Services on Demand
Journal
Article
Indicators
-
Cited by SciELO
-
Access statistics
Related links
-
Similars in SciELO
Share
Revista mexicana de ciencias agrícolas
Print version ISSN 2007-0934
Rev. Mex. Cienc. Agríc vol.5 n.spe10 Texcoco Nov./Dec. 2014
Articles
Impact of climate change on the geographic distribution of Gossypium hirsutum L. in Mexico
1Campo Experimental Centro Altos de Jalisco-INIFAP. Carretera Libre Tepatitlán-Lagos de Moreno km 8. Tepatitlán de Morelos, Jalisco, México.
2Centro Nacional de Recursos Genéticos- INIFAP. Carretera Libre Tepatitlán-Lagos de Moreno km 8. Tepatitlán de Morelos, Jalisco, México.
3Universidad de Guadalajara, Centro Universitario de Ciencias Biológicas yAgropecuarias. Carretera a Nogales km. 15.5, Predio Las Agujas S/N, Zapopan, Jalisco, México.
4Unidad Académicade Agricultura. Universidad Autónoma de Nayarit. Carretera Tepic-Compostela, km 9. Xalisco, Nayarit, México.
Climate change due to global warming is expected to have important effects on plant genetic resources, which has significant implications for agriculture, since it is assumed that these resources have genes that can provide features related hardiness "for most extreme weather conditions. The present study aimed to estimate the effect of climate change on the geographic distribution of Gossypium hirsutum in Mexico. The passport data of 387 accessions conducted between 1980 and 2011 by nine national and international institutions were used. Potential distribution of G. hirsutum was simulated, reference climatology for 1961-1990 and 2040-2069 climate change scenario using the model Maxent. Climate information was obtained from the portal WorldClim Earth System Grid where ASCII type images are downloaded with a spatial resolution of 2.5 minutes. For the 2040-2069 climate change scenario three general circulation models (MCG) were considered ECHAM5, MIROC (Medres) and UKMO_HADCM3 low emission scenario of greenhouse gases A2 in ASCII format with spatial resolution of 2.5 min. Both climatologies were worked to Idrisi selva software. The modelling results show that in the three MGG, the distribution G. hirsutum L. will be favoured by climate change, greatly increasing the areas in which it now appears.
Keywords: Gossypium hirsutum L.; climate change; geographical distribution; plant genetic resources
El cambio climático derivado del calentamiento global se prevé tendrá importantes efectos sobre los recursos fitogenéticos, lo cual tiene implicaciones significativas en la agricultura, ya que dichos recursos se asume poseen genes que pueden aportar características de rusticidad relacionadas con condiciones climáticas más extremosas. La presente investigación tuvo como objetivo estimar el efecto del cambio climático sobre la distribución geográfica de Gossypium hirsutum en México. Para ello se utilizaron los datos pasaporte de 387 accesiones realizadas entre 1980 y 2011 por nueve instituciones nacionales e internacionales. Se simuló la distribución potencial de G. hirsutum para la climatología de referencia 1961-1990 y un escenario de cambio climático 2040 -2069 utilizando el modelo Maxent. La información climática se obtuvo del portal WorldClim Earth System Grid de donde se descargaron imágenes tipo ASCII con una resolución espacial de 2.5 minutos. Para el escenario de cambio climático 2040-2069, se consideraron tres modelos de circulación general (MCG): ECHAM5, MIROC (Medres) y UKMO_HADCM3, bajo el escenario de emisiones de gases de efecto invernadero A2, en formato ASCII y con resolución espacial de 2.5 min. Ambas climatologías fueron trabajadas con el software Idrisi Selva. Los resultados de la modelación muestran que en los tres MGG se verá favorecida la distribución de G. hirsutum L. por el cambio climático, aumentando considerablemente las zonas en las que aparece actualmente.
