Services on Demand
Journal
Article
Indicators
-
Cited by SciELO
-
Access statistics
Related links
-
Similars in SciELO
Share
Ciencias marinas
Print version ISSN 0185-3880
Cienc. mar vol.41 n.4 Ensenada Dec. 2015
https://doi.org/10.7773/cm.v41i4.2492
Review article
The 16S rRNA gene in the study of marine microbial communities
1Centro de Investigación en Alimentación y Desarrollo, PO Box 1735, Hermosillo CP 83000, Sonora, México
New sequencing technologies and analytical capabilities have stimulated the study of microbial communities from specific environments, enabling researchers to understand the complexity of those systems. The 16S rRNA gene has proved very useful in describing the diversity and characterization of marine microbial communities, particularly of uncultivated organisms. The development of new sequencing techniques has contributed to the exponential increase in the number of reported 16S rRNA sequences as barcodes for microorganisms, forcing a review of concepts and methods for the taxonomic classification of these organisms. Manipulation and analysis of large amounts of genetic information have prompted the development of specific databases, specialized algorithms, and computational tools to compare thousands of such sequences and make a taxonomic assignment. Complete 16S rRNA sequences are thus needed for accurate and reproducible taxonomy assignment in the study of marine bacterial communities.
Key words: metagenomics; marine microbial communities; 16S rRNA databases
Las nuevas tecnologías de secuenciación y capacidades analíticas han estimulado el estudio de las comunidades microbianas de ambientes específicos, lo cual ha permitido conocer la complejidad de estos sistemas. El gen ARNr 16S ha demostrado ser de gran utilidad para describir y caracterizar las comunidades microbianas marinas, especialmente los organismos no cultivados. Las nuevas técnicas de secuenciación han contribuido al incremento exponencial de registro de secuencias, aunque parciales, del ARNr 16S como elemento de código de barras para microorganismos. Consecuentemente, ha sido necesaria una revisión de conceptos y métodos de clasificación taxonómica para estos organismos. El manejo y análisis de una gran cantidad de información génica han impulsado el desarrollo de bases de datos específicas, algoritmos y herramientas computacionales especializadas para comparar miles de secuencias semejantes y hacer la asignación taxonómica. Por lo tanto, las secuencias completas del ARNr 16S son necesarias para una asignación taxonómica certera y reproducible en los estudios de comunidades microbianas marinas.
Palabras clave: metagenómica; comunidades microbianas marinas; bases de datos ARNr 16S
Introduction
The oceans, the world's largest ecological system, are inhabited by appproximately 3.6 × 1028 microorganisms at a mean density of 5 × 105 cells mL-1 (Cock et al. 2010). Having originated in a similar environment, marine microbial communities are highly abundant and diverse and have developed successful physiological adaptations (Hellweger et al. 2014).
The importance and impact of microbial communities in a specific environment have been demonstrated by studies of the microbiota that inhabit the human body. The human microbiome is a reservoir of approximately 109 microorganisms. There are ten times more microbes in the human body than human cells, and the microbiological community thus plays a major role in human health and disease (NRC 2007). The highest microbial densities are found in the human gastrointestinal tract (Ley et al. 2006), and the identification of 3.3 million different sequences in fecal samples from 124 individuals revealed that the microbiota of the human gut contains at least 160 of the 1000-1150 possible bacterial species (Olsen et al. 2012).
Oceans are the most extensive environments on Earth and microorganisms are the most predominant in both biomass and metabolic activity. The study of the composition and dynamics of marine microbial communities is therefore of the utmost importance (Fuhrman et al. 2015). In oceans, microorganisms capture and convert solar energy, catalyze key biogeochemical transformations of nutrients and trace elements that support productivity, participate in the regulation of greenhouse gases, and represent a vast reservoir of genetic diversity (Karl 2007, Karl and Church 2014). In the aquaculture industry, the microbial communities of bioflocs have become excellent tools for controlling water quality and improving production (Crab et al. 2012).
