Services on Demand
Journal
Article
Indicators
-
Cited by SciELO
-
Access statistics
Related links
-
Similars in SciELO
Share
Agrociencia
On-line version ISSN 2521-9766Print version ISSN 1405-3195
Agrociencia vol.50 n.2 Texcoco Feb./Mar. 2016
Crop science
Localization of garlic apex by digital image analysis techniques
1 Universidad de Guanajuato, División de Ciencias de la Vida. Ex Hacienda El Copal. 36500. Carr. Irapuato-Silao km. 9. A.P. 311. Irapuato, Guanajuato. México. (saldanar@ugto.mx).
Sowing and harvesting are the most costly operations in the garlic production (Allium sativum L.), because they are carried out by hand. In order for mechanized sowing to be feasible, the garlic clove must be placed in the soil with the apex facing upwards because placing it randomly reduces its yield in up to 23 %. The objective of this study was to develop an algorithm to identify the garlic apex through artificial vision. For this purpose, a video camera and illumination lamps were used, with which digital images of the cloves of garlic were obtained. The images were processed to identify the apex in four steps: 1) capturing the image; 2) detecting the perimeter edge of the garlic cloves; 3) calculating the angles on the inside edge of the cloves and localizing the apex under the hypothesis that it coincides with the smallest angle on the inside edge; 4) identifying the need of reorienting the apex. The impact of the location and size in the correct identification of the apex was evaluated statistically and the border detection methods Canny, Roberts and Sobel were compared. The results did not show significant statistical differences (p>0.10) between the different sizes and positions of the garlic when localizing the apex, which is convenient. However, there were significant statistical differences (p≤0.10) between the border detection methods, because Canny’s had a better performance in the localization of the apex. The algorithm developed in this research could be used to design a mechanical system to reorient the apex through artificial vision in garlic sowing.
Keywords: Allium sativum L.; border detection; Canny; mechanization of sowing; artificial vision
La siembra y la cosecha son las operaciones más costosas en la producción del ajo (Allium sativum L.), pues se realizan a mano. Para hacer factible la siembra mecanizada, el diente de ajo se debe colocar en el suelo con el ápice hacia arriba porque colocarlo al azar reduce el rendimiento hasta en 23 %. El objetivo de este estudio fue desarrollar un algoritmo para identificar el ápice del ajo mediante visión artificial. Para ello se empleó una cámara de video y lámparas de iluminación, con lo cual se obtuvieron imágenes digitales de dientes de ajo. Las imágenes se procesaron para identificar el ápice en cuatro pasos: 1) captura de la imagen; 2) detección del borde perimetral de los dientes de ajo; 3) cálculo de ángulos en el interior del borde de los dientes y localización del ápice bajo la hipótesis de que éste coincide con el ángulo más pequeño en el interior del borde; 4) identificación de la necesidad de reorientar el ápice. El impacto de la posición y tamaño en la correcta identificación del ápice se evaluó estadísticamente y se compararon los métodos de detección de bordes Canny, Roberts y Sobel. Los resultados no mostraron diferencias estadísticas significativas (p>0.10) entre los diferentes tamaños y las posiciones del ajo al localizar el ápice, lo cual resulta conveniente. Pero sí hubo diferencias estadísticas significativas (p≤0.10) entre los métodos de detección de bordes utilizados, porque el de Canny tuvo mejor desempeño en la localización del ápice. El algoritmo desarrollado en esta investigación se podría usar para diseñar un sistema mecánico que reoriente el ápice a través de visión artificial en la siembra de ajo.
