, moderate, high).
Remotely sensed data
Four Landsat images were selected according to their availability and cloud coverage. For the Madrid fire, we selected a Landsat 7 Enhanced Thematic Mapper Plus (ETM+) image (path 201, row 32), corresponding to June 10, 2003 (pre-fire), and a Landsat 5 Thematic Mapper (TM) image corresponding to July 6, 2003 (post-fire). For the Guadalajara fire, we selected a Landsat 7 ETM+ image (path 200, row 32), corresponding to June 10, 2005 (pre-fire), and a Landsat 5 TM image corresponding to August 5, 2005 (post-fire). Table 3 shows a summary.
Table 3 Summary of remotely sensed data used in this study.
Fire name | Burning dates | Landsat Path/Row | Pre-fire date and sensor | Post-fire date and sensor |
Madrid | 26-27/June/2003 | 201/32 | 10/June/2003 Landsat 7 ETM+ | 06/July/2003 Landsat 5 TM |
Guadalajara | 16-19/July/2005 | 200/32 | 10/June/2005 Landsat 7 ETM+ | 05/August/2005 Landsat 5 TM |
Imagery was downloaded either from the GloVis server (</http://earthexplorer.usgs.gov/>) of the United States Geological Survey (USGS), or from the Earth Science Data Interface (ESDI) server (</http://glcfapp.glcf.umd.edu:8080/esdi/>) of the University of Maryland. We selected the Level L1T product (Standard Terrain Correction), which is radiometrically calibrated and ortho-rectified. Afterwards, we further process Landsat data for atmospheric and topographic correction, as described latter in this paper.
Workflow
The methodology goes as follows (Figure 2): imagery pre-processing (Section 2.5), spectral index calculation (Section 2.6), spectral separability analysis (Section 2.7), burn severity classification (Section 2.8), and accuracy assessment (Section 2.9).
After pre-processing ETM+ and TM pre- and post-fire images, six spectral indices were calculated. Then, we compared the capability of the different spectral indices to discriminate between different burn severity levels using field data. The best index was used to perform two classification methods: regression models and MLE. We trained both classification methods using 80% of the field plots; and the remaining 20%, to perform an accuracy assessment by means of a matrix error and the Kappa coefficient. As stated before, selection for training and validation was random within each of the four field-calculated burn severity levels (unburned, low, moderate, and high).
Imagery pre-procesing
Pre-processing of remotely sensed data included geometric accuracy check, radiance into reflectance conversion, subsetting of the scene, and topographic and atmospheric correction. Landsat images were Level L1T, which had been radiometrically corrected and ortho-rectified (WGS84 - UTM coordinate system, the study area is located in Zone 30 North) using the Shuttle Radar Topographic Mission (SRTM) 30 m pixel size Digital Elevation Model (DEM).
Temporal analysis between pre- and post-fire images requires good spatial and spectral consistency between datasets. We evaluated the spatial coherence between scenes using the Root Mean Square Error (RMSE) of the metadata, which involved the use of 213 pairs of coordinates (ground control points). Atmospheric and topographic correction aims at providing good spectral coherence between scenes. Atmospheric correction is a critical step in image processing, especially when the objectives of the study are based on the analysis of spectral indices, the use of different sensors, or in multi-temporal analysis (Chuvieco, 2009; Said et al., 2015 and Hantson and Chuvieco, 2011). This correction consists of the conversion of measured radiances above the atmosphere (Top Of Atmosphere, TOA) to surface reflectances (Bottom Of Atmosphere, BOA).
In this study, we used the ATCOR atmospheric correction model included in the GEOMATICA PCI-2015 software (Richter, 2007). This model calculates reflectance values at ground level based on the input image metadata, eliminating atmospheric effects in satellite images depending on different atmospheric conditions, aerosol types and water vapor. In addition, the spectral response of more or less illuminated areas is homogenized depending on the relief (topographic correction) and the effect of bidirectional reflectivity (BRDF) (Richter, 2007). Surface reflectance was calculated assuming complete solar illumination, that is to say, considering both the direct (Edir) and the diffuse (Edif) components (Flood et al., 2013) (Equation 6):
Where, i represents the spectral band, DN is the digital number of a pixel, LP is the atmospheric radiance, τ is the atmospheric transmittance (from sensor to Earth), d is the relative Earth - Sun distance in astronomical units for the day of image acquisition, and c 0 and c 1 are the sensor calibration coefficients (offset and gain).
