Services on Demand
Journal
Article
Indicators
Related links
Share
Problemas del desarrollo
Print version ISSN 0301-7036
Prob. Des vol.50 n.198 Ciudad de México Jul./Sep. 2019 Epub Nov 16, 2019
https://doi.org/10.22201/iiec.20078951e.2019.198.66383
Articles
Road infrastructure and economic growth in Mexico
a National Autonomous University of Mexico (UNAM), Mexico, E-mail: ing.isidroenrique@hotmail.com.
b National Polytechnic Institute of Mexico (IPN), Mexico, E-mail: gangeles@ipn.mx
c Independent Consultant. E-mail: dkdoctorat@hotmail.com
Results from studies of the relationship between road infrastructure and regional economic growth have so far been inconclusive. This paper analyzes the effect of the availability of road infrastructure on regional production in Mexican municipalities. At a regional level, the extent of road availability in a municipality was determined using an Geographic Information System (GIS) and ordinary least squares analysis, which identified the existence of a positive relation between road density and production in municipal regions. It was shown that the most significant impact of roads on gross regional production is in the manufacturing and retail sectors, while roads have no significant impact on agriculture, livestock production, fishing, and mining. Toll-free roads in particular afford increased regional benefits in comparison to toll roads.
Key Words: road infrastructure; municipalities; regional growth; sectoral analysis; geographic extension; least square models
La relación entre infraestructura carretera y crecimiento económico regional ha sido estudiada con resultados poco concluyentes. En este documento se analiza el efecto de la disponibilidad de infraestructura carretera sobre la producción regional de los municipios de México. Se caracterizaron regionalmente las carreteras disponibles por municipio aplicando un Sistema de Información Geográfica (SIG) y mediante un análisis de mínimos cuadrados ordinarios, y se explica la relación positiva densidad carretera- producción para las regiones municipales. Se demuestra que las carreteras tienen sus mayores efectos en la producción bruta regional de las actividades manufactureras y el comercio minorista, mientras que en la agricultura, la ganadería, la pesca y la minería, no tienen influencia significativa; particularmente las carreteras libres brindan mayor beneficio regional en comparación con las carreteras de peaje.
Palabras clave: infraestructura carretera; municipios; crecimiento regional; análisis sectorial; extensión geográfica; modelo de mínimos cuadrados
Clasificación JEL: H54; H76; R11; R15
1. INTRODUCTION
Infrastructure has been pointed out as an agent for economic growth. The increase or decrease of transportation networks affects the social and economic activities that a region can accept; nevertheless, the dynamics of how this growth is produced is one of the least understood areas in transportation, geography, urban economy, and regional science (Zhang and Levinson, 2007).
Even though there is an ample amount of literature, there is little consensus on the exact effects of investing in transportation infrastructures and regional development (see Chandra and Thompson, 2000, and Deng et al., 2014). The debate continues to be polemic in nature. Previous studies give different results, given the source of their data, the disaggregation and specifics of the model. In the majority of cases, the results assert a positive and significant impact, but other studies conclude that the impact is insignificant or significantly negative (Zepeda et al., 2017).
Furthermore, there is a two-part question that is worth exploring: on the one hand, the infrastructure for transportation and growth seems to maintain a perpetually polemic relationship of an inverse causality (IFMO, 2007); on the other hand, different levels of investment in infrastructure present different degrees of effects. This makes one think that the levels of infrastructure available in a region have a degree of efficiency in their economic effect, so new transportation infrastructure in regions which are well endowed do not exhibit the expected results. This in turn calls into question the need for new investments, leading us to the query of “whether transportation infrastructure can have a degree of efficiency in its impact” (Zepeda et al., 2017, p. 338).
This study focuses on determining the spatial effect of road infrastructures on regional growth at a municipal level in Mexico through the analysis of gross municipal production and sectorial analysis, especially in four economic sectors: Agriculture, Livestock and Fishing, Retail, Manufacturing and Mining, with the aim of determining if the available infrastructure can present an obstacle to regional growth, especially in poorer regions.
Different studies explain the effects of roads on growth, but they do so without considering the economic sectors and without using as sources of information the aggregates at a regional municipal level. In the particular case of Mexico, this is the first type of analysis which surveys and considers it possible to do so through the use of an SIG and an econometric analysis of ordinary least squares using geographical density interactive variables.
This study covers 2,456 municipalities with data from 2010, which was published in 2014 and which corresponds to the most recent Economic Census available. The census was carried out with details at the level of economic sectors in accordance with the most recently available geographic data at that moment and with information for each Municipality, the types of road and the number of lanes they possess. Finally, we determined the regions which are most lagging behind in the country in regards to road infrastructure and identified road density as a factor which explains the production of business entities dedicated to manufacturing and retail. It is not however possible to assert the same with regards to the sectors of agriculture, livestock and fishing, nor mining.
The study was organized in the following form: Section 2 has three parts, a review of the literature, the availability of road infrastructure in Mexico, as well as production in the country. In Section 3 we explain the methodology employed, the statistical data sources and how the road infrastructure stocks were determined based on an SIG, carrying out a separate description for toll roads as well as toll-free roads. We then consider the construction of the ordinary least squares models with interactive dummy variables (OLSIDV) for a global, regional, and sectorial analysis. The model’s functional test results are reviewed, as are the results from the econometric model. The results obtained analyzing regression coefficients are then reported in Section 4. Then we present the conclusions in which the positive relationship between the variables is demonstrated and recommendations based on the results are made.