Marine microbial communities
Far-reaching projects have been developed to analyze microbial groups and understand the marine environment. In the Sargasso Sea shotgun sequencing project, the random sequencing of microbial cell and virus samples allowed the identification of more than one million protein-coding genes (Venter et al. 2004, Sjöstedt et al. 2014). This project was followed by the Global Ocean Sampling Expedition, probably the largest sequencing project undertaken to build a meta-genomic library, and more than 148 new phylotypes and 69,901 new genes have been identified (Rusch et al. 2007, Barberá et al. 2012). In the Hawaii Ocean Time-series program, observations carried out over a five-year period at Station ALOHA (10-4000 m depth) allowed the description of new genes (Karl and Church 2014). The International Census of Marine Microbes (ICoMM), one of 14 censuses of marine life supported by several research laboratories, aims to determine the range of genetic diversity and distribution of different microorganisms in the oceans. It comprises 356 datasets of sequences from the V6 region of the bacterial 16S rRNA gene obtained from studies of 40 different biomes, from pelagic and benthic regions to mangrove forests and sponge-associated bacteria (Amaral-Zettler et al. 2010, Karsenti et al. 2011, Zinger et al. 2012). Based on studies of samples collected at 210 diverse oceanic sites and depths of 2000 m, the multinational consortium Tara Oceans has generated the Ocean Microbial Reference Gene Catalog comprising 35,650 taxonomically different organisms (Karsenti et al. 2011, Logares et al. 2014, Sunagawa et al. 2015).
Other studies have searched for a correlation between the genomic properties of 16S of microbiomes and environmental conditions. The results, from different oceanic regions, indicate that temperature is the most important factor determining the composition of microbial communities in the epipelagic zone (Sunagawa et al. 2015).
The studies of marine microbial communities can be separated according to the characteristics of the environment they inhabit:
Pelagic planktonic microbial communities. Studies have focused on determining the global patterns of microbial community composition. The findings indicate that the same taxa are present in all the oceans. That observed in one region at a given time are the changes in the relative abundance of community members (Gibbons et al. 2013). In addition to qualitative descriptions, studies have also addressed seasonal, interannual, and interseasonal variations (Hatosy et al. 2013, Karl and Church 2014), and considerable advances have been made in understanding the metabolic processes of the communities and their role in biogeochemical cycles (Hahnke et al. 2013, Brown et al. 2014, Karl and Church 2014, Klindworth et al. 2014, Logares et al. 2014).
Coastal planktonic microbial communities. Regardless of the oceanic influence, changes in the community structure have been attributed to suspended particles from rivers, runoff, and upwelling (Boeuf et al. 2013, Ameryk et al. 2014, Satinsky et al. 2014, Aylward et al. 2015, Mueller et al. 2015). In these environments it is possible to distinguish communities attached to organic matter from free-living communities (Smith et al. 2013, Bižić-Ionescu et al. 2014, Mohit et al. 2014, Simon et al. 2014).
Benthic microbial communities. These communities are very dynamic and the most diverse of the marine communities, most likely because of the high rates of population exchange promoted by the nutrients, environmental conditions, and substrate heterogeneity in the benthic zone (Ramette et al. 2009, Miller et al. 2013, Gobet et al. 2014). They are capable of nitrification and anaerobic ammonium oxidation (Prabavathi and Mathivanan 2012, Wang et al. 2012, Laverock et al. 2014, Lipsewers et al. 2014, Vigneron et al. 2014, Bowen et al. 2015), which allows the reuse of organic nitrogen.
Microbial communities related to submarine volcanoes (fumaroles). These communities are representative of extreme environments and do not show a high diversity. The members of the main taxonic groups, however, show greater variability in the 16S sequences, a reflection of the great evolutionary pressure exerted by the environment (Biddle et al. 2006, Havelsrud et al. 2011, Roussel et al. 2011, Tang et al. 2013, Shao et al. 2014, Kato et al. 2015).