Spectral indices calculation
Burn severity assessment clearly benefits from the analysis of spectral indices, particularly if Landsat data is used (Meng et al., 2017; Arnett et al., 2015; Holden et al., 2010 and Veraverbeke et al., 2011). Some of these spectral indices are vegetation indices; however, most of them were specifically design to detect burn severity, like the NBR index, that integrates the bands (NIR and SWIR) that respond most, but in opposite ways, to burning effects. Besides, many burn severity indices have been derived from the Normalized Burn Ratio. In this study, we calculated six spectral indices using the pre-processed Landsat bands. Some of them, like NDVI and NBR, were single-date. The others were multi-date, that is to say, they involved the use of pre- and post-fire imagery. Table 4 summarizes the spectral indices used in this study.
Table 4 Spectral indices used for burn severity assessment
Spectral index | Equation* | Reference |
Normalized Difference Vegetation Index |
|
Tucker (1979) |
Differenced NDVI |
|
Schepers et.al. (2014) and Escuin et al.(2008) |
Normalized Burn Ratio |
|
Key and Benson (2006) |
Differenced Normalized Burn Ratio |
|
Key and Benson (2006) |
Relative Differenced Normalized Burn Ratio |
|
Miller and Thode (2007) |
Relativized burn ratio |
|
Parks et al. (2014) |
*NBR is particularly designed for Landsat TM bands 4 (NIR) and 7 (SWIR).
RdNBR and RBR have been used previously as adjusted indices for bare ground and pre-fire vegetation conditions, and they were proved to improve burn severity estimations (Miller and Quayle, 2015; Miller and Thode, 2007 and Parks et al., 2014). We expected them to improve our estimates, through the adjustment of the effect that has the increased bare ground within the burned areas as a result of the fire-caused canopy loss.
Spectral separability análisis
We calculated a spectral separability index (M), as formulated in Equation 7, in order to evaluate the spectral indices differentiating capability of field-based burn severity levels, as a proxy for assessing the capacity of the spectral indices for discriminating burned severity effects from Landsat imagery. In particular, we used M with two objectives: (i) to both estimate the capability to discriminate between burned and unburned pixels and, (ii) the capability to discriminate among burn severity levels. The spectral separability index, also called normalized distance separability index, has been frequently used to assess the degree of discrimination of both broadband and imaging spectroscopy sensors in fire ecology studies (Meng et al., 2017; Schepers et al., 2014; Harris et al., 2011; Pereira et al., 1999 and Pleniou and Koutsias, 2013). The spectral separability index (M) is calculated as:
Where,
Burn severity classificaction
Both, the spectral separability index, and the coefficient of determination between GeoCBI field data and each of the six spectral indices (NDVI, dNDVI, NBR, dNBR, RdNBR, RBR), leaded us to the selection of the most appropriate spectral index. The latter would be the one on which the two classification methods (regression analysis and MLE) would be applied.
The first classification method was a regression model. These models evaluate the performance of the satellite-derived spectral indices as continuous metrics of burn severity, and test their correspondence to GeoCBI using regression equations (Miller and Quayle, 2015). In previous studies, simple linear and various non-linear regression models have been used to predict the relationship between CBI or GeoCBI (field data), and dNBR or RdNBR (satellite data) (Quintano et al., 2017; Miller and Thode, 2007; Meng et al., 2017 and Cansler and McKenzie, 2012). To facilitate the comparison between our results and other studies (Miller and Quayle, 2015; Cansler and McKenzie, 2012 and Stambaugh et al., 2015), we chose to use a linear regression model (Equation 8):
Where, Y is the satellite-derived metric being evaluated (spectral index), a is the gain, Xs is the GeoCBI index, and b is the offset value of the model. The goodness of fit of the regression model was evaluated through the coefficient of determination (R2) (Parks et al., 2014). We estimated two linear regression models, one for each fire (Madrid and Guadalajara), using in both cases 80% of the plots for training and 20% for validation. Next, we evaluated each remotely sensed burn severity classification metrics relative to GeoCBI. Four distinct categories are commonly used when mapping burn severity: unburned (0.0 ≤ GeoCBI < 0.1), low (0.1 ≤ GeoCBI ≤ 1.25), moderate (1.25 < GeoCBI ≤ 2.25), and high (2.25 < GeoCBI ≤ 3.0) (Key and Benson, 2006 and De Santis and Chuvieco, 2009). We calculated the values of each burn severity metric (spectral index) that corresponded to every GeoCBI threshold value (0.0, 0.1, 1.25, 2.25 and 3.0) to define burn severity metric thresholds for every class, using the linear regression model described in Equation (8).