Palabras clave: Gossypium hirsutum L.; cambio climático; distribución geográfica; recursos fitogenéticos
Introduction
Agriculture is one of the most vulnerable sectors to the impacts of climate change (FAO, 2007). The rainfed and irrigated agriculture yet are sensitive to changes in regional weather patterns, including interannual scale. Climate predictions of twenty-first century (IPCC, 2007) are not promising and rather indicate that climate change will be emphasized during the course of this century and established most extreme conditions for crops, both in terms oftemperature and humidity, so it will be necessary to generate measures of adaptation to new climatic conditions. One of the most important steps that are displayed, is the generation ofnew varieties, which are expected to be adapted to warmer and probably less humid environment (IPCC, 2007; Zarazúa, 2011).
Against this background, it is likely that the formation of new gene to feed the generation of genotypes with features such populations required. In this process, the use of wild relatives of crops will be critical, since it is known that associated genes found in these plant genetic resources for higher hardiness in relation to several factors related to crop production, such as drought resistance (Ruiz et al., 2008), resistance to high and low temperatures (López et al., 2005; Ruiz et al., 2013), and resistance to pests and diseases (De la Paz et al. , 2010), among other.
The presence and distribution of wild species is closely related to their agroclimatic requirements and how those requirements are satisfied in various environments (FAO, 2007) . The presence of climate change may threaten the satisfaction of these requirements and thus for the presence and distribution of wild species (Jarvis et al., 2008) .
Mexico is considered as one of the countries with the highest biodiversity (Mittermeier and Goettsch, 1992), among other factors due to its great climatic diversity (García, 2004). It is currently estimated that 12% of the total world's biodiversity is found in Mexico; this biodiversity, 12% of genera and 50-60% of the plant species are endemic (CONABIO, 2006).
With the presence of climate change, climate diversity of the country may be altered, so that may change the composition ofecosystems (Lasco et al., 2008; Schneider et al., 2009) and the geographical distribution of plant genetic resources. Gossypium hirsutum is considered one of these resources, as Mexico with Central America is considered the centre of origin of this species (Jenkins, 2003). The importance of this resource is that currently 90% of world cotton production is derived from G. hirsutum. Probably the native inhabitants of the Yucatán Peninsula were the first to domesticate G. hirsutum (Brubaker and Wendel, 1994). These early forms of semi-domesticated cotton dispersed to other Middle and into the Caribbean (Iqbal et al., 2001). From here, there was a selection to a reduced seed dormancy, annual growth habit and photoperiod independent flowering, creating very similar genotypes to current cultivars (OGTR, 2008).
The aim ofthis investigation was to determine the potential impact of climate change on the geographic distribution of niches G. hirsutum L. under baseline and climate change with three GCMs (MCG). ECHAM5, MIROC (Medres) and UKMO_HADCM3 under the scenario of greenhouse gasA2 greenhouse. For this, algorithms and models are used distribution niches.
One ofthese models is Maxent which is a method ofartificial intelligence that applies the principle ofmaximum entropy to calculate the most probable geographic distribution for a species. The result of the model expresses the most appropriate value ofthe potential habitat for the species as a function of environmental variables used. A high value of the distribution function at each pixel indicates that it has favourable conditions to find the species (Phillips et al., 2008; Morales, 2012). This model has recently been used to determine both the distribution of species of plants, animals and insects (Contreras et al, 2010; González et al, 2010; Ibarra et al, 2010; Sork et al., 2010; Torres and Khayat, 2010; Paredes et al., 2011; Sobek et al, 2012).
The comparison between the results ofthree different MCG give an idea of the trend of the distribution of the species under study.
Materials and methods
Databases
A database of 387 geo-referenced accessions was used which took place between 1980 and 2011 by nine institutions: National Research Institute for Forestry, Agriculture and Livestock (INIFAP), National Institute of Ecology (INECOL), Autonomous University of Mexico (UNAM), Yucatan Centre for Scientific Research (CICY), Department of Agriculture of the United States (USDA), Autonomous University of Yucatan (UAY), Chapingo Autonomous University (UACH), Graduate College of Science Agricultural (CP) and the Missouri Botanical Garden (MBG).