2. REVIEW OF THE LITERATURE
The macroeconomic theory on endogenous growth develops an approach in which technology is an agent which affects economic growth. One interpretation of technology in an endogenous model considers it possible for production factors to have a public origin (Barro, 1990). In this fashion, the transportation infrastructures favor a technological change in the endogenous model and, as such, contribute to growth (Aschauer, 1989; Garcia-Mila and McGuire, 1992). In the opinion of Barro and Sala-i-Martin (2004), public capital can be whichever product or good, such as infrastructures, and can be analyzed as a private good provided by the government.
On the other hand, it has been observed that improvements in transportation lead to a reduction in the cost of businesses’ consumables as well as a greater productivity of factors, which leads to economies of scale at competitive levels which favor more productive businesses (Baldwin and Okubo, 2006).
From a spatial point of view, when transportation diminishes or decreases, distances produce economies of agglomeration in which economic agents benefit from being in proximity to other agents.
In the beginning, the businesses decide to make private investments in an open space and are essentially guided by the advantages and disadvantages afforded by each region and which are valued by the investor, who expects a return on their private capital. As such, private investments or businesses find themselves drawn to setting up in regions which have quality resources, infrastructure and innovative capacity, whose local businesses are susceptible to generating economies of scale and which produce goods and services which favor competition (Vazquez Barquero, 1997).
Siting decisions are affected by infrastructure and have a primary transportation component, as accessibility is a necessary condition for commercial activity. With high transportation costs, businesses’ siting is based on the need for proximity to their end clients. Based on this, it is possible to explain the formation of agglomerations and regions with higher levels of growth (Fujita et al., 2001), with the consequence of leaving other regions with diminished growth.
The discussion regarding the topic is vast, as better transportation conditions make it so that siting spreads out, reducing agglomeration; at the same time, improvements in transportation can make it so that economic agents can be closer together, thereby reducing costs and increasing their potential for interaction, which can lead to reaping the benefits of agglomeration over larger geographic areas (Eberts and McMillen, 1999).
Previous studies show that there are direct and indirect effects in the relationship of infrastructure as an engine for economic growth and that there is a positive influence among both variables (Moomaw and Williams, 1991; Garcia-Milà and McGuire, 1992; Rietveld and Nijkamp, 1992; Aschauer, 1998, Fujita et al., 2001; Démurger, 2001; Cantos et al., 2005; Berechman et al., 2006; Lall, 2007; Ozbay et al., 2007; Hong et al., 2011; Singh and Bhanumurthy, 2014). Nevertheless, the results display variations (Deng, 2013). For example, in the case of Aschauer (1998), he found that between infrastructure and growth, there was an elasticity between 0.39 to 0.56, and the results of the Garcia-Milà and McGuire (1992) present an elasticity of 0.04, Ozbay et al. (2007) found relationships between 0.017 and 0.206; while some others (Moomaw and Williams, 1991; Evans and Karras, 1994; Chandra and Thompson, 2000) found little evidence to support a hypothesis of economic growth led by transportation, with elasticities ranging from -0.35 to 0.178. The variations in the results from different empirical studies have different causes. Among these are the different levels of disaggregation for the data, methods for measuring the variables, and how the models were specified (Deng, 2013).
When it comes to regional studies, research found that in the case of China the impact of transportation on growth depends on the quantity and quality of pre-existing the roads: when road density is less than 0.17 km/km2, the relationship is insignificant, for densities between 0.17 and 0.38 km/km2, the impact is positive at 0.23 but upon exceeding the latter density, the impact drops to 0.09. As such a basic initial supply of roads or rural roads does not constitute a network and therefore does not act as an engine for the local economy. On the other hand, above a certain upper value, the positive effects tend to taper off significantly (Deng et al., 2013).
In the particular case of Mexico, the results indicate that the transportation infrastructure has a greater impact on intermediate regions than poor ones (Looney and Frederiksen, 1981), especially in the south-east of Mexico, as the impact on regional productivity arises when the transportation infrastructure links urban areas (Deichmann et al., 2004). However, the study at a municipal regional level, sectorial level, and regarding the different types of open and continuous roads is where this study is focused. These lines stay open as is recommended in studies which analyze research on the topic (Deng, 2013).
Road infrastructure in Mexico
The SCT1 (INEGI, 2014) reported that in 2010 there were 138,444 km of state and federal paved roads, proprietary and licensed (see Map 1). Toll roads, also known as licensed, are controlled by a financial institution (63.5%), private management (25.8%) state governments (5.9%), or the federal government (4.7%).

Source: created by the authors based on data reported by INEGI (2014).
Map 1 Road infrastructure by management type in 2010.
Table 1 shows the number of municipal regions in each federal entity, its surface area, the length of roads present and sorted by fees (toll or toll-free), by federal or state administration, as well as the number of business entities in all of the economic sectors which were analyzed. Also displayed is the density of total roads (length per unit area) and the density of business entities.