The second classification method was a Maximum Likelihood Estimation (MLE). Other studies have already used supervised classifiers for mapping severity (Henry, 2008 and Turner et al., 1994). Mitri and Gitas (2006) were able to increase up to 83% overall accuracy of burn severity mapping using object-based supervised classification. In this case, we hypothesized they could had improved the classification accuracy through field data (GeoCBI). Among the conventional methods of classifying multispectral imagery, the MLE is the most widely used algorithm for pixel-based classification. MLE has shown to give the best results for classification of remotely sensed natural resource data among the parametric classifying algorithms (Yang et al., 2006). As is well known, the MLE allocates a pixel to the class with which it has the highest probability of correspondence (Ahmad and Quegan, 2013), where the likelihood L i (x) that a pixel x is a member of class (i) is given by (Equation 9):
Where, V i is the covariance matrix of class I, n is the number of spectral bands, and y is the Mahalanobis distance. The rescaling of L i (x) between 0 and 1 yields the MLE a posteriori probability Pi(x) (Dean and Smith, 2003). Field training sites were chosen based upon previous knowledge of the severity class each plot belonged to. Although it is possible to generate more classes during the classification process, the four severity classes were kept for training purposes due to the spectral disparities between them (e.g. moderate and high). Besides, it was also important to distinguish between burned from unburned areas, so field plots outside of the fire perimeter were included in the training procedure.
Fire perimeters were constructed based on our own burn severity maps, and assessed using official data from the Fire Management Office in Madrid (CAM, 2005), as well as the results of De Santis and Chuvieco (De Santis and Chuvieco 2009) for the Guadalajara fire. In particular, fire perimeters were created based on the burn severity maps developed from pre-fire NBR and dNBR (Kolden and Weisberg, 2007 and Finco et al., 2012).
Accuracy assesment
We assessed the regression models by using the coefficient of determination, and discretized-into-burn-severity-classes regression results were evaluated using confusion matrices; furthermore, we also evaluated the MLE using the latter technique. Confusion matrices produce several metrics: overall accuracy, producers and users accuracy, and the Kappa coefficient. Producer’s accuracy (omission error) is an evaluation of when a field plot is not assigned to the correct category. User’s accuracy (commission error) is an evaluation of when a field plot is assigned to the wrong category. The Kappa coefficient is a measure of the difference between the actual agreement between reference data and classified data, and the chance agreement between the reference data and randomly classified data. Confusion matrices allowed the comparison between classification methods (regression models and MLE), and between fire sites (Madrid and Guadalajara). We calculated overall accuracy as the percentage of field plots rightly classified into each burn severity class relative to its GeoCBI value, using the validation group of field plots (20% in each case). The validation assessment was conducted for each fire (Madrid and Guadalajara) individually. Our evaluation of the best classification method involves: (i) the overall classification accuracy of the field data, and (ii) the classification accuracy of each burn severity level. In this process, we used the GeoCBI thresholds that are most common, based on ecological conditions determined by the CBI and GeoCBI scale, in order to allow a more consistent interpretation of classes across multiple fires. These GeoCBI thresholds also facilitate comparison with previous studies (Miller and Thode, 2007 and Cansler and McKenzie, 2012).
Results
GeoCBI field data
Results from fieldwork revealed (in both cases Madrid and Guadalajara) that most of the plots belonged to the high severity class. In the case of Madrid, 56% of the field plots belonged to the high severity class, 42% to the moderate, and just 2% to the low severity class. The average GeoCBI for the Madrid fire was 2.67, thus classifying this fire into the high severity class. In the case of Guadalajara, 90.3% of the field plots belonged to the high severity class, 7.7% to the moderate, and just 2% to the low severity class. The average GeoCBI for the Guadalajara fire was 2.85, thus this fire was also assigned to the high severity class. Figure 3 shows the distribution of the GeoCBI field results.
