1 Introduction
Time of flight image (TOF) is a noninvasive and painless technique widely used in Magnetic Resonance Imaging (MRI) for the diagnosis of aneurysm. A medical image analysis can be subjective and susceptible to errors as it is based on a visual examination followed by a manual delineation of blood vessels. Computational techniques obtain more efficient image segmentation, consequently these techniques are used for segmentation of blood vessels.
Most bleeding are frequently the result of rupture on small vessels, breaking on arteries walls or veins associated with different pathologies. Particularly, diseases as aneurysms and arteriovenous malformations [5].
Medical studies commonly used for detection of these cerebrovascular problems usually are focused on medical imaging, such as MRI and CT, to name two examples of the various methods of medical imaging. Thus, research areas dedicated to medical imaging have great importance.
One of the techniques implemented in these diagnosis areas, mainly in MRI, is the TOF (Time of Flight) [6]. The human body is composed of geometric structures of different types, i.e. linear structural such as: ducts, veins, nerves, arteries and others. Additionally, is medical techniques are constantly being designed to be less invasive without compromising the image quality so that sufficient information may be provided on vascular structures [4].
The aim of this work is to enhance vascular structures using a Vesselness filter, upon completion of a decision based on fuzzy thresholding, error in the selection of vascular structures is minimized.
1.1 Theoretical Basis
One of the techniques implemented in this area of diagnosis is the TOF, which consists in emitting radio frequency pulses to excite protons in tissues and the vascular system. There are two types of proton reactions to these radio frequencies. The first corresponding to protons at rest that are saturated by continuously receiving radio frequencies, thus the response signal intensity is low. The second type of reaction corresponds to protons in movement and are not saturated resulting in a high intensity signal. This allows blood to be distinguished from tissue [6].
According to the theory of fuzzy logic control every element belonging to a set "U" has a degree of membership and can be measured with a real number ranging from 0 to 1. The benefits of using this technique are the control combination of elements and the use of prior knowledge of these elements which in our case will be the selection of pixels.
Membership functions in classical logic has set in which elements may be present or not, on the contrary, fuzzy logic allows to describe the degree of belonging or inclusion of an object.
In medical imaging, segmentation is the process of splitting an image into anatomical sections that are of interest, this being a difficult task. Images often have more noise and usually contain a structure with different shapes and sizes, which varies depending on the medical imaging technique. Segmentation can rely on an operator by whole, partly or be completely automated. These two types of segmentation algorithms depend governing its operation [3].
2 Materials and Methods
The purpose of using a Hessian matrix is to use variations of gray values in the neighborhood of a point of interest r 0 that can be modeled as a Taylor expansion of second order [2]
where ∇0 is the gradient and H0 the Hessian matrix. The second derivative of a gray value of the function “f” in either direction is given by:
with
Analyzing the Hessian matrix makes it possible to detect linear structures. In this case, a dark structure on a bright background is characterized by a second derivative positively through a line and a small second derivative of any of the signals along the line. A Hessian matrix with positive and negative eigenvalues, large and small, reflects this. In general, these eigenvalues can be used to simulate structures.
Fuzzy logic can deal with information that is inaccurate, as the average height, low temperature, or high strength. Taking the example of the temperature control system based on fuzzy logic, which can interpret if the temperature is high or low. Using this method in image processing provides the ease to integrate knowledge from expert's ambiguous issues such as the selection of veins or arteries [1].
TOF intensities of images and the results of applying the TOF Vesselness filter, with the help of specialized neurologists, are analyzed and the probability of the voxels with high intensity values belonging to the vascular system is determined. A set of rules that allowed implement-filtering intensities of voxels and information yielding the Vesselness technique were created.
3 Results
Vesselness results of applying the filter to images TOF type, cutting Sagittal 45 and 80 are shown in the sets of images of figures 1 and 2.