The climatology WorldClim for the climate period 1960 1990 was used to characterize the environmental conditions ofthe collection sites. To model the distribution niches of G. hirsutum the aforementioned weather and climate change scenario was used, as well as the climate change scenario 2040-2069, considering three MCGs (ECHAM5, MIROC (Medres) and UKMO_HADCM3) by considering a scenario of greenhouse gas, A2. All of these with a spatial resolution of 2.5 minutes. Both GIS were compiled in the Idrisi selva software (Eastman, 2013). In addition to these variables, other variables of diagnostic soil type and topography from the environmental information system (SIAN) of the National Research Institute of Livestock Agricultural and Forestry were also included (Díaz et al, 2012).
Study variables
From the geographical coordinates of the collection sites, a vector file was generated and, with appropriate reference to the climate of raster file used was made of the GIS Analysis system module IDRISI Selva (Eastman, 2013) to extract accession timely information related to the following variables: average annual accumulated precipitation (PAA), mean annual temperature (TMA), average annual minimum temperature (TIA), maximum average temperature (TXA), maximum mean temperature of the warmest month (TXMMC), mean minimum temperature of the coldest month (TIMMF) and altitude (A).
Slope, annual temperature variation, temperature variation, precipitation, average minimum temperature, average temperature and average maximum temperature from May to October and November-April periods: to model the distribution of niche, plus the following variables were used above.
Modelling the geographic distribution
In order to determine the potential geographic distribution of G. hirsutum we used the model Maxent (Phillips et al, 2006) version 3.3.3 k, which has been tested and proven as one of the best modellers niche distribution (Elith et al, 2006). This software is based on the statistical principle of maximum entropy, which calculates the probability distribution of maximum entropy (closest to the uniform distribution), from presence data (collection) and absence, adjusting species distribution to all pixels in the study area and on the principle the idea that the best explanation for an unknown phenomenon maximize uncertainty probability distribution depending on those pixels where the species has been detected (Phillips et al., 2004, 2006). The end result is an expression ofthe type:
Where= C1, C2 ... Cn, are constant; F1, F2 ... Fn are climate variables and Z is a constant which ensures that the sum of all values of P for all cells is equal to 1.
The result can be given by three types of outputs: raw, cumulative and logistics, forthis case the output oflogistic type is used, which provides results in a map of potential distribution where the maximum ambient fitness is between 0 to 1.
Ecological descriptors
Organic descriptors were determined for G. hirsutum from all collection points used for analysis and climatic range in which they settle. This was done using the "IDRISI Selva" system with raster images of each environmental variable and the geographical coordinates ofeach point ofcollection. After obtaining these data, outliers were sought to establish environmental ranges in which the species is established.
Results and discussion
The Table 1 shows some basic statistics of environmental variables sites of the accession that describe G. hirsutum. The environmental ranges of this species are large. This vs la points to the hardiness of this species, a feature that could be beneficial in environments where climate change will manifest more aggressively (FAO, 2007).
The maximum value, minimum value and average value of each variable expressed in Table 1 also represent descriptors ecological G. hirsutum. According to the accessions tested, the range of annual precipitation varies between 198 and 1945 mm, contrasting values (especially the minimum value) with those reported by Aragon (1995) who sets a range from 750 to 1 500 mm annually.Also, the analysis result altitudinal limits ofthe accessions studied, reported that the distribution range of G. hirsutum goes from 0 to 2 344 m, values that differ from those reported by Baradas (1994), who notes that the altitudinal range is from 0 to 600 m.
Also, the species is adapted to a wide climatic range, as might find them in places where the temperature ranges from -0.1 up to 41.2 °C.
In the Figure 1, are shown the accessions used for the modelling of G. hirsutum using the software Maxent where we can clearly see that, the species is distributed in the warm sub-humid region ofthe country, presenting some accessions in the arid region.