Palabras clave: Allium sativum L.; detección de bordes; Canny; mecanización de la siembra; visión artificial
Introduction
Mexico occupied one of the first ten places in garlic exports (Allium sativum L.) in the world, between 2002 and 2011, representing income of over 13 million dollars annually (Reveles et al., 2009). The primary garlic producing states in Mexico are Zacatecas, Guanajuato and Baja California with a surface sown of over 5000 ha (SIAP, 2015). There are studies about the climate adaptation of garlic in strategic geographical regions in Mexico (Pérez et al., 2010; Reveles H. et al., 2011). Garlic sowing has been performed manually by submerging the clove in the soil with the apex facing upwards at a depth of 4-6 cm; this task requires experience, since good germination and plant development depend on it (Calderón et al., 2003). The seed placed with the apex randomly will have a negative impact on the yield (Chengqian et al., 2008). Mechanical or pneumatic sowers reduce the sowing time in 3 to 1 (Calderón et al., 2003), but they reduce up to 23 % of the yield (Castellanos et al., 2004), compared to manual garlic sowing. Mechanized sowing seeks to place cloves at a regular distance, reduce the mechanical damage to the cloves and place the clove in the soil with the apex facing upwards (Bakhtiari and Loghavi, 2009). Chi and Hui (2013) presented a device to orient the garlic based on the analysis of digital image, for which they used an algorithm that made the image of the garlic binary (zeros for the background and ones for the garlic) and analyzed the inferior part of the image by reviewing two horizontal lines of pixels; if one of the lines presented few white pixels, they considered that it was the apex, and otherwise it was the root. Tavakoli and Najafzadeh (2015) identified shoots in potatoes by analyzing the edge of the potatoes through an algorithm based on the detector of Prewitt edges. In some prototypes of pneumatic sowers for garlic, such as the one developed by Cabrera and Serwatowski (1996), an artificial vision system could be installed that allows orienting the garlic apex correctly during sowing through an electromechanical mechanism. Because of this, the objective of this study was to develop and evaluate an algorithm for the artificial vision system of the prototype to allow localizing and deciding whether the garlic apex should be reoriented or not. The hypothesis was that the algorithm proposed could identify the orientation of the apex of the garlic cloves through the analysis of images of the cloves, captured with a camera, under conditions of controlled illumination, by using an Edge Detector Method and without the influence of the size and the localization of the garlic clove.
Materials and Methods
The evaluation of the algorithm consisted in analyzing the influence of the size and the localization of the garlic cloves on the correct localization of the apex. The algorithm is based on the information of the garlic edge, so that three methods for edge detection (MED) were evaluated: Canny, Robert and Sobel.
The algorithm developed to detect the apex in this study took up ideas from other studies (Chi and Hui, 2013; Tavakoli and Najafzadeh, 2015), and is made up of four stages: 1) Capturing the image of the garlic clove; 2) detecting the edge of the garlic clove in the image; 3) locating the apex from the edge; and 4) identifying the need to reorient the apex. The algorithm was developed in MATLAB® 8a installed in a desktop computer (Intel®, Pentium® CPU 3.80 GHz and 2.00 GB RAM). The seed was selected by eliminating the cloves of garlic with mechanical damage, with disease, rotten or deformed, and they were classified into four sizes according to their thickness (Figure 1): small (0.4-0.8 cm), medium (0.8-1.3 cm), large (1.3- 1.8 cm) and jumbo (1.9-2.5 cm). The total of garlic cloves was 60, that is, 15 of each size.
The images of garlic cloves were captured with a CS120-Intel video camera configured for a resolution of 320x288 pixels and connected to the desktop computer. The camera was installed on a metallic bridge structure with the lens oriented downwards, 28 cm above the garlic, on the transporter band of the prototype already described (Figure 2).
A pair of Stocker Yale Lotus Lamps (Prophotonics, Salem, NH, USA) were placed 45 cm above the garlic, with an angle of 25 degrees with regard to the horizontal (Figure 2). The heights and angles mentioned allowed a uniform and adequate intensity in illumination to maximize the garlic-background contrast and minimize the garlic’s shadow. The background of the images used to contrast the garlic color was a flat band of neoprene rubber. The garlics were placed on the transporter band with the apex in a random position. Images of the 60 cloves of garlic were captured with the cloves in two positions, lateral and frontal, which because of the garlic’s geometry are the only ones possible. The images were processed using three MEDs and generating 360 images of edges of garlic cloves.
The algorithm developed in this study includes the detection of the edge of the garlic clove as a fundamental part. The information contained on the edge can be used to segment the image or to recognize objects and the geometrical shapes of their contours. Most of the MEDs use local operators based on different discrete approximations from the first and second derivatives of the levels of grey in the image (Alcañiz et al., 1999). The results generated by each MED are different (Bin and Samiei, 2012). Therefore, in this study three of the most common MEDs were tested: Canny (1986), Sobel (1990) and Roberts (1965). The thresholds for each MED were chosen according to the conditions of illumination of the image, since the lamps were placed on a garlic clove minimizing the shadows in the image and expecting a definite and closed edge of the garlic clove’s contour as a result of the MED (Figure 3B). The resulting thresholds of the test were: 0.6 for Roberts, 0.6 for Sobel, and in Canny the inferior threshold was 0.45 and the superior threshold 0.8.

Figure 3 A) Image of a clove of garlic; B) detection of the edge by Canny; C) calculation of the internal angle to identify the clove’s apex.