Relationship between GeoCBI and the spectral indices
GeoCBI field data and the six spectral indices showed a relatively high degree of correlation, especially in the Madrid fire. Figure 4 shows some examples of GeoCBI field data and the corresponding RBR spectral values and locations. Table 5 shows the coefficient of determination (R2), the averaged by spectral index coefficient of determination, the adjusted R2 and the Root Mean Squared Error (RMSE). Although differences between spectral indices were not very large, RdNBR and RBR performed slightly better than the others did. The best R2-adjusted values were for the RBR and RdNBR indices, with values of 0.827 and 0.822, respectively. These results indicate better performance of RBR and RdNBR, higher correspondence between GeoCBI and these two spectral indices, and better capacity for explaining the variance in burn severity when compared to the rest of spectral indices.
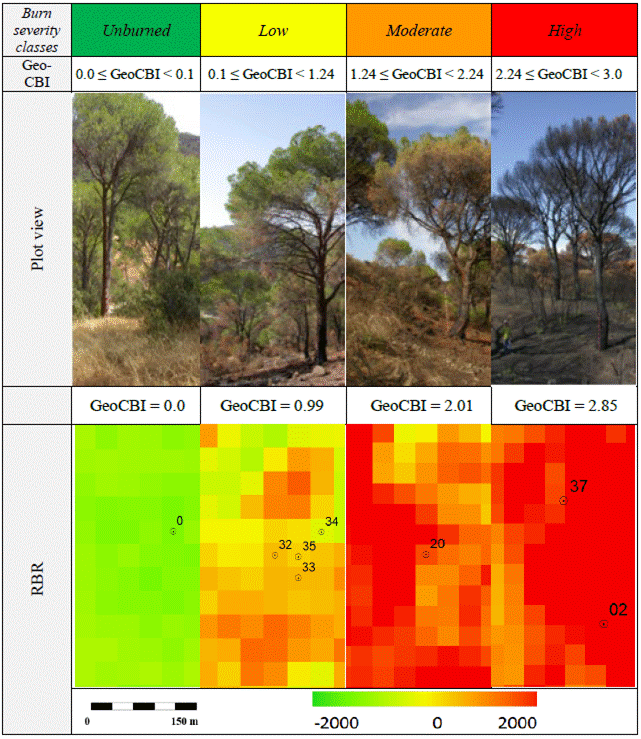
Figure 4 GeoCBI field plots (Madrid fire): burn severity classes, GeoCBI intervals, plot views, GeoCBI values for those plots shown on the pictures, and RBR index.
Table 5 Variance explained and overall correlation between GeoCBI and the six spectral indices
Spectral Index | Fire name | R 2 | R 2 average | R 2 adjusted | RMSE |
NDVI | Guadalajara | 0.583 | 0.699 | 0.580 | 0.730 |
Madrid | 0.814 | 0.811 | 0.423 | ||
NBR | Guadalajara | 0.596 | 0.697 | 0.593 | 0.719 |
Madrid | 0.798 | 0.794 | 0.441 | ||
dNDVI | Guadalajara | 0.615 | 0.694 | 0.612 | 0.702 |
Madrid | 0.772 | 0.768 | 0.469 | ||
dNBR | Guadalajara | 0.609 | 0.711 | 0.606 | 0.708 |
Madrid | 0.812 | 0.809 | 0.408 | ||
RdNBR | Guadalajara | 0.627 | 0.725 | 0.624 | 0.691 |
Madrid | 0.822 | 0.820 | 0.413 | ||
RBR | Guadalajara | 0.626 | 0.727 | 0.623 | 0.692 |
Madrid | 0.827 | 0.824 | 0.408 |
Figure 5 shows the correspondence between field data and satellite-derived information. These plots also show the distribution of GeoCBI field scores. For the Madrid fire GeoCBI severity values were more evenly distributed than for the Guadalajara fire. In the latter, most of the field-measured severity scores were of the high severity classes since it was a catastrophic fire. However, satellite response seemed to be not as fine as we would had expected, and showed a certain degree of signal saturation. According to Van Wagtendonk et al. (2004), dNBR saturates when CBI scores are larger than 2.3, as it could had happen in our case. The way field and satellite data relate to each other in the Guadalajara fire made that this fire was more difficult to be modelled than the Madrid fire.