16S rRNA
In order to have a standardized tool for the identification of organisms inhabiting different environments, Hebert et al. (2003) proposed the so-called DNA barcode for use in the fields of ecology, systematics, and evolutionary biology. The idea was to create a fast, reliable, and reproducible method based on the amplification of a standardized DNA region by polymerase chain reaction (PCR), and the proposed region was a 600 base-pair fragment of mitochondrial DNA encoding cytochrome c oxidase subunit I (COI). The COI region proved to be a valuable tool for the taxonomic classification of many animals and even species, but its use was limited in taxonomic and phylogenetic studies of plants, fungi, and microorganisms (Blaxter 2004, Lebonah et al. 2014). It thus became necessary to find other candidate genes or sequences that could be used as markers.
In general, to be considered a molecular marker for barcoding studies and other taxonomic or evolutionary studies, the DNA region must possess the following characteristics: (a) have significant genetic variability and divergence at species level; (b) have conserved sites for designing universal primers for PCR amplification; and (c) have an appropriate length that will allow, with current capabilities, easy, reproducible, and precise extraction and sequencing (Kress and Erickson 2012). Several genes or sequences were proposed as markers, but 16S ribosomal ribonucleic acid (16S rRNA), originally proposed by Pace et al. (1986), was considered a good option for the classification of bacteria. It was rapidly adopted by the scientific community and has been used to develop specialized databases. The 16S rRNA sequences have thus become an important tool for reconstructing phylogenetic relationships. Moreover, the use of 16S rRNA sequences led to the establishment of the All-Species Living Tree Project, which has become a reference of relationships among prokaryotes easily organized in dynamic databases that compile and treat the data of all the available 16S rRNA gene sequences (Yarza et al. 2008, 2010). Despite some controversies and technical difficulties, the 16S rRNA gene is still used as a molecular marker and, in view of the advantages of novel genomic techniques, new study strategies have been suggested (Savolainen et al. 2005, Tanabe and Toju 2013). Owing to the rapid generation of genomic information and the characterization of the 16S rRNA sequences, recent years have seen important changes in the identification of bacterial species and an accelerated assignation of species.
Characteristics of the 16S rRNA gene
The 16S rRNA gene is a polyribonucleotide of approximately 1500 nucleotides, encoded by the gene rrs, also called 16S ribosomal DNA. Like any single-chain nucleotide sequence, 16S rRNA folds and acquires a secondary structure, with alternating double-chain and single-chain segments forming approximately 50 helices. This molecule is considered a powerful universal marker because it is found in all known organisms. Its structure is apparently maintained over long periods and as its function has not changed, the changes in the sequence are likely random. In its eukaryotic counterpart, 18S rRNA, mutations are acquired slowly, so it is possible to obtain information about all organisms throughout the evolutionary scale. Nonetheless, rRNA sequences have sufficient variability to differentiate not only the most distant organisms but also the nearest, and it is possible to differentiate species, strains, and varieties. Moreover, the relatively long size of 16S rRNA (1500 nucleotides) minimizes statistical fluctuations, while the conservation of its secondary structure allows precise alignment during the comparison of sequences (Rodicio and Mendoza 2004). The 16S rRNA gene contains nine less-conserved or hypervariable regions (V1-V9) (Baker et al. 2003), and it is these regions that contribute the most useful information for phylogenetic and taxonomic studies. The conserved regions, on the other hand, are very useful for designing universal primers that allow the amplification of the different hypervariable regions of the 16S rRNA genes of microorganisms found in a community.
While the use of universal primers has undoubtedly favored the detection and analysis of sequences, some authors indicate that they are deficient in detecting a considerable number of uncultured bacterial species from environmental samples (Baker et al. 2003, Huws et al. 2007). Several studies have reported the coverage of known universal primers and their combinations with sequences obtained from metagenomic studies. For example, an in silico analysis revealed that the V4/V5 region was the most accurate for the classification of bacterial sequences from intestinal microbiota, whereas the V7/V8 region was the least accurate (Liu et al. 2007, 2008).
Hypervariable regions
With the advent of massive sequencing methods there was an important increase in the number of reports on the characterization of bacterial communities using the 16S rRNA gene as marker. Since only partial sequences are obtained from different variable regions, however, the discrepancies in the results promoted comparative studies between some variable regions and the full-length gene (Nelson 2011, Sun et al. 2013).