The apex was located according to the steps in Figure 4: 1) The three MEDs were applied to the images of garlic cloves (Figure 3A); 2) the procedure of cleaning the edge generated images of the edge (Figure 3B); 3) the pixels that made up the edges detected were defined in function of their location within the image, for which each pixel was considered as a P point of coordinates (x, y), where x is the number of the column, y is the number of the row of the pixel matrix that makes up the image of the edge, where the image has the pixel of the coordinates (0,0) in its superior left corner; and 4) the internal angles θ for each pixel of the edge were calculated from the P coordinates in three pixels (Figure 3C).
The concept is shown in Figure 3C, the vectors
where θ is the internal angle formed between vectors
The last step of the algorithm was to indicate the localization of P found for the apex, as well as a brief message about reorienting the apex or not. Figure 4 summarizes the algorithm to locate and decide on the reorientation of the garlic’s apex.
When concluding the algorithm, the results from localization of the apex and the reorientation message were reviewed visually. The response variable of Detection of the Apex (DA) acquired a value of 1 for the cases where the algorithm located the garlic’s apex and made the decision of rotating it correctly, and 0 otherwise. The factors implicated in the DA analysis were size (TA), position (PO) and the MED. Since the DA variable had a dichotomous response, a variable was transformed in an interval scale (DAT), which is a particular type of scale where amplitude is divided into equidistant fractions, to measure the relative differences with regard to the points of the total scale. To create it, three sets of data were established for each size with five garlic cloves in each, of which the proportion of correct detections of the apex were calculated, giving as a result three repetitions. The new DAT variable expressed in the percentage did not fulfill the assumptions of normalcy (Shapiro-Wilks test) and homogeneity of variance between the levels of each factor (Levene test), which is why the non-parametrical tests H of Kruskal-Wallis for TA and MED and U of Mann-Whitney for PO, were used, using StatGraphics Plus V5.1 STSC and Statistical Graphics Corporation (Bekersville, Maryland, USA).
Results and Discussion
In 83.3 % of the 360 images of garlic edges, the apex was identified correctly based on the minimum value of the internal angle. For the frontal position, the apex was detected correctly in 80 % of the cases, and for the lateral position it was 86.7 %. For the size factor, the percentages of correct responses were: 85 %, 80 %, 86.5 % and 81.5 % for small, medium, large and jumbo, respectively. With regard to the MEDs, the proportions of correct responses were 91.25 % for Canny, 81.25 % for Sobel and 77.5 % for Roberts. Figure 5 shows the graph of the averages of proportion of correct responses that contrasts TA against PO, where the higher percentage of correct responses for large garlic in the lateral position stands out (93 %), followed by small garlic in this same position. For the frontal position, the size of the garlic did not make a difference in the percentages of correct responses.
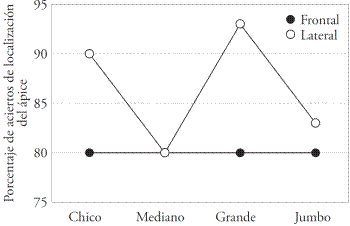
Figure 5 Averages of proportion of correct responses for localization of the garlic apex for the combination of factors Position and Size.
In the lateral view, small (Figure 6A) and large (Figure 6C) garlics exhibit a marked difference in the curvature of the edge for the base and apex, whereas some medium garlics show very similar acute angles for the base and apex (Figure 6B), and the jumbo garlics present a semi-circular edge in base and apex (Figure 6D). This explains the higher percentage of correct responses in the identification of the apex for small and large garlic.
Figure 7 is the comparative graph of the average of percentages of correct responses between MED and PO, and Canny had the highest percentage of correct responses for both positions, followed by Sobel and then Roberts. The lowest percentage of correct responses was obtained in the frontal position of the garlics for the three MEDs, which could be because the pointed shape of the apex is more exposed in the lateral view than in the frontal.

Figure 7 Averages of proportion of correct responses for localization of the garlic apex for the combination of factors Methods for Edge Detection and Position.
Canny had the highest percentages of correct responses (95 %) for the small, medium and large sizes, except for jumbo (Figure 8). The greatest difference between the percentages of correct responses for the three MEDs was observed in medium garlic, since Roberts obtained the least percentage of correct responses (65 %). Roberts presented higher sensitivity to noise than the other MEDs, and the lowest percentage of correct responses for the small, medium and large sizes, except in the jumbo size where it obtained a higher percentage of correct responses.