Table 1 Number of municipalities in the states making up the Mexican Republic, length and type of road infrastructure, business entities and densities.
State Region | Municipalities 2010 |
Area Km2 |
Length of roads 2010 (km) | Business Entities (BE) |
Density | ||||
State | Federal | Road | BE | ||||||
Toll-free | Toll | Toll-free | Toll | ||||||
Aguascalientes | 11 | 5 558 | 762 | - | 496 | - | 47 449 | 0.2263 | 8.54 |
Baja California | 5 | 73 551 | 914 | - | 1 350 | 463 | 95 882 | 0.0371 | 1.30 |
Baja California Sur | 5 | 73 970 | 636 | - | 1 429 | 23 | 28 114 | 0.0282 | 0.38 |
Campeche | 11 | 57 278 | 2 196 | 2 | 1 423 | 95 | 32 628 | 0.0649 | 0.57 |
Coahuila | 38 | 150 671 | 2 332 | - | 1 999 | 519 | 83 639 | 0.0322 | 2.11 |
Colima | 10 | 5 755 | 568 | - | 384 | 205 | 29 273 | 0.2010 | 0.39 |
Chiapas | 118 | 73 582 | 2 837 | - | 2 340 | 516 | 155 280 | 0.0774 | 0.56 |
Chihuahua | 67 | 246 969 | 2 453 | 155 | 1 528 | 1 300 | 97 044 | 0.0220 | 5.09 |
Mexico City | 16 | 1 487 | 69 | 15 | 30 | 35 | 415 481 | 0.1003 | 256.93 |
Durango | 39 | 122 131 | 1 795 | 76 | 2 295 | 800 | 50 452 | 0.0407 | 0.41 |
Guanajuato | 46 | 30 338 | 4 043 | 35 | 1 770 | 335 | 222 969 | 0.2038 | 7.35 |
Guerrero | 81 | 63 567 | 2 120 | 359 | 2 302 | 437 | 135 564 | 0.0821 | 2.13 |
Hidalgo | 84 | 20 655 | 2 506 | 9 | 1 196 | 145 | 98 567 | 0.1867 | 4.77 |
Jalisco | 125 | 77 964 | 3 348 | 10 | 2 139 | 1 060 | 313 013 | 0.0841 | 4.01 |
State of Mexico | 125 | 22 227 | 4 903 | 50 | 991 | 689 | 534 838 | 0.2984 | 24.06 |
Michoacán | 113 | 58 301 | 3 836 | - | 2 248 | 800 | 195 355 | 0.1181 | 3.35 |
Morelos | 33 | 4 861 | 1 018 | - | 308 | 347 | 84 651 | 0.3442 | 17.41 |
Nayarit | 20 | 27 817 | 1 275 | - | 1 327 | 458 | 46 958 | 0.1100 | 1.69 |
Nuevo León | 51 | 63 560 | 2 610 | 128 | 1 405 | 535 | 135 482 | 0.0736 | 2.13 |
Oaxaca | 570 | 93 960 | 1 563 | - | 3 733 | 581 | 177 954 | 0.0625 | 1.89 |
Puebla | 217 | 34 151 | 3 263 | 144 | 1 332 | 470 | 251 318 | 0.1525 | 7.36 |
Querétaro | 18 | 11 591 | 1 037 | - | 485 | 309 | 69 022 | 0.1580 | 5.95 |
Quintana Roo | 9 | 51717 | 1 621 | - | 1 014 | 181 | 45 488 | 0.0545 | 0.88 |
San Luis Potosí | 58 | 60 500 | 2 917 | 211 | 1 968 | 77 | 88 154 | 0.0855 | 1.46 |
Sinaloa | 18 | 56 803 | 2 919 | - | 924 | 1 033 | 93 242 | 0.0858 | 1.64 |
Sonora | 72 | 180 844 | 3 904 | 506 | 1 641 | 718 | 90 642 | 0.0374 | 0.50 |
Tabasco | 17 | 24 697 | 3 474 | - | 661 | 175 | 59 973 | 0.1745 | 2.43 |
Tamaulipas | 43 | 79 423 | 2 354 | - | 2 640 | 83 | 104 334 | 0.0639 | 1.31 |
Tlaxcala | 60 | 3 975 | 818 | 0 | 570 | 71 | 58 245 | 0.3670 | 14.65 |
Veracruz | 212 | 71 461 | 3 498 | 27 | 2 490 | 1 186 | 239 392 | 0.1008 | 3.35 |
Yucatán | 106 | 39 533 | 4 150 | - | 1 590 | 450 | 98 478 | 0.1566 | 2.49 |
Zacatecas | 58 | 74 479 | 2 508 | - | 2 086 | 235 | 51 864 | 0.0648 | 0.70 |
Total | 2 456 | 1 963 376 | 74 248 | 1 728 | 48 097 | 14 331 | 4 230 745 |
Source: Created by the authors based on the data reported by the INEGI and SCT
Regions and economic growth
Determining a geographic space for analysis or defining a region is a complex task due to a lack of unanimity when it comes to their demarcation (Marín, 2015).