Diversity indices are one way of evaluating bacterial communities, and the ability of the primer pairs and the region of the 16S rRNA gene that they amplify will strongly influence the description of the bacterial diversity of environmental samples. For example, in an analysis of microbial populations in sediments, Miller et al. (2013) observed that the estimated diversity was lower and that the number of operational taxonomic units (OTUs) that could not be classified, even at phylum level, increased from 8.6% to 34.6% when the V3 region was used as taxonomic tool instead of the complete sequence. In a characterization of microbial communities in wastewater, the information obtained with a fragment containing the V1 and V2 regions proved insufficient to detect organisms belonging to the phyla Verrucomicrobia, Planctomycetes, and Chlamydiae (Cai et al. 2013). In another study analyzing samples from the human gut and deep-sea vents, differences were observed in the diversity found for the 16S rRNA regions used: 42 taxa were detected in the V3 region and only 26 in the V6 region (Huse et al. 2008).
Despite these inconveniences, the 16S rRNA gene continues to be the most commonly used marker to understand bacterial communities in all environments. In view of the differences in primer specificities, sometimes highly specific for a spectrum of bacterial species, some authors recommend the combined use of different primer sets, different DNA extraction methods, and deep sequencing of the bacterial community (Tringe and Hugenholtz 2008, Hong et al. 2009, Wang and Qian 2009).
Metagenomics, one of the new "omics" research fields, arose out of the interest of studying bacterial communities of specific environments in an integrated manner. Diverse approaches and methodologies are used to understand the functions of a microbial community. The term meta means to transcend and in metagenomics it represents a strategic concept that includes research at three interrelated levels (sample processing, DNA sequencing, and functional analysis) in order to understand the function and importance of genes in a community and how they influence each other's activities in a collective function (NRC 2007). The general study process (shown in Fig. 1) and some metagenomic techniques have also proved useful for studying the genomic information of unculturable species. Some authors define these studies as the methodological route for the genomic characterization of microbial communities using culture-independent approaches (Chen and Pachter 2005).

Figure 1: Studies of the 16S rRNA gene follow similar steps to those in metagenomic studies, as shown in the figure modified from Cock et al. (2010), except that the cloning process includes only small-insert libraries.
These studies are important because approximately 99% of microorganisms present in a natural environment are not culturable (Amann et al. 1995, Curtis et al. 2002, Handelsman 2004, Cock et al. 2010). The percentage of unculturable organisms varies depending on the environment from which they come and how intensely they have been studied. For example, in the human microbiome, the percentage of unculturable organisms is approximately 70-80% (Nelson 2011), whereas in marine biomes it is higher than 97% (Rappé and Giovannoni 2003). Studies of marine microbial communities would benefit from the application of genomic techniques that prevent the isolation and growth of microbes while generating useful information for their taxo-nomic identification and classification.
Taxonomic assignment
When 16S rRNA is used to study a microbial community, the extraction of DNA and the amplification and subsequent sequencing of the gene or a segment do not represent technical challenges since the general protocols used are well established and highly reproducible. The taxonomic assignment and/or identification of the members, on the other hand, can be challenging not only because of the differences that can occur depending on the primers and regions selected for the study but also because of the conceptual aspects inherent to taxonomic work, the bioinformatics tools, and the availability of reference sequences.
The biological species concept is, to a certain extent, difficult to apply to microorganisms. For many years, the assignment of taxonomic categories (species and/or strain) was based on distinct biochemical and/or antigenic characteristics, and at best, on physiological features detectable by chemical or biochemical analyses. With the advent of genomic technologies, some chemical and physical properties of nucleic acids could be considered and relatively short fragment sequences or a pair of genes could subsequently be compared. New high-resolution techniques that allow massive analyses propose a species concept based on more than the similarity of a nucleotide segment or of some genes. While it is true that these new tools enhance the accuracy and contribution of large amounts of information, they also introduce greater complexity and the boundaries between species and strains are still not accurately defined. A prokaryotic species is described as "a monophyletic and genomically coherent cluster of individual organisms that show a high degree of overall similarity in many independent characteristics" (Rosselló-Mora and Amann 2001).