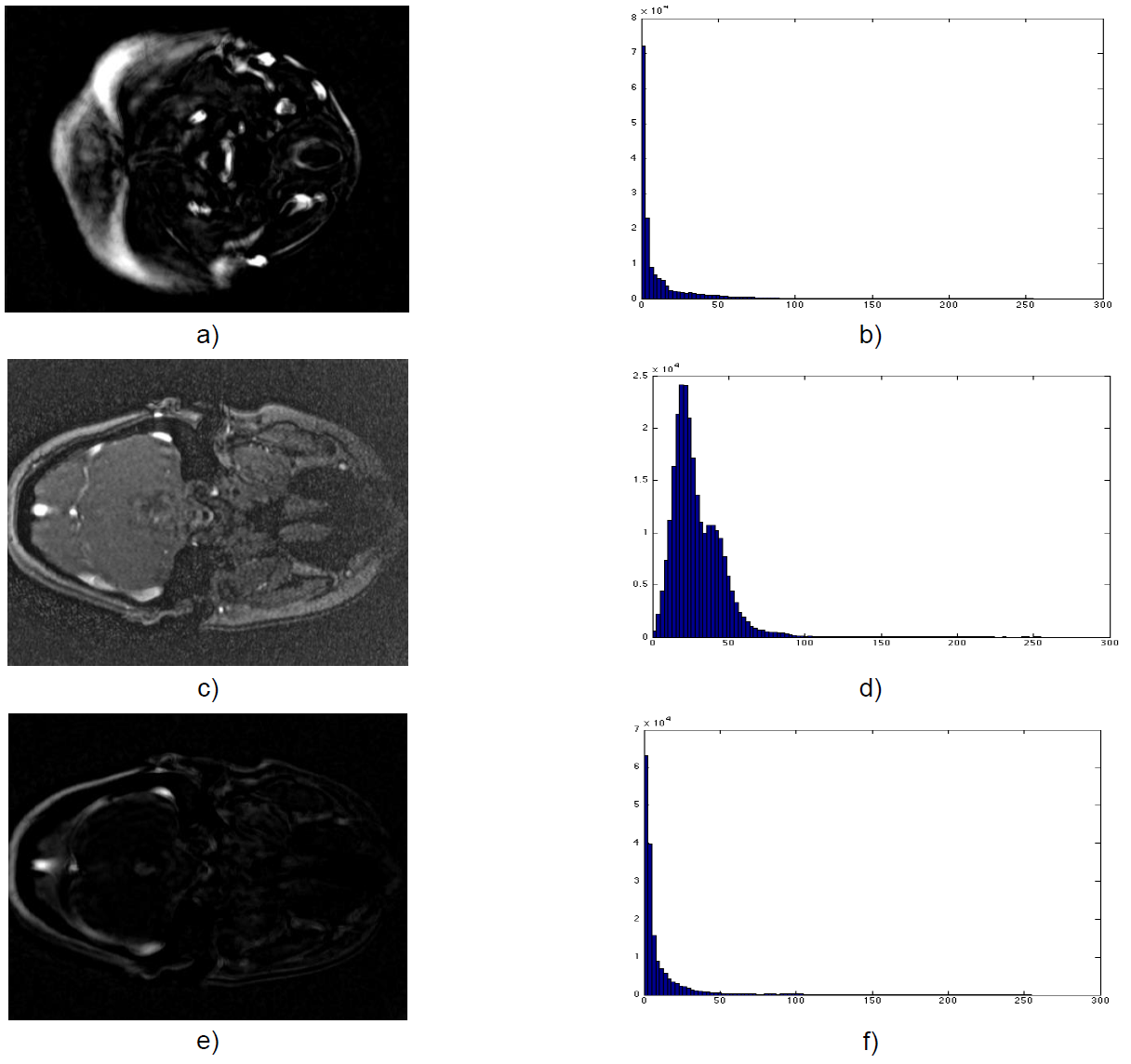
Fig. 2 Vesselness filter. a). Vesselness image, b) Histogram of a), c) Slice 80 of dataset, d). Histogram of c), e) Vesselness image, f) Histogram of e)
Images in figure 3 represent results of the thresholding by fuzzy logic. The histograms show a decrease of pixels with low intensities.

Fig. 3 Fuzzy classification, a). Image of fuzzy classification, b). Histogram of a), c). Image of fuzzy classification, d). Histogram of c)

Figure 4 Fuzzy thresholding, a). Image of fuzzy thresholding, b). Histogram of a), c). Image of fuzzy thresholding, d). Histogram of c)
The segmentation method for the vascular system eliminates low-intensity pixels that do not belong to the vascular system that could be taken as false positive when evaluating images.
Table 1 presents the values of sensitivity, specificity, accuracy. This allows measuring the performance objective and quantitative method. A visualization of the jugular veins segmented by the proposed method is shown in figure 5.
Table 1 Performance method
Image | Sensitivity | Specificity | Accuracy |
---|---|---|---|
40 | 0.671 | 0.9816 | 0.9792 |
41 | 0.726 | 0.9804 | 0.9784 |
42 | 0.671 | 0.9789 | 0.9763 |
43 | 0.604 | 0.9823 | 0.9797 |
44 | 0.590 | 0.9821 | 0.9797 |
45 | 0.602 | 0.9837 | 0.9809 |
46 | 0.671 | 0.9941 | 0.9912 |
47 | 0.724 | 0.9989 | 0.9971 |
48 | 0.725 | 0.9991 | 0.9974 |
49 | 0.756 | 0.9985 | 0.9971 |
4 Discussion
The proposed method has an advantage over other algorithms that do not consider intensities. As a method based on intensity threshold selection it is critical to the success of the technique, the threshold selection decisions based on diffuse for a better response in the segmentation.
Although some images were not segmented in its entirety, the chosen filter showed its effectiveness in treating such images concerning the results of sensibility and specificity
5 Conclusions
Although some images were not segmented in its entirety, the chosen filter showed its effectiveness in treating such images concerning the results of sensibility and specificity. As reported in the tests conducted with images, increasing or decreasing the number of filters and techniques could greatly affect the segmentation of the vascular system.
The final model based on fuzzy thresholding, showed an approximate segmentation, however changing the threshold selection rules using fuzzy logic could improve the response of the method.