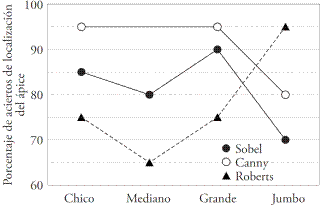
Figure 8 Averages of proportion of correct responses for the combination of factors Methods for Edge Detection and Garlic Size.
In Figures 9 and 10, the steps for detection of the jumbo garlic edge were observed in the lateral position using Roberts and Canny as MED, respectively. The process of cleaning the image to detect the edge of the garlic consisted in the dilation of the edge (Figures 9C and 10B), the filling of holes (Figures 9D and 10C), and the erosion and extraction of the final edge (Figures 9E and 10D). Canny obtained a definite edge and without noise (Figure 10A), while Roberts was more sensitive to noise (Figure 9B). Some jumbo garlics in both positions generated a shadow, which was detected by the three MEDs generating a line close to the edge of the garlic, and as a result of the rest of the process, the line was incorporated to the edge of the garlic. Canny and Sobel generated a line more distant from the edge than Roberts, which modified the curvature of the edge when integrating the line (Figure 10D). For Roberts, the line was integrated to the edge by increasing the size without changing its curvature (Figure 9E). An additional lamp could eliminate the shadows and improve the results obtained by Canny and Sobel. Palomares et al. (2005) presented a MED based on Canny and the paradigm of Logarithmic Image Processing (LIP), managing for non-homogeneous illumination in an image not to affect the identification of the edges, which is another alternative for this study.
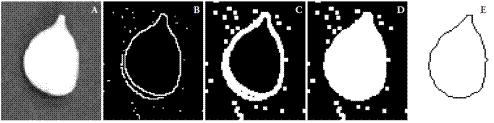
Figure 9 A) Image of a clove of garlic; B) detection of the edge by Roberts; C) dilation of the edge; D) filling of holes; E) final erosion and extraction of the edge.

Figure 10 A) Detection of the edge by Canny; B) dilation of the edge; C) filling of holes; D) final erosion and extraction of the edge.
Canny was the MED that allowed obtaining the highest number of correct responses (91.25 %), followed by Sobel and then Roberts. In a similar study, Chi and Hui (2013) report 94 %, 97 % and 92 % of correct responses in the identification of the apex for medium, large and jumbo garlic, respectively. This was quite close to what was achieved by Canny in our study for the medium and large garlic (95 %), except for jumbo garlic. In other studies various MEDs were compared for other applications (Bin and Samiei, 2012; Sachin et al., 2011; Shrivakshan and Chandrasekar, 2012; Akram and Ismail, 2013), with results that allow concluding a better performance than Canny.
In Table 1, the non-parametric tests applied to DAT for the factors in study are presented. The value of the Mann-Whitney statistical U test did not show significant differences (p>0.10) for PO; the same for TA, H of Kruskal-Wallis and the four medians were similar; and for MED, H of Kruskal- Wallis allowed concluding that at least one median is different (p≤0.10). In this last case the DMS test (p≤0.10) was performed on the averages of the ranges assigned by the Kruskal-Wallis test and two statistical groups were detected: the Canny method (group A) with a higher percentage of correct responses in the apex detection, followed by the Sobel and Roberts methods (group B).
Table 1 Statistics applied to DAT. H of Kruskal-Wallis for TA and MED, and U of Mann-Whitney for PO.

†Significant value (p≤0.10). ¶Not significant value (p>0.10).
In sum, the averages of percentages of correct responses for the identification of the apex did not show significant statistical difference over the detection of the apex, when compared for the garlic PO and TA. This is desirable because garlic will not require prior selection and could feed in a random position with small influence on the correct placement and reorientation of the apex.
Conclusions
The Canny technique obtained the highest percentage of correct responses in the apex detection, outperforming the Sobel and Roberts methods, without influence from the size and position of the garlic according to the Kruskal- Wallis and U of Mann-Whitney tests. However, Roberts obtained the highest percentage of correct responses for the jumbo size, since the shadow of the jumbo garlic was integrated to the edge without altering its curvature, which happened with Canny and Sobel. The lateral view of small and large garlics obtained the highest percentage of correct responses in the apex detection.
The high percentage of correct responses in the localization of the apex achieved with Canny suggests the implementation of the algorithm proposed in an automatic system for reorientation of the apex, which will require demonstrating its functioning under field work conditions. The algorithm presented sensitivity to uniformity in illumination, which was observed in the results obtained by Canny for jumbo garlic, so a detailed study of the illumination will be necessary.