The first genotypic method systematically used in taxo-nomic or identification studies consisted in determining the guanine and cytosine (G + C) content. It is currently relatively easy to estimate the composition of a whole genome and it has been observed that, in nearly all cases, the difference in G + C content between strains does not exceed 1%; higher values are indicative of different species (Rosselló-Mora and Amann 2001, Meier-Kolthoff et al. 2014). Over the past 50 years, the DNA-DNA hybridization (DDH) technique proved to be a valuable tool for comparing prokaryotes and measuring the degree of similarity between two genomes. It is still used to show the degree of hybridization and similarity between probable members in those cases where one taxon has more than one strain (Tindall et al. 2010). The DDH technique is also recommended for determining closeness when strains have more than 97% 16S rRNA sequence similarity. Initially, a 16S rRNA sequence similarity equal to or greater than 97% indicated that the strains belonged to the same species (Stackebrandt and Ebers 2006, Vos 2011). A more recent study, supported by cross-validation tests, revealed that 98.65% 16S rRNA gene sequence similarity can be used as the threshold for differentiating two species (Kim et al. 2014). It has also been established that when comparing two 16S rRNA, a similarity equal to or less than 94.5%, 86.5%, 82.0%, 78.5% and 75% distinguishes genus, family, order, class, and phylum, respectively (Yarza et al. 2014).
The use of sequences of other genes has also been proposed for taxonomic assignment; however, less than 100 genes have the distribution and variability that make them suitable for use as markers (Koonin 2003). The average nucleotide identity (ANI) represents a mean of identity/ similarity values between homologous genomic regions (Konstantinidis and Tiedje 2005). ANI values of 95-96% correspond to a DDH value of 70% and they are the minimum values for two organisms to belong to the same species (Kim et al. 2014). This measure can be supported by multi-locus sequence analysis (MLSA), though it is not applicable to uncultured organisms (Schleifer 2009). Ribosomal mul-tilocus sequence typing (rMLST), a variation of MLSA, analyzes 53 genes encoding ribosomal proteins, even though some of these genes have not been detected in some bacterial genomes (Larsen et al. 2014). On the other hand, the Genome BLAST Distance Phylogeny (GBDP) strategy considers the whole genome sequence and a global comparison is made using alignment tools such as BLAT or BLAST (Basic Local Alignment Search Tool) (Chun and Rainey 2014). Another genomic approach for taxonomic assignment is the characterization of DNA fragments and their variations, which can be restriction sites, insertions and deletions, DNA sequence repeats or microsatellites, single nucleotide polymorphisms or other sequence variations (Moore et al. 2010). Amplified fragment length polymorphism (AFLP) has also proven useful for the delineation of new species (Nemec et al. 2001). In order to apply as much information as possible to obtain the most accurate characterization possible, taxonomic assignment protocols using other molecules and a polyphasic approach, including MALD-TOF mass spectrometry (Ramasamy et al. 2014), have also been proposed (Moore et al. 2010). The most commonly used techniques and the level of taxonomic resolution are shown in Figure 2.

Figure 2: Methodology for the characterization of prokaryotes and the different levels of taxonomic resolution (modified from Moore et al. 2010).
Databases and analysis strategies
Taxonomic assignments are made by comparing sequences, and the databases that store them, the search tools, and the comparison strategies used are key to achieving this goal. Several databases and programs are available. The programs have different analysis strategies and the databases they operate show some variations. In addition to the general databases, given the progress of 16S rRNA gene sequencing methods, specific databases have been established that are fundamental tools for the taxonomic classification of microorganisms (Santamaria et al. 2012, Kim et al. 2013, Selama et al. 2013, Chun and Rainey 2014).
General databases
The National Center for Biotechnology Information (NCBI) in the United States is the institution that has the highest number of sequences (more than 182 million) deposited in its GenBank database (Fig. 3). Sequences of the 16S rRNA gene are stored in two nucleotide sequence databases: the non-redundant nucleotide (nr/nt) database containing more than 30 million sequences and the 16S database, which is dedicated to 16S rRNA sequences of identified bacteria and archaea and currently contains more than 17,600 sequences (for the most part whole). While both databases can serve as reference for searching and comparing sequences using the BLAST algorithm, the nr/nt database is broader and contains the GenBank, European Bioinformatics Institute (EMBL-EBI), DNA Databank of Japan (DDBJ), and Protein Data Bank (PDB) sequence collections, as well as the NCBI Reference Sequence (RefSeq) database. It is a non-redundant database because in some cases identical sequences have been merged into one entry, while preserving the accession number, GenBank identifier, title, and taxonomy information for each sequence (http://www. ncbi.nlm.nih.gov/guide/all/#databases).