Numerous studies have carried out political demarcations, contrary to other studies which analyze a smaller number of larger regions (See Deichmann et al., 2004; Hong et al., 2011 and Jiwattanakulpaisarn et al., 2011). In this study, the geographical spaces were demarcated using as regions for study the 2,456 municipalities which make up the 32 states in which the republic Mexican Republic was divided prior to 2016,2 as seen in Table 1. In this table we can see the average density of business entities in the macro regions (states) and the average road density of these, except for Mexico City, which has little road density and a high density of business entities. It is seen that the regions with lower road infrastructure density correspond to those with a lower business entity density.

Source: created by the authors with data from Table 1.
Figure 1 Road density by state region, except for Mexico City.
3. METHODOLOGY
Specifying the model
Macroeconomic theory for endogenous growth develops an approach, in which public infrastructure is believed to contribute to technological change, considering it a resource for economic growth, as such, various studies have employed a Cobb-Douglas type production function where investment in an infrastructure is interpreted as capital with positive investment returns (Aschauer, 1989; Garcia-Milà and McGuire, 1992; Garcia-Milà et al., 1996; Aschauer, 1998; Song and van Geenhuizen, 2014). We employed this function in part to test road infrastructure as an entry in production functions and in part thanks to the ease in comparing it to other studies. As such, the production function is specified as:
Where Y i is the production (total gross product) of spatial region i, A a technological improvement which affects the total of the function, K i is the capital stock and L i the labor force of region i, respectively.
Recent studies have successfully used proxy variables to quantitatively and qualitatively measure the characteristics of transportation infrastructures and to compare its impact on growth (Hong et al., 2011; Song and van Geenhuizen, 2014). As such, if we follow these models, we can incorporate the production function of capital stock using proxy variables for physical investment in road infrastructure through its length and the number lanes present in each municipal region.
In order to achieve this, the lengths of toll-free state roads (EL)3 were identified as were toll roads (EC), as well as those which were toll-free federal roads (FL) and toll federal roads (FC) and with their respective available lanes. It was determined that length L i corresponds to each municipality i and said length was affected by the number of lanes n, and by the inverse of geographical area S i in order to obtain the density for each type of road in each region, which was used as a proxy variable of capital stock. For that we employed the following equations:
Where i is the region for analysis; n is a number of available lanes in a length of roads within the region; D_EL is the density of toll-free state roads; lEL refers to the length of toll-free state roads; D_EC refers to the density of toll state roads; lEC refers to the length of toll state roads; D_FL refers to the density of federal toll-free roads; lEL refers to the length of federal toll-free roads; D_FC refers to the density of federal toll roads; and lFC refers to the length of federal toll roads.
Regarding the labor force, it was considered as the occupied personnel P i in the business entities in the region. Furthermore, the investment Inv i carried out by business entities of the region was incorporated as capital, as was the value of fixed assets Act i .
The production function in the region i ends up being:
Through the use of linearization in logarithmic terms for the production function, we can obtain an equation whose parameters can be determined by the use of a least ordinary squares model:
Data
A data table was created with the value of variables for the period of 2010, based on the data published by the Economic Census and in the National Geo-statistical Framework for the 2,456 municipalities. This was due to limitations of data at municipal levels and the characteristics of roads which would allow the construction of chronological series or a data panel.
The data of the business entities found in each municipality, their gross production, employed workforce, investment and fixed assets, were grouped together according to their economic activity in order to give results which are worth mentioning in relationship to retail, agriculture, livestock and fishing, manufacturing and mining.
In order to analyze the length of roads in each municipality, and SIG was used in order to determine the length of each road segment within each municipal region (L i ). This length was affected by characteristics such as lanes in each segment and by the geographical area of each region in order to obtain density as was previously explained. In table 2 we can see the descriptive statistics for the variables in the model: Y (millions of pesos), P (thousands), Inv (millions of pesos), Act (millions of pesos), and the densities (km/km2)DELi, DECi, DFLi, DFCi
Table 2 Descriptive statistics for the variables employed in the model.
Variable | Obs. | Avg. | STDEV. | Min | Max |
(Y) Prod_bruto (milions of pesos) | 2 456 | 5 693.939 | 34 575.49 | 0.103 | 792 318.9 |
P (thousands) | 2 456 | 8 785.162 | 39 769.32 | 5 | 733 557 |
Act (milions of pesos) | 2 456 | 3 286.941 | 44 739.94 | 0.02 | 2 082 621 |
Inv (milions of pesos) | 2 456 | 224.291 | 2 345.46 | -2 108.222 | 9 0795.27 |
Densities (km/km²) | |||||
DELi | 2 456 | 0.0001281 | 0.0001709 | 0.0000000 | 1.6388630 |
DECi | 2 456 | 0.0000022 | 0.0000225 | 0.0000000 | 0.5437317 |
DFLi | 2 456 | 0.0000696 | 0.0001251 | 0.0000000 | 1.6672872 |
DFCi | 2 456 | 0.0000241 | 0.0000984 | 0.0000000 | 1.8893679 |
Note: aTl: "Gross product", kept in the original Spanish to maintain consistency with other tables and equations.
Source: created by the authors base don the results from the calculations carried out.
The total gross product (Y) in the regions is shown in Map 2, while in Map 3 are the densities accumulated by the road infrastructure in each municipal region where the concentration of value of variables in clusters is observed.