This algorithm performs some statistical tests based on 25% of data allocated in the random test. One of the statistics is performed regarding sensitivity vs. specificity of data for model generation, receiver operating characteristic (ROC); i.e., the ratio oftrue positives against the rate offalse positives, corresponding to the probability of selecting at random a pair ofvalues, one positive (pixel with presence of the species) and negative (pixel with no species), the model awarded to the positive value higher value (Hanley, 1982). This test shows that the AUC (area under the ROC curve) for the training data is 0.937 (data used for model generation), this means that the model fits almost perfectly.
It is noteworthy that, the species is adapted to arid and semi-arid regions with warm climates and semi-warm (Aragón 1995; Ruiz et al., 1999), although in the map can be seen that the accessions are mostly in the warm humid region of the country.
In the Figure 2, the resulting map between environmental analysis classified by ranks reference to the weather is displayed. The classification was made in 5 slots fitness: very high, high, medium, low and very low, corresponding to 0.20 fitness ranges from logistics performance thrown by Maxent (0-1), with areas of very low environmental aptitude whatever is between 0 to 0.20 and the high ability of those found in ranges of 0.80 to 1.
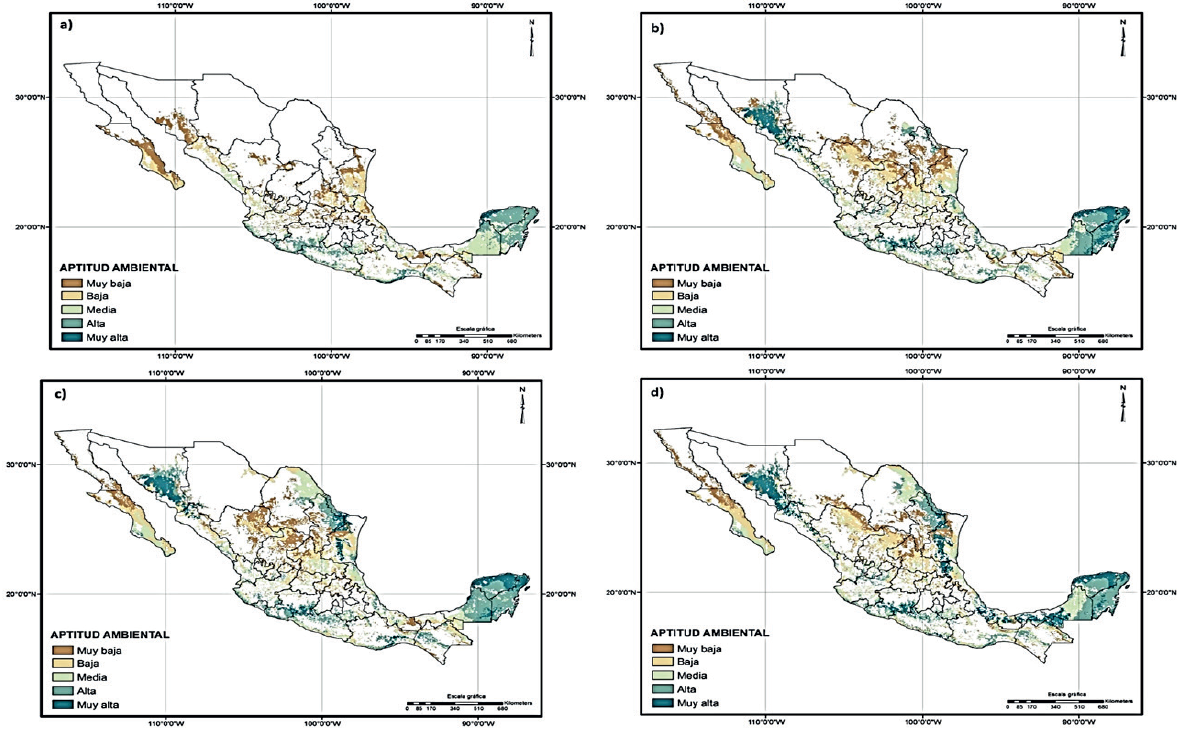
Figure 2 Environmental fitness of G. hirsutum: a) climatology reference 1960-1990 period; b) 2040-2069 climatology ECHAM5 GCM; c) 2040-2069 climatology MCG UKMO_HADCM3; d) 2040-2069 climatology MCG MIROC (Medres).