Spectral separability
Figure 6 shows the spectral signatures that corresponded to four field locations in the Madrid (a) and Guadalajara (b) fire, one for each burn severity class (unburned, low, moderate and high). Spectral data came from Landsat TM images (post-fire data). Spectral values were extracted from the pixel where the field plots were located. The spectral signatures of burned areas were compared between the two fires, in order to obtain a better understanding of their spectral behavior and potential discriminatory ability. Bands in the NIR (B4) and SWIR (B7) are highlighted in the graph since they showed the highest changes in spectral response, what is the reason why most of the burn severity spectral indices uses them. The discrimination capability of the NIR band is much higher than that of the visible channels, despite the low spectral distance between bare land and vegetation in the NIR, and the low spectral distance between bare land and burned areas in the SWIR. Among these, the NIR band (B4) showed the highest discrimination between severity levels, and between burned and unburned areas, followed by band 7 (SWIR), band 5 (SWIR) and band 3 (red). (Table 6)

Figura 6 Spectral signature of the four burn severity classes according to Landsat TM channels: (a) Madrid fire and (b) Guadalajara fire. Spectral signature refers to four particular field plots, one per severity class.
Table 6 Spectral separability index (M) for each spectral index and fire site
Spectral index | Fire name | Separability index (M) | M average |
NDVI | Guadalajara | 0.707 | 1.338 |
Madrid | 1.968 | ||
NBR | Guadalajara | 0.922 | 1.386 |
Madrid | 1.850 | ||
dNDVI | Guadalajara | 0.871 | 1.614 |
Madrid | 2.356 | ||
dNBR | Guadalajara | 1.040 | 1.815 |
Madrid | 2.590 | ||
RdNBR | Guadalajara | 1.082 | 1.800 |
Madrid | 2.518 | ||
RBR | Guadalajara | 1.068 | 2.000 |
Madrid | 2.931 |
Spectral index selection
Spectral index selection was based on the results from the two previous sections, that is to say, the relationship between field and satellite data, and the spectral separability analysis. In general, we found a high correlation for all the spectral indices in both study areas. In the case of the Madrid fire, the RBR showed the highest correlation with GeoCBI (R2= 0.827) and the largest separability score (M= 2.93). In the case of Guadalajara, the best fit of R2 was presented for the RdNBR and the RBR indices (R2= 0.627 and 0.626, respectively), being also the two indices that showed the greatest spectral separability (M= 1.082 and 1.068, respectively). According to these results, RBR was selected for subsequent burn severity mapping as the best index for discrimination of severity.
Burn severity classifications
The RBR index, together with 80% of the field data, was used to carry out both classification burn severity methods proposed in this research: linear regression and MLE. Figure 7 shows the resulting maps along with the post-fire Landsat images used in this study. Although the detailed accuracy assessment is presented in the next section, results reached so far showed a relatively strong correlation based on the regression parameters of the RBR: in the Madrid fire, with a significant Pearson correlation of 0.897 and a RMSE of 0.440, and in the Guadalajara fire, with a Pearson correlation of 0.759 and a RMSE of 0.734. We also found that linear regression models tended to overestimate the unburned class, as well as to increment the number of pixels in the low and moderate burn severity classes versus the high severity ones.
The spatial heterogeneity within the burned area is clearly visible in both fires. All the burn severity maps (Figure 7) showed different levels of burn severity within the two study areas, and some small unburned or low-severity patches bordered by large moderate to high-severity areas. The spatial heterogeneity was higher in the Guadalajara fire. This fact might be due to a couple of reasons. Firstly, it was a high intensity and high fire and burn severity fire as one can conclude from the fieldwork (GeoCBI scores) and previous research (De Santis and Chuvieco, 2007, 2009). Secondly, the fire lasted several days and alternated intensive periods of burning (during the hot and dry daytimes) with less intensive burning phases (during nighttime).