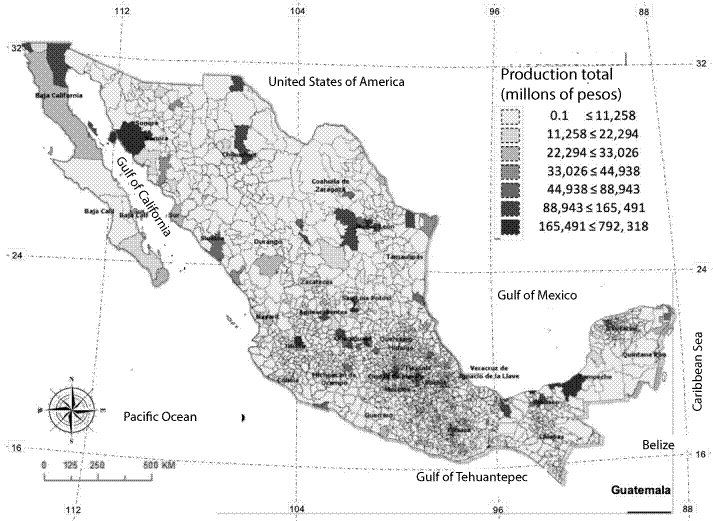
Source: created by the authors with data from the INEGI (2014)
Map 2 Spatial distribution of total production at a regional level in 2010.

Source: created by the authors using the values determined in Table 2
Map 3 Spatial distribution of road density in Mexico, 2010
Sample data was applied in order to guarantee a reliability of the regression results. The general specification test for the lineal regression model (Ramsey RESET test) verified whether the nonlinear combinations of adjusted values help to explain response variable (the results from the test are seen in Table 3).
Table 3 Results from the model specification test (Ramsey)
Index | Determined Value |
F (3, 2445) = | 2.0500 |
Prob > F = | 0.1045 |
Ho: the model has no omitted variables.
Source: created by the authors.
In order to contrast the homoscedasticity a Breusch-Pagan test was used. The results are shown in Table 4.
Table 4 Results from the Breusch-Pagan test
Index | Determined Value |
chi2(1) = | 2.2200 |
Prob > Chi2 = | 0.1363 |
Ho: the variance is constant.
Source: created by the authors.
The testing showed a high value of p (0.1363), which suggests that one cannot discard the null hypothesis that the residuals are homoscedastic. With the aim of validating the supposed normality in distribution of the disturbances, that is that the errors have normal distribution, a Jarque-Bera test was carried out. In Table 5 we can see how the probabilities are less than one and the null hypothesis is rejected. As such, the errors are not distributed in a normal fashion; however, we consider the sample sufficiently large (2,456 obs.)
4. RESULTS
An analysis using ordinary least squares models was carried out in order to determine the coefficient of the variables (the results can be seen in Table 6).
Table 6 Regression results via an OLS model using regional observations of total production in all economic sectors
lnProd_bru~t | Coef. | P >| t | | Prob > F = | 0.0000 |
ln_PerOCU | 0.4010 | 0.0000*** | Adj.R-squared = | 0.9588 |
ln_inver | 0.0699 | 0.0000*** | R-squared = | 0.9589 |
ln_ACtFij | 0.6676 | 0.0000*** | ||
ln_D_FL | 0.0088 | 0.0000*** | ||
Ln_D_FC | 0.0121 | 0.0000*** | ||
Ln_D_EL | 0.0135 | 0.0000*** | ||
Ln_D_EC | 0.0174 | 0.0200* | ||
_cons | -1.248431 | 0.0000*** |
Note: *statistically significant at the 95%, ***statistically significant at 99%
Source: created by the authors.
The elasticities obtained turned out to be statistically significant at 99% for the most part, except for toll state road density which is significant at 95%. The fixed assets of business entities provide a greater impact on total gross production (0.6676) for regions where they are found, secondly, we identified the employed workforce (0.4010), and thirdly, the investment realized (0.0699), the roads have a smaller positive impact.
Likewise, the elasticity of state road density, both toll and toll-free (0.0121 and 0.0088 respectively), is greater than that obtained for federal road density including both toll and toll-free (0.0174 and 0.0135 respectively).
In order to observe the effect that roads have, in particular economic sectors, an OLSIDV regression was carried out with the set of business entities from all economic sectors as only four, Agriculture, Livestock and Fishing, Retail, Manufacturing and Mining, presented results worth mentioning and can be seen in Table 7.
Table 7 Disaggregated effects for variables on the dependent for different economic sectors via an OLSIDV model.