Moreover, the mapping b, c and d of Figure 2, showing the potential geographic distribution of G. hirsutum under the 2040-2069 climate change scenario for the different MCG used in this study, which have very contrasting results with the current situation and very similar to each other (Table 2).
Table 2 Percentage of territorial occupation of G. hirsutumL., baseline for territorial occupation and potential for climate change 2040-2069 scenario with three MCGs .
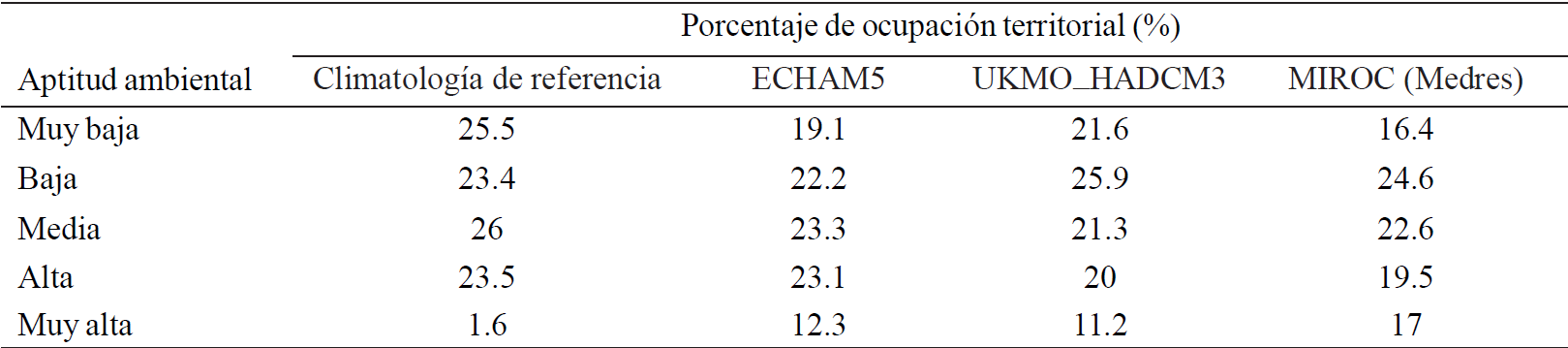
It is noteworthy that, the percentages listed in the Table 2 were subtracted in surfaces of water bodies, urban sprawl and all those types of vegetation where G. hirsutum cannot be set as coniferous forests and riparian vegetation, to mention a few. This layer ofinformation comes from the digital chart using soil and vegetation (INEGI, 2005).
In general it can be seen that compared to the baseline, all models have the same trend, i.e., decreasing of the lowest category and increase in the categories oflow, medium, high and very high environmental suitability.
The model that showed a higher decrease in the surface ofthe very low category was UKMO_HADCM3 with a decrease of 21.6%, with model MIROC which showed less decline from baseline climatology in this category (16.4%). As for the other categories MIROC has more gain surface in the high category and ECHAM5 features higher gain in the middle and high categories.
Models that have lower profit percentage area occupied by category are ECHAM5 in the low category, UKMO_ HADCM3 in middle and high class and MIROC (Medres) in the high category.
In general it can be observed that all models show a trend in which the species expanded their distribution to climate change conditions, extending the States of Sonora, Sinaloa, Tamaulipas and Nuevo León.