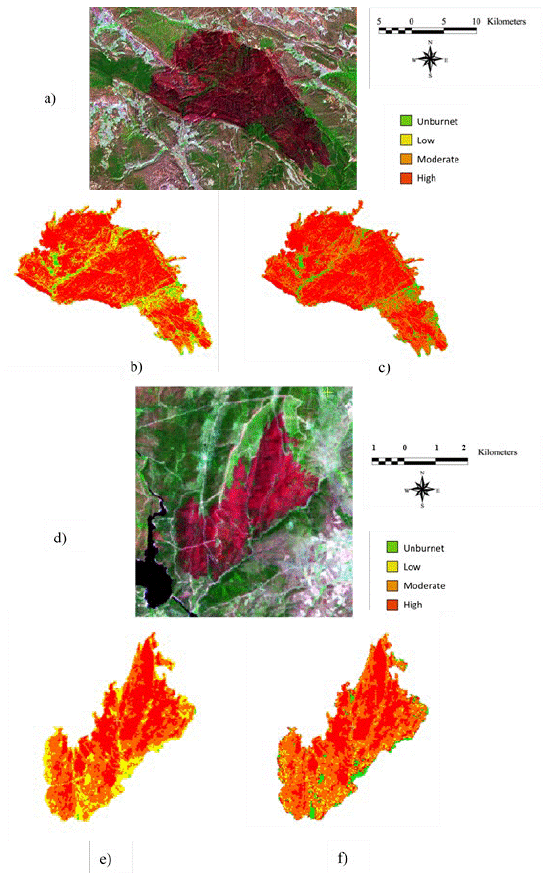
Figure 7 Burn severity mapping: a) Landsat TM (RGB 741), Guadalajara fire; b) Burn severity map by linear regression, Guadalajara fire; c) Burn severity map by MLE, Guadalajara fire; d) Landsat TM (RGB 741), Madrid fire; e) Burn severity map by linear regression, Madrid fire; f) Burn severity map by MLE, Madrid fire.
Accuracy Assesment
Overall classification accuracies for individual fires ranged from 58% (MLE, Guadalajara fire) to 92% (MLE, Madrid fire) (Table 7). When averaged among fires, the MLE classification method retrieved higher overall accuracy (75%) than the regression model (72.5%). Considering the Kappa coefficient, MLE classification method performed better than the regression models. Besides, when analyzing classification accuracies per class, we found that the accuracy of the classification in the lower and middle classes improved with the use of the MLE, in relation to the regression model technique. Which means that classification accuracy in the moderate and high severity classes decreased when the linear regression model was used, as opposed to the MLE model (Table 7). Classification accuracies among severity classes were significantly different, but indicated a general agreement between burn severity classifications and validation field plots. The increased classification accuracy in the low and moderate burn severity classes reflects differences between classification models, an effect due to the probabilistic classification of the MLE model in pixels where there is confusion between thresholds (Figure 8).
Table 7 Overall classification accuracy for classification methods using individual burn severity classes on RBR
Classification method | Fire name | Overall accuracy | Kappa | Kappa average | Classification accuracy (%) | ||||
Unburned | Low | Moderate | High | ||||||
Regression model | Guadalajara | 60 | 0.38 | 0.58 | User’s | 100 | 14 | 33 | 81 |
Producer’s | 100 | 50 | 50 | 53 | |||||
Madrid | 85 | 0.77 | User’s | 00.0 | 33 | 100 | 100 | ||
Producer’s | 00.0 | 100 | 100 | 100 | |||||
MLE | Guadalajara | 58 | 0.41 | 0.65 | User’s | 67 | 00.0 | 20 | 100 |
Producer’s | 100 | 00.0 | 100 | 41 | |||||
Madrid | 92 | 0.88 | User’s | 100 | 100 | 83 | 100 | ||
Producer’s | 100 | 100 | 100 | 80 |
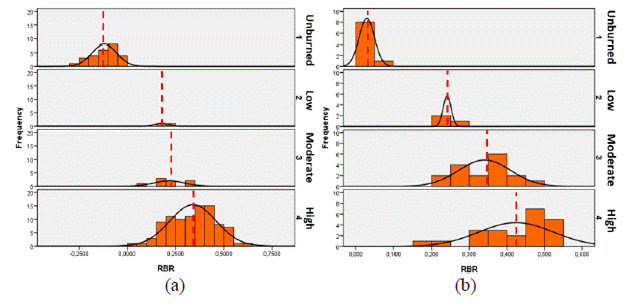
Figure 8 Frequency distributions of burn severity levels extracted pixels of RBR (regression models): (a) Guadalajara fire, and (b) Madrid fire. The vertical dash lines show a mean RBR value per class.