Agriculture, Livestock and Fishing | Retail | Manufacturing | Mining | ||||||
Breusch y Pagan | Prob > F = | 0.0003 | 0.0000 | 0.0000 | 0.0002 | ||||
Ramsey | Prob > F = | 0.2291 | 0.7901 | 0.0003 | 0.5007 | ||||
Obs. | 483 | 2433 | 2302 | 186 | |||||
Prob > F | 0.0000 | 0.0000 | 0.0000 | 0.0000 | |||||
Adj.R-squared | 0.8133 | 0.9565 | 0.9562 | 0.8437 | |||||
R-squared | 0.816 | 0.9566 | 0.9563 | 0.8496 | |||||
lnProd_bru~t | Coef. | P>|t| | Coef. | P>|t| | Coef. | P>|t| | Coef. | P>|t| | |
ln_PerOCU | 0.7111 | 0.0000*** | 0.6383 | 0.0000*** | 0.4975 | 0.0000*** | 0.6235 | 0.0000*** | |
ln_inver | 0.0196 | 0.3510 | 0.1038 | 0.0000*** | 0.0294 | 0.0000*** | 0.0121 | 0.7430 | |
ln_ACtFij | 0.4448 | 0.0000*** | 0.4330 | 0.0000*** | 0.6342 | 0.0000*** | 0.5857 | 0.0000*** | |
ln_D_FL | 0.0018 | 0.9300 | 0.0284 | 0.0000*** | 0.0139 | 0.0200* | 0.0361 | 0.3730 | |
Ln_D_FC | -0.0085 | 0.7370 | -0.0039 | 0.5500 | 0.0305 | 0.0000*** | 0.0277 | 0.5200 | |
Ln_D_EL | -0.0536 | 0.0620* | 0.0299 | 0.0000*** | 0.0344 | 0.0000*** | 0.0143 | 0.7870 | |
Ln_D_EC | 0.0211 | 0.7640 | 0.0069 | 0.6760 | 0.0284 | 0.1700 | 0.1206 | 0.1560 | |
_cons | -2.3295 | 0.0000*** | -2.2249 | 0.0000*** | -1.0447 | 0.0000*** | -1.8356 | 0.0000*** |
Note: *Statistically significant at 95%, ***statistically significant at 99%.
Source: created by the authors with the results obtained from the analysis via Stata.
In the previous table, we can see that the coefficients obtained for the primary sector are less significant when compared against the results from the global regression. In this case, only the referents for the employed workforce (0.7111) and fixed assets (0.4448), are statistically significant at 99% so that it is not possible to assert from the results that road density has an effect on agricultural production.
This behavior is repeated in the mining sector where applied regression coefficients applied to the observations are for the most part not significant in the case of road infrastructure and only the referent for the employed workforce (0.6235) and fixed assets (0.5857) are statistically significant at 99%. This can be explained due to mining production depending fundamentally on the personnel employed and assets which companies employ in mining extraction. In this case the siting of business entities is not determined by the presence of road infrastructures but natural veins and deposits.
In the case of economic activities identified as Retail and Manufacturing we can observe that the regression coefficients are positive and for the most part statistically significant at 99%. Upon evaluating the total gross product of the business entities in the retail sector, the elasticity of the employed workforce is greater than that for manufacturing, which is in contrast to the elasticity found in manufacturing where the elasticity of fixed assets is greater (0.6342) than it is in retail (0.4330). This means that in manufacturing, the companies’ fixed assets provided a greater effect for regional growth.
The impact of toll-free road density, both state and federal, on commerce turn out to be positive (0.0284 and 0.0299, respectively) and statistically significant at 99%, unlike the density of toll roads, which are not statistically significant. This makes one think about the tunneling effect that toll roads can come to have on the sector.
With regards to the impact on manufacturing production due to toll-free road density, both state and federal, turn out to be positive (0.0139 and 0.0344 respectively) as well as that of federal toll road density (.0305), given that all of them are statistically significant at 99%. One can see that toll-free roads have a greater positive relationship with the production of manufacturing companies than toll roads as the use of the latter generates cost for the companies which must be evaluated and can restrict competitiveness, which hinders growth rather than facilitate it.
In order to consider the effects that roads can have in urban areas on production in these regions, an analysis was carried out, taking into consideration dummy variables with the classification of municipalities according to the size of the regions: ME4 , Metropolitan (more than 50% of the population resided in locations with more than 1 million inhabitants), LU, large urban area (more than 50% of the population resides in locations between 100,000 and less than 1 million inhabitants), MU, medium urban region (more than 50% of the population lives in locations between 15,000 and less than 100,000 inhabitants), SU, semi urban (more than 50% of the population resides in locations between 2500 and less than 15,000 inhabitants), RU, Rural (more than 50% of the population lives in locations with less than less than 2500 inhabitants), MI, mixed (populations are distributed among the aforementioned categories with no one category accounting for more than 50% of the population).
Likewise, the consolidation of municipal regions in macro regions were analyzed, taking into consideration the regions defined by the INEGI for Mexico: Northwest, Northeast, West, East, North-Central, South-Central, Southwest, and Southeast. In most cases no results were found that were worth mentioning.
Discussions
Within a municipal region, the density of toll-free state road network (D_EL), toll state roads (D_EC), toll-free federal (D_FL) and federal toll roads (D_FC) have a positive influence on the total gross production of the business in said regions. Nevertheless, they are not elements with greater influence when compared to other fixed assets, the workforce employed, and the investments which have a greater positive influence. At the municipal regional level, we can see that some economic sectors benefit more from the roads.
In the farming and mining sectors there is a similar behavior, as road density does not present a statistically significant positive influence on gross production as the sectors are more driven from the location of production centers when it comes to farming and in the case of mining the from locations which provide Hydro resources, productive land, veins and metal and non-metallic mineral deposits.