It is quite evident that, the impact caused by climate change will benefit the distribution of G. hirsutum L., unlike many other species whose geographical distribution is affected by changes in weather patterns (Jarvis, 2008; Villers, 2009; Contreras et al., 2010; Sork et al, 2010). Within the benefit climate change will bring, is the expansion ofareas with high and very high environmental suitability, which will cause the appearance ofnew areas with this level ofenvironmental fitness, particularly in the region consists of the southern and Sonora and northern Sinaloa, the region formed by the boundary area of Tamaulipas and Nuevo León and the Southern Gulf State of Tabasco (Figure 2).
Studies in the southwestern United States show that, the fields for cotton cultivation (Gossipyum sp.) will increase up to 26-36% of its current condition, if the levels of carbon dioxide continue to increase (Doherty, 2003).
The plant processes that are directly affected by changes in the atmosphere (CO2 concentration) are mainly photosynthesis, photorespiration, night respiration and transpiration (Fitter, 1987). The temperature increase under future climate change and elevated CO2 levels that will be beneficial to the productivity of cotton to a certain threshold, where the rate of photosynthesis is less than optimal or environmental stress is high (Reddy et al., 2000).
Conclusions
For future climate scenarios, it is expected thatthe distribution ofthe species will be favoured, because climate change will increase the areas of highest environmental suitability for G. hirsutum. On this conclusion there is agreement on the three MCGs used, being the model MIROC (Medres) which reported higher environmental surface high fitness.
Therefore, it is important to identify those areas that will be enhanced or be affected and begin to implement the necessary measures to ensure the conservation ofthe species as genetic resources strategies.
As for the cotton production from G. hirsutum it is very likely that no additional time is required for the varieties already available, but it is a medium term that will have to develop strategies focused on developing varieties that efficiently go though photosynthesis at high temperatures and under low moisture availability on the soil, in order to adapt to any climate scenario.
Literatura citada
Brubaker, C. L.and. Wendel, J. F. 1994. Reevaluating the origin of domesticated cotton (Gossypium hirsutum, Malvacea) using nuclear restriction fragment length polymorphisms (RFLPs) Am. J. Bot. 81:1039-1326. [ Links ]
Contreras, R.; Luna, I. y Ríos, C. 2010. Distribución de Taxus globosa (Taxaceae) en México: Modelos ecológicos de nicho, efectos del cambio de uso de suelo y conservación. Revista Chilena de Historia Natural 83:421-433. [ Links ]
De la Paz G. S.; Sánchez, G. J. J.; Ruiz, C. J.A.; Ron, P. J.; Miranda, M. R.; De la Cruz L.; Lépiz I. R. 2010. Diversidad de especies insectiles en maíz y teocintle en México. Folia Entomol. Mex. 48(2):1-6. [ Links ]
Doherty, R.; Mearns, L.; Raja, K.; Downton, M.; McDaniel, L. 2003. Spatial scale effects of climate scenarios on simulated cotton production in the southeastern U.S.A. Climatic Change 60:99-129. [ Links ]
Elith, J.; Graham, C.; Anderson, R.; Dudík, M.; Ferrier, S.; Guisan, A.; Hijmans, R.; Huettmann, F.; Leathwick, J.; Lehmann, A.; Li, J.; Lohmann, L.; Loiselle, B.; Manion, G.; Moritz, C.; Nakamura, M.; Nakazawa, Y.; Overton, J.; McPeterson, A.; Phillips, S.; Richardson, K.; Scachetti-Pereira, R.; Schapire, R.; Soberón, J.; Williams, S.; Wisz, M. and Zimmermann, N. 2006. Novel methods to improve prediction of species distributions from occurrence data. Ecography 29: 129-151. [ Links ]