In order to understand the performance of the models in more detail, we extracted frequency distributions of RBR-based severity classes derived from the regression models for the Guadalajara and the Madrid fire, as shown in Figure 8. The histograms show that burned and unburned pixels were well separated and relatively easy to be discriminated, especially in the Madrid fire. However, the frequency distribution of the burn severity classes (low, moderate, high) was superposed between levels, e.g. mean RBR value of certain class (red dash line in Figure 8) was within the range of an upper class, and vice versa.
Discussion
Burn severity mapping is critical for the understanding of post-fire landscape changes and ecosystem resilience (San-Miguel et al., 2013; Ariza, 2017; Arnett et al., 2015 and Turner et al., 1994), a situation that has led to an increase of studies that use moderate to high spatial resolution satellite sensors (Key and Benson, 2006; De Santis and Chuvieco, 2009 and Veraverbeke, 2010). The increased availability of better spatial resolution imagery (e.g. Landsat or Sentinel series) provides an important opportunity not only for mapping burn severity, but also for studying post-fire regeneration and successional effects (Montealegre et al., 2014; Chuvieco, 2009; Key and Benson, 2006; De Santis and Chuvieco, 2009 and Finco et al., 2012). In this study, we explore the correspondence between satellite data and field-based measures of burn severity with the objective of choosing the index that performs best. Additionally, we use field and satellite data to investigate the differences in the use of supervised classification though MLE versus regression models for burn severity mapping.
Overall, burn severity mapping at Landsat TM and ETM+ scales was consistent with GeoCBI field measurements. The good performance of the weighted version of the CBI, the GeoCBI, may be due to the fact that it measures field parameters (FCOV, LAI, new sprouts) that are more closely related to the post-fire reflectance of the plot (De Santis and Chuvieco, 2009; Meng et al., 2017 and Cansler and McKenzie, 2012). Our results are comparable to those other studies with similar characteristics in different territories -Canada, USA, Spain- (Cocke et al., 2005; Holden et al., 2005; Miller and Quayle, 2015; De Santis and Chuvieco, 2009 and Cansler and McKanzie, 2012). Additionally, the correspondence between the newer indices (RBR, RdNBR) and GeoCBI field-based measures of burn severity indicates an improvement over more classical burn and vegetation indices (dNBR, NBR, dNDVI, NDVI), as it was found in previous research (Parks et al., 2014); none the less, we did find important differences between the two fires of the study.
The correspondence between satellite and field data was stronger in the Madrid fire. GeoCBI measures depend on several factors, such as the ecosystem type, the type of sampling, the number of samples and the severity intervals. Concerning the two first factors (ecosystem and sampling types) there were not great differences between the two wildfires. The number of samples per unit area was much larger in the Madrid fire, a simple fact that led to count on more significant field information; besides, the Guadalajara fire was much bigger and more severe. However, it seems that the main difficulty was due to the severity intervals. The weaker correspondence between satellite and field data in the Guadalajara fire was because 90% of the field plots belonged to the high severity class, according to the severity intervals previously defined. The high severity class in this study area was scattered, leading to lower R2 and separability values. This issue could have been solved using more adequate severity intervals, at the expense of making this research less comparable.
The findings of our investigation can be explained, at least partially, by the spectral properties of the severity ratings of the burn according to the severity classes. Previous studies of Cansler and McKenzie (2012) determined how robust were some spectral indices when applied to new regions. Additionally, our study identified the spectral index that performs the best in severity discrimination, the RBR, and the sensitivity of spectral reflectance values with respect to different levels of burn severity. After assessing the separability of six spectral indices for the discrimination of burning effects in Mediterranean ecosystems, we found that the spectral indices using NIR and SWIR bands (NBR, dNBR, RdNBR, RBR) performed better than those using the NIR bands (NDVI, dNDVI), as described previously (Miller and Quayle, 2015; Miller and Thode, 2007 and Meng et al., 2017). This is coherent with the higher capability of the SWIR (TM B7) in comparison with the red band (TM B3) for the discrimination between severity levels, and between burned and unburned areas (Figure 6).