In contrast, the effects from road density on the retail sector's production have been able to be determined. The greatest impact on production in municipal regions is found related to toll-free state roads and federal toll-free roads as their use between population centers and localities, allow access to administrative, financial, commercial, and retail services for the inhabitants of said regions, as well as constructing a network providing access from other federal entities without adding an excessive cost for their use.
In the case of manufacturing toll-free state roads allow a low-cost flow of consumables and the outflow of final products to other regions in adjoining federal entities. It is under these conditions that toll roads come to be significant, possibly from the businesses’ need to transport their high value final products with better speed, handling, and safety.
We see the construction of high productivity clusters in key municipalities due to them having very particular use cases thanks to their location and economic history: Tijuana in Baja California (manufacturing sector, thanks to its proximity to the United States of America), Guaymas in Sonora (seafood based food manufacturing and production thanks to its port), Ciudad del Carmen in Campeche (oil exploitation), Paraiso in Tabasco (oil exploitation), Salina Cruz in Oaxaca (oil derived industry), Mexico City (economic and political centralization), Guadalajara in Jalisco and Monterey in Nuevo Leon (manufacturing industry), just to name a few.
In Map 3, we can see a central/radial makeup for road density for all the roads, placing a higher density of roads in the center of the country (with Mexico City acting as the point of origin) and creating a horizontal path from the port region of Colima and Michoacan and from Mexico City towards the ports of Veracruz and Tuxpan in the state of Veracruz. In a vertical sense, one can see the construction of the path from Mexico City to the US border which goes through Nuevo Leon. It is also worth mentioning the high-density roads in the region of Merida, Yucatán, thanks to its proximity to the port of Progreso, and from Reforma in Chiapas to the ports of Dos Bocas and Frontera as they go through Villa Hermosa in Tabasco and connect with Ciudad del Carmen in Campeche. These last ones are cities whose economic activity is related to petroleum.
5. CONCLUSIONS
The availability of road infrastructures has a positive influence on the municipal regional economic growth by affecting production. The effects of the road infrastructure are greater when they are made available to production agents without a fee (toll), as this allows the creation of competitive conditions at a regional level by reducing the costs which transportation implies, as well as the diversification in siting and diminishing further concentration in already favored regions.
The effects of investing in road infrastructure are different depending on the economic sector, as the farming and mining sectors are the ones which benefit the least. While the industrial sector sees the greatest benefits.
As such, the endowment of the infrastructure should be linked with public policies and projects which boost the expectation, economic use, and social benefit of the infrastructure and relocate economic activity and urban development.
As for the effects of road density on other economic sectors, econometric analyses were carried out with the three models without finding consistent or significant results worth mentioning. Nevertheless, it is recommendable to deepen the study with another methodology as well as extending the period to be analyzed in this current study. One temporal study could provide elements for a greater understanding when evaluating the relationships between the variables in the long term, especially as has been pointed out by other authors (Jiwattanakulpaisarn et al., 2011), a study with dynamic effects could provide relevant empirical results. As such, the integration of municipal into larger or macro-regions is a line of study which is left open for future study as the methodology employed and the data available were inadequate to properly capture these effects.
REFERENCES
Aschauer, D. (1989), “Is public expenditure productive?”, Journal of Monetary Economics, vol. 23, núm. 2, Holanda, Elsevier, marzo. [ Links ]
______(1998), “The role of public infraestructure capital in mexican economic growth”, Economía Mexicana, Nueva Epoca, vol. VII, núm. 1, MéxicoCentro de Investigación y Docencia Económicas, primer semestre. [ Links ]
Baldwin, R. y Okubo, T. (2006), “Agglomeration, offshoring and heterogenous firms,” CEPR Discussion Paper No. 5663, London, Centre for Economic Policy Research. [ Links ]
Barro, R. J. (1990), “Government spending in a simple model of endogenous growth”, Journal of Political Economy, vol. 98, núm. 5, part 2, USA, The University of Chicago Press, octubre. [ Links ]
Barro, R. J. y Sala-i-Martin, X. (2004), Economic growth, USA, MIT Press. [ Links ]
Berechman, J., Ozmen, D. y Ozbay, K. (2006), “Empirical analysis of transportation investment and economic development at state, country and municipality levels”, Transportation, vol. 33, núm. 6, USA, Springer Science+Business Media, noviembre. [ Links ]