Organization of the United Nations Food and Agriculture Organization (FAO). 2007. Adaptation to climate change in agriculture, forestry and fisheries: Perspective, framework and priorities. FAO Interdepartmental working group on climate change. FAO Electronic Publication. Rome, Italy. 24 p. [ Links ]
Fitter, A. and Hay, R. 1987. Environmental physiology of plants. 2nd Edition. Academic Press, London. [ Links ]
García, E. 1989. Apuntes de climatología. Offset Larios. México, D. F. 155 p. [ Links ]
García, F. 2006. La conservación de los recursos fitogenéticos de México. Revista Ciencia, Universidad Autónoma de Nuevo León, 10(2):115. [ Links ]
González, C.; Wang, O.; Strutz, S.; González, C.; Sánchez, V. and Sarkar, S. 2010. Climate Change and risk of Leishmaniasis in North America: predictions from ecological niche models of vector and reservoir species. PLoS Negl Trop Dis. 4(1):585. [ Links ]
Hanley, J. and McNeil, B. 1982. The meaning and use of the area under a receiver operating characteristic (ROC) Curve. Radiology. 143(1): 29-36. [ Links ]
Holdridge, L. R. 1967. Life zone ecology. Tropical science center. San José, Costa Rica. [ Links ]
Ibarra, M. J. L.; Rangel, P. G.; González, F. F. A.; De Anda, J.; Zamudio, R. M. E.; Martínez, M. E. y Macías, C. H. 2010. Modelo de nicho ecológico para predecir la distribución potencial de fitoplancton en la Presa Hidroeléctrica Aguamilpa, Nayarit, México. AmbiAgua. 5(3):60-75. [ Links ]
Instituto Nacional de Estadística, Geografía e Informática (INEGI). 2005. Conjunto de la carta de uso de suelo y vegetación. Continuo Nacional. Serie II. Escala 1: 1 000 000. Instituto Nacional de Estadística y Geografía. México, D. F. [ Links ]
Intergovernmental Panel on Climate Change (IPCC). 2007. Climate Change 2007: mitigation of climate change. Contribution of working group III to the Fourth Assessment Report of the Intergovernmental Panel on Climate Change. Metz, B.; Davidson, O.; Bosch, P.; Dave, R. and Meyer, L. (Eds.). Cambridge University Press, UK. and USA. 851 p. [ Links ]
Iqbal, M. J.; Reddy, O. U. K.; El Zik, K. M. and Pepper, A. E. 2001. A genetic bottleneck in the 'evolution under domestication' of upland cotton Gossypium hirsutum L. examined using DNA fingerprinting. Theoretical and applied genetics. 103: 547-554. [ Links ]
Jarvis, A.; Lane, A. and Hijmans, R. 2008. The effect of climate change on crop wild relatives. Agric. Ecosys. Environ. 3158:11. [ Links ]
Jenkins, J. N. 2003. Cotton. In: traditional crop breeding practices: an historical review to serve as a baseline for assessing the role of modern biotechnology. OECD. 61.70pp. [ Links ]
Lasco, R. D.; Pulhin, F. B.; Sánchez, P. A. J.; Villamor, G. B. and Villegas, K.A. L. 2008. Climate change and forest ecosystems in Philippines: Vulnerability, adaptability and mitigation. J. Environ. Sci. Managem. 11(1):1-14. [ Links ]
López, S. J. L.; Ruiz, C. J.A.; Sánchez, G. J. J. y Lépiz, I. R. 2005.Adaptación climática de 25 especies de frijol silvestre (Phaseolus spp.) en la República Mexicana. Rev. Fitotec. Mex. 28(3): 221-230. [ Links ]
McArthur, R. H. 1972. Geographical ecology: patterns in the distribution of species. Harper and Row, New York. 54 p. [ Links ]
Mittermeier, R. y Goettsch, C. 1992. La importancia de la diversidad biológica de México. México ante los retos de la biodiversidad. Conabio, México. 57-62pp. [ Links ]