In their study on the evaluation of spectral indices for burned area discrimination using MODIS/ASTER (MASTER) airborne simulated data, Veraverbeke et al. (2011) demonstrated that the highest sensitivity of the long SWIR region (1 900 - 2 500 nm) was between the 2 310 and 2 360 nm interval. In our case, we observed that the sensitivity in the VIS region (450-690 nm) was very poor, while the SWIR region (2 080-2 350 nm) showed moderate discriminatory power. However, the highest spectral separability between severity classes was observed in the spectral region of NIR (760-900 nm). This finding is consistent with the studies by Schepers et al. (2014) and Arnett et al. (2015), who used high resolution imagery for assessing burn effects in heathlands of Europe, and in mixed forests of western Canada, respectively.
In general terms, the spectral indices that were designed to account for the reflectance from the canopy affected by burn severity and the pre-fire conditions (RBR, RdNBR, dNBR), tended to show higher separability (Table 7), as preceding studies have shown (Miller and Quayle, 2015; Miller and Thode, 2007 and Meng et al., 2017). However, like in the Parks et al. (2014) study, the RBR was the index that best corresponded to field-based burn severity measurements, and had the highest classification accuracy compared to dNBR and RdNBR when discriminating different levels of severity. The RBR index, followed by RdNBR, offered better results than dNBR due to their adjustment to bare ground and pre-fire vegetation conditions (Miller and Quayle, 2015; Miller and Thode, 2007 and Parks et al., 2014). These differences, however, were especially noticeable in the Madrid fire.
Based on the error matrices parameters, the approach of the supervised classification by MLE, with an overall average accuracy of 76%, slightly improves the linear regression models approach, with an overall accuracy of 75%. The gain is low when we compared averaged values. However, it is noticeable in the Madrid fire (in the overall accuracy and the Kappa coefficient), and in the moderate and high classes of the Guadalajara fire. Therefore, our evaluation of the resulting burn severity maps indicate that the MLE approach can be used for forest burn severity mapping at the spatial scales of Landsat TM and ETM+ data with reasonable accuracy (Table 7), especially for forest fires similar to the Madrid one. The weaker results in Guadalajara could be explained by the severe characteristics of this wildfire and the massive concentration of scattered data in a single severity class. User and producer accuracies for low and moderate severity categories were poor for two reasons (Table 7): firstly, low and moderate classes had much less plots that the other categories, especially in the Guadalajara fire, it is important to consider the number of plots evaluated per category, as well as the accuracy of the registration of imagery (Miller and Quayle, 2015). Secondly, the location of the plots could had not been optimal in relation to the study area; additionally, another problem arises from the fact that plots do not align perfectly with the 30 m satellite pixel. The way fire behaves from surface to crown within 30 m according to Miller & Quayle (2015) and Safford et al (2012), is also a factor, since fire effects can vary considerably within a pixel, resulting in less precise evaluation of the burn severity in pixels adjacent to the limits of the fire perimeter, and the severity classes.
Finally, Figure 7 indicates that the heterogeneity of burn severity patterns was high in the two fires. Future research could determine the relationship between the degrees of severity and the changes induced by fire in the landscape, in order to provide information to post-fire management tasks and restoration programs, but also to provide information on changes in plant function associated with fire impacts (Smith et al., 2016 and Morgan et al., 2014).
Conclusions
GeoCBI field data and the Landsat-based spectral indices were used in this study to generate burn severity maps in Mediterranean ecosystems using two classification algorithms: linear regression models and supervised classification by maximum likelihood estimation (MLE). GeoCBI field data adjust moderately to the spectral indices, being very dependent on the ecosystem type, the type of sampling, the number of samples and the severity intervals. Among the analyzed six spectral indices, the Relativized Burn Ratio (RBR) and the Relative Differenced Normalized Burn Ratio (RdNBR) showed the best correspondence between satellite and field-based measures of burn severity. The RBR index was preferred in this study because it showed better spectral separability and consistency across regions. The supervised classification by MLE method, which used GeoCBI field data and the RBR index, improved the performance of the classical regression models for burn severity mapping. Differences between study areas might be due to the severity characteristics of the two wildfires. Since the Guadalajara fire was more severe than the Madrid fire, spectral indices tended to saturate more, a fact that affected the capacity of the spectral indices and the algorithms to model burn severity in a reliable manner.