Cantos, P., Gumbau-Albert, M. y Maudos, J. (2005), “Transport infrastructures, spillover effects and regional growth: evidence of the Spanish case”, Transport Reviews, vol. 25, núm.1, United Kingdom, Taylor & Francis, febrero. [ Links ]
Chandra, A. y Thompson, E. (2000), “Does public infrastructure affect economic activity? evidence from the rural interstate highway system”, Regional Science and Urban Economics, vol. 30, núm. 4, Holanda, Elsevier , julio. [ Links ]
Deichmann, U., Fay, M., Koo, J. y Lall, S. (2004), “Economic structure, productivity, and infrastructure quality in southern Mexico”, The Annals of Regional Science, vol. 38, núm. 3, USA, Springer, septiembre. [ Links ]
Démurger, S. (2001), “Infrastructure development and economic growth: an explanation for regional disparities in China?”, Journal of Comparative Economics, vol. 29, núm. 1, Holanda, Elsevier , septiembre. [ Links ]
Deng, T. (2013), “Impacts of transport infrastructure on productivity and economic growth: recent advances and research challenges”, Transport Reviews, 33(6). [ Links ]
Deng, T., Shao, S., Yang, L. y Zhang, X. (2014), “Has the transportled economic growth effect reached a peak in China? A panel threshold regression approach”, Transportation , vol. 41, núm. 3, USA, Springer Science+Business Media, mayo. [ Links ]
Eberts, R. y McMillen, D. (1999), “Agglomeration economies and urban public infrastructure”, en P. Cheshire y E. Mills, Handbook of Regional and Urban Economics, 3. [ Links ]
Evans, P. y Karras, G. (1994), “Is government capital productive? evidence from a panel of seven countries”, Journal of Macroeconomics, vol. 16, núm 2, Holanda, Elsevier , Summer. [ Links ]
Fujita, M., Krugman, P. y Venables, A. (2001), The spatial economy: cities, regions and international trade, USA, MIT Press . [ Links ]
Garcia-Milà, T. y McGuire, T. (1992), “The contribution of publicly provided inputs to states’ economies”, Regional Science and Urban Economics , vol. 22, núm. 2, Holanda, Elsevier , junio. [ Links ]
______, McGuire, T. y Porter, R. (1996), “The effect of public capital in state- level production functions reconsidered”, Reviews on Economics Statistics, vol. 78, núm. 1, USA, Elsevier, marzo. [ Links ]
Hong, J., Chu, Z. y Wang, Q. (2011), “Transport infrastructure and regional economic growth: evidence from China”, Transportation , vol. 38, núm. 5, USA, Springer , septiembre. [ Links ]
Institut für Mobilitätsforschung (IFMO) (2007), Transport, trade and economic growth. Coupled or decoupled?, Alemania, Springer-Verlag Berlin Heidelberg. [ Links ]
Instituto Nacional de Estadística Geografía e Informática (INEGI) (2014), Anuario Estadístico y Geográfico de los Estados Unidos Mexicanos 2014, México, Instituto Nacional de Estadística Geografía e Informática. [ Links ]
Jiwattanakulpaisarn, P., Noland, R. y Graham, D. J. (2011), “Highway infrastructure and private output: evidence from static and dynamic production function models”, Transportmetrica, 7 (5). [ Links ]
Lall, S. (2007), “Infrastructure and regional growth, growth dynamics and policy relevance for India”, The Annals of Regional Science, vol. 41, núm. 3, Berlín-Heidelberg, Alemania, Springer, septiembre. [ Links ]
Looney, R. y Frederiksen, P. (1981), “The regional impact of infrastructure investment in Mexico”, Regional Studies, vol. 15, núm. 4, United Kingdom, Routledge, noviembre. [ Links ]
Marín, C. (2015), “Anotaciones en torno al concepto de región”, Nimbus, vol. 67, núm. 11-12, Almería, Universidad de Almería. [ Links ]
Moomaw, R. y Williams, M. (1991), “Total factor productivity growth in manufacturing: further evidence from the States”, Journal of Regional Science, vol. 31, núm. 1, USA, Wiley-Blackwell, febrero. [ Links ]
Ozbay, K., Ozmen-Ertekiny, D. y Berechman, J. (2007), “Contribution of transportation investments to country output”, Transport Policy, vol. 14, núm. 4, United Kingdom, Elsevier, julio. [ Links ]
Rietveld, P. y Nijkamp, P. (1992), “Transport and regional development”, Research Memorandum, núm. 50, Serie Research Memoranda, VU University Amsterdam, Holanda, diciembre. [ Links ]
Singh, P. y Bhanumurthy, N. R. (2014), “Infrastructure development and regional growth in India”, en G. Ambar y K. Asim (coords.), Analytical Issues in Trade, Development and Finance, India, Springer. [ Links ]
Song, L. y van Geenhuizen, M. (2014), “Port infrastructure investment and regional economic growth in China: panel evidence in port regions and provinces”, Transport Policy , vol. 36, United Kingdom, Elsevier, noviembre. [ Links ]
Vázquez Barquero, A. (1997), “Gran empresa y desarrollo endógeno: la convergencia estratégica de las empresas y territorios ante el desafío de la competencia”, EURE, 23(70). [ Links ]
Zepeda, I., Ángeles, G. y Carrillo, D. (2017), “Infraestructura portuaria y crecimiento económico regional en México”, Economía, sociedad y territorio, vol. 17, núm. 54, México, El Colegio Mexiquense, mayo-agosto. [ Links ]
Zhang, L. y Levinson, D. (2007), “The economics of transportation network growth”, en P. Coto-Millán y V. Inglada (eds.), Essays on Transport Economics, Germany, Physica-Verlag HD. [ Links ]
3Tl: from the original Spanish, EL=estatal libre, EC=estatal cuota, FL=federal libre, FC=federal cuota. These abbreviations will be incorporated elsewhere throughout the paper, at times in conjunction with Density D.
Received: August 31, 2018; Accepted: January 09, 2019