Morales, S. N. 2012. Modelos de distribución de especies: Software Maxent y sus aplicaciones en conservación. Revista Conservación Ambiental. 2(1):1-5. [ Links ]
Morrone, J. 2005. Hacia una síntesis biogeografía de México. Rev. Mex. Biod. Diciembre. 76:2. [ Links ]
Office ofthe Gene Technology Regulator (OGTR). 2008. The biology of Gossypium hirsutum L. and Gossypium barbadense L. (Cotton), versión 2. Department of Health and Ageing, Government of Australia. Australia. 87 p. [ Links ]
Paredes, G. D. M.; Ramírez, B.A. y Martínez, M. M. A. 2011. Distribución y representatividad de las especies del género Crotalus en las áreas naturales protegidas de México. Rev. Mex. Biod. 82:689-700. [ Links ]
Phillips, S. and Dudik, M. 2008. Modeling of species distribution with MaxEnt: new extensions and a comprehensive evaluation. Ecography. 31:161-175. [ Links ]
Reddy, K.; Hodges, H. and Kimball, B. 2000. Crop ecosystem responses to global climate change: cotton. In: Reddy, K. R. and Hodges, H. F. (Eds.). Climate Change and Global Crop Productivity, Chapter 8, CAB International, UK. 161-187 pp. [ Links ]
Ruiz, C. J. A.; Durán, P. N.; Sánchez, G. J. J.; Ron, P. J.; González, E. D. R.; Holland, J. B. and Medina, G. G. 2008. Climatic adaptation and ecological descriptors of 42 Mexican maize (Zea mays L.) races. Crop Sci. 48:1502-1512. [ Links ]
Ruiz Corral, J. A.; Sánchez, G. J.; de J. Hernández, C. J. M.; Willcox, M. C.; Ramírez, O. G.; Ramírez, D. J. L. y González, E. D. R. 2013. Identificación de razas de maíz adaptadas a condiciones deficientes de humedad mediante datos biogeográficos. Rev. Mex. Cienc. Agric. 4(6):829-842. [ Links ]
Ruíz, J. A. 1999. Requerimientos agroecológicos de cultivos. Centro de Investigación Regional del Pacifico Centro, INIFAP, SAGAR. 24-26pp. [ Links ]
Ruíz, J. A.; Medina, G.; Ramírez, J. L.; Flores, H. E.; Ramírez, G.; Manríquez, J. D.; Zarazúa, P.; González, D. R.; Díaz, G. and de la Mora C. 2011. Climate change and its implications in five producing areas of maize in Mexico. Rev. Mex. Cienc. Agric. 2:309-323. [ Links ]
Schneider, R. R.; Hamann, A.; Farr, D.; Wang, X. and Boutin, S. 2009. Potential effects of climate change on ecosystem distribution in Alberta. Can J. For. Res, 39: 1001-1010. [ Links ]
Sobek, S. S.; Kluza, D.A.; Cuddington, K. and Lyons, D. B. 2012. Potential distribution of emerald ash borer: What can we learns from ecological niche models using Maxent and GARP? Forest Ecol. Managem. 281(1):23-31. [ Links ]
Sork, V.; Davis, F.; Westfall, R.; Flints, A.; Ikegami, M.: Wang, H. and Grivet, D. D. 2010. Gene movements and genetic association with regional gradients in California valley oak (Quercus lobata Née) in the face of climate change. Mol. Ecol. 19:3806-3823. [ Links ]
Torres, R. y Jayat, J. 2010. Modelos predictivos de distribución para cuatro especies de mamíferos (Cingulata, Artiodactyla y Rodentia) típicas del Chaco en Argentina. Mastozoología Neotropical. 17(2):335-352. [ Links ]
Villers, L.; Arizpe, N.; Orellana, R.; Conde C. y Hernández, J. 2009. Impactos del cambio climático en la floración y desarrollo del fruto del café en Veracruz, México. INCI. 34(5):322-329. [ Links ]
Walter, H. 1985. Vegetation of the earth and ecological systems of geobiosphere, third (Ed.). Springer, Heidelberg. [ Links ]
Zarazúa, V. P. 2011. Evaluación del cambio climático y sus impactos sobre los cultivos de trigo, maíz y agave de la región Ciénega de Chapala análisis retrospectivo y prospectivo. Tesis doctoral. Universidad de Guadalajara. Zapopan, Jalisco, México. [ Links ]
Received: February 2014; Accepted: June 2014