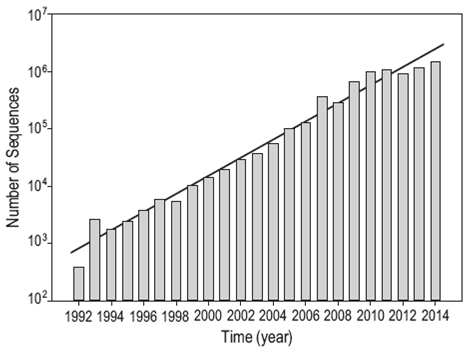
Figure 3: Exponential growth of the number of 16S rRNA sequences registered in the GenBank database managed by the National Center for Biotechnology Information (NCBI, USA).
Through the International Nucleotide Sequence Database Collaboration (INSDC), all the GenBank information can be found in the EMBL-EBI and DDBJ databases. INSDC is a long-standing initiative between DDBJ, EMBL-EPI, and NCBI for the maintenance of sequence databases and covers the spectrum of data, including raw reads, assemblies and alignments to functional annotations and contextual information relating to the samples (Karsch-Mizrachi et al. 2012).
Specific databases
While the 16S rRNA gene was originally used to identify bacteria, today it is used as a standard for the classification and identification of microorganisms and the sequences are readily available in public databases. As these sequences often lack validation, databases that collect only 16S sequences were created. Some databases, such as the Greengenes database (http://greengenes.lbl.gov/), have not been updated but can still be accessed. Below we mention the most important databases.
SILVA: High quality ribosomal RNA databases
The SILVA website (http://www.arb-silva.de) provides databases of aligned small subunit (16S/18S, SSU) and large subunit (23S/28S, LSU) rRNA sequences for the Bacteria, Archaea, and Eukarya domains. In the case of SSU, the information is found in three datasets that differ by the size and quality of the sequences: (1) SSU Parc contains more than 4.3 million sequences larger than 300 nucleotides; (2) SSU Ref contains more than 1.5 million high-quality, nearly full-length sequences (1450 nucleotides); and (3) SSU Ref NR contains more than 534,000 non-redundant sequences, which are basically the same as in SSU Ref but have <99% similarity. All sequences are associated with their GenBank accession number, taxonomy, multiple sequence alignment, type of strain, and last valid nomenclature (Yilmaz et al. 2014).
In addition to the datasets, SILVA also developed an alignment tool called SINA (SILVA Incremental Aligner), which can align hundred thousands of sequences based on a curated seed alignment. SINA uses a combination of &-mer searching and partial order alignment to maintain high alignment accuracy (Pruesse et al. 2012). After the alignment, tax-onomic classification is possible using the lowest common ancestor (LCA) method (Clemente et al. 2011), based on different taxonomies provided by SILVA (SILVA, LTP, Greengenes, RDP, EMBL).
Ribosomal Database Project
The Ribosomal Database Project (RDP) at Michigan State University provides ribosome-related data services, including online data analysis, rRNA-derived phylogenetic trees, and aligned and annotated rRNA sequences (https:// rdp.cme.msu.edu/index.jsp). This database contains more than three million sequences of both culturable and uncultur-able bacteria and archaea (Cole et al. 2005, 2014). The sequences are aligned by an alignment program, called INFERNAL (INFERence of RNA ALignment), based on stochastic context that incorporates secondary structure information (Nawrocki et al. 2009, Nawrocki and Eddy 2013). For the taxonomic identification, this database uses the RDP Classifier tool, based on a simple Bayesian algorithm (Vilo and Dong 2012).