Literatura Citada
Alcañiz R. M., V. Grau C., J. Lizandra, M. Aranda, J. Navarro, y E. Moltó G. 1999. Procesamiento Digital de Imagen. Servicio de Publicaciones Universidad Politécnica de Valencia. España. pp: 108-109. [ Links ]
Akram, A., and A. Ismail. 2013. Comparison of edge detectors. Int. J. Comp. Sci. Information Technol. Res. 1: 16-24. [ Links ]
Bakhtiari, M. R., and M. Loghavi. 2009. Development and evaluation of an innovative garlic clove precision planter. J. Agric. Sci. Technol. 11: 125-136. [ Links ]
Bin, L., and M. Samiei. 2012. Comparison for image edge detection algorithms. IOSR J. Comp. Eng. 2: 1-4. [ Links ]
Cabrera S. J. M., y R. Serwatowski. 1996. Diseño de una sembradora neumática para ajo. In: Memoria del II Congreso Latinoamericano de Ingeniería Agrícola. Bauru, SP. Brasil. (Edición en CD, clave MCA518). pp: 1-9. [ Links ]
Calderón R., E., R. Serwatowski H., J. M. Cabrera S., y C. Gracia L. 2003. Siembra mecanizada del ajo: métodos y equipos. Agrociencia 37: 483-493. [ Links ]
Canny, J. F. 1986. A computational approach to edge detection. IEEE Trans. Pattern Analysis Machine Intell. 8: 679-698. [ Links ]
Castellanos, J. Z., P. Vargas-Tapia, J. L. Ojodeagua, and G. Hoyos. 2004. Garlic productivity and profitability as affected by seed clove size. HortScience 39(6): 1272-1277. [ Links ]
Chengqian, J., Y. Wensheng, W. Chongyou, and Z. Min. 2008. Experimental study on effects of the bulbil direction on garlic growth. Trans. Chinese Soc. Agric. Eng. 4: 155-158. [ Links ]
Chi, G., and G. Hui. 2013. Direction identification system of garlic clove based on machine vision. ELKOMNIKA Indonesian J. Electr. Eng. 11(5): 2323-2329. [ Links ]
Palomares, J. M., J. González, y E. Ros. 2005. Detección de bordes en imágenes con sombras mediante LIP-Canny. In: Memoria del Simposio de Reconocimiento de Formas y Análisis de Imágenes del I Congreso Nacional de Informática. Granada, España. pp: 71-76. [ Links ]
Pérez M., L., J. Navarro L., B. Mendoza C., y R. Ramírez M. 2010. Evaluación del rendimiento de compuestos de ajo tipo Taiwán. Rev. Acta Univ. 20: 63-69. [ Links ]
Reveles H., M., R. Velásquez V., M. D. Alvarado N., y S. Rubio D. 2011. “CEZAC 06”: nueva variedad de ajo tipo Jaspeado para la región norte centro de México. Rev. Mex. Ciencias Agríc. 2(4): 601-606. [ Links ]
Reveles H., M., R. Velásquez V., y A. G. Bravo L. 2009. Tecnología para cultivar ajo en Zacatecas. Boletín técnico no. 11. Instituto de Investigaciones Forestales, Agrícolas y Pecuarias. Centro de Investigación regional Norte-Centro. Campo experimental Zacatecas. pp: 26-32. [ Links ]
Roberts, L. G., 1965. Machine Perception of 3D solids, In: Optical and Electro-Optical Information Processing. MIT Pres. pp: 159-197. [ Links ]
Sachin G., B, S. Jivan Y. and S. Aishwari P. 2011. Comparison of SUSAN and Sobel edge detection in MRI images for feature extraction. Int. J. Comp. Appl. 2: 23-27. [ Links ]
Shrivakshan G. T., and C. Chandrasekar. 2012. A comparison of various edge detection techniques used in image processing. Int. J. Comp. Sci. Issues 9: 269-276. [ Links ]
SIAP (Sistema de Información Agroalimentaria y Pesquera). 2014. Secretaria de Agricultura, Ganadería, Desarrollo Rural, Pesca y Alimentación. http://www.siap.gob.mx/ . (Consulta: Septiembre 2014). [ Links ]
Sobel, I. 1990. An Isotropic 3X3 Gradient Operator. Machine Vision for Three - Dimensional Scenes. Freeman, H. (ed). Academic Pres. New York. pp: 376-379. [ Links ]
Tavakoli, M., and M. Najafzadeh. 2015. Application of the image processing technique for separating sprouted potatoes in the sorting line. J. Appl. Environ. Biol. Science. 4: 223-227. [ Links ]
Received: March 2015; Accepted: September 2015