EzTaxon
The EzTaxon database (http://www.ezbiocloud.net/eztaxon) originally included only 16S sequences of type strains of identified bacterial species (Chun et al. 2007). It was later expanded to include sequences of uncultured species, which are very frequent in environmental studies (Kim et al. 2012). This database currently contains more than 62,600 species and phylotypes. BLAST and MEGABLAST searches are followed by rigorous global pair-wise sequence alignment (Myers and Miller 1988).
Computer programs
MEGAN allows the analysis of metagenomic sequences using the BLAST comparison tool and the LCA algorithm to assign taxons and the NCBI taxonomy as reference (Huson et al. 2007).
PhymmBL was designed for the taxonomic classification of short metagenomic reads. Basically, this program combines composition-based (Phymm) and homology-based (BLAST) taxonomic predictions (Brady and Salzberg 2009).
RITA searches for an agreement between homology-based and composition-based approaches, which considerably increases the accuracy of taxonomic assignments. This program uses a naive Bayesian classifier and optimized homology detection algorithms, and is thus faster than PhymmBL and other programs (MacDonald et al. 2012).
Kraken is an accurate program for assigning taxonomic lables to metagenomic sequences based on the exact alignment of k-mers, making it a faster tool than those that use inexact alignment of sequences. It also provides greater taxo-nomic richness because it uses k-mers of 31 base pairs, which allows high sensitivity among sequences with a high degree of similarity. The sensitivity and precision achieved depend on the database used by the program to make the comparisons (Wood and Salzberg 2014).
The RDP Classifier, a naive Bayesian classifier, provides taxonomic assignments of bacteria from domain to genus. It uses eight-base subsequences (words) to achieve a balance between the sensitivity and speed of analysis or computer requirements. The position of the word is ignored and only the words found contribute to the classification. This program is useful for analyzing long sequences but does not discriminate well when using short sequences (Wang et al. 2007).
Trends
Multiple strategies exist for the use of 16S rRNA as a tool in microbial identification; however, the accurate classification of microorganisms is a complex task. The identification of organisms from a community, using either the complete sequence or a region of the 16S rRNA gene, is made easier if several of its members have previously been placed in a taxonomic arrangement. In environments such as the marine environment, where the microbial life has not yet been well documented, the task of identification is hampered by the absence of reference sequences. The advent of these novel sequencing techniques allowed many studies aiming to characterize little-known microbial communities to generate genetic information, but other limitations then arose associated with the storage, processing, analysis, and interpretation of the large amount of raw data produced. Fortunately, all these limitations have been solved with the development of computational tools and of algorithms and programs for accurate microbial identification.
Massive sequencing has produced a large amount of partial sequences but efforts to obtain a complete sequence have not yet produced the desired results (Miller et al. 2011, 2013). Many studies that use partial sequences to identify microorganisms do not find a reference sequence and the tax-onomic certainty is reduced. In some cases, the result can be an overestimation of the diversity. In an analysis of a simulated community, built by the 16S rRNA sequences of soil bacteria belonging to 20 OTUs and using fragments that included the V4/V5 and V2/V3 regions, after appropriate selection and removal of chimera, from 72 to 333 OTUs were found, depending on the experimental conditions during the sequencing process (Jeon et al. 2015). Hence, to maintain parallelism in the development of "omics" techniques and the exponential growth of 16S rRNA databases, and particularly in the study of marine bacterial communities (Giovannoni et al. 2005, Pedros-Alio 2006, Kämpfer and Glaeser 2012), complete sequences of this gene are needed in order to solve with greater precision and reproducibility the different taxonomic assignments.
References
Amann, RI, Ludwig, W, Schleifer, KH 1995. Phylogenetic identification and in situ detection of individual microbial cells without cultivation. Microbiol. Mol. Biol. Rev. 59: 143-169. [ Links ]
Amaral-Zettler L, Artigas LF, Baross J, Bharathi L, Boetius A, Chandramohan D, Herndl G, Kogure K, Neal P, Pedros-Alio C, Ramette A, Schouten S, Stal L, Thessen A, de Leeuw J, Sogin M. 2010. A global census of marine microbes. In: McIntyre A (ed.), Life in the World's Oceans: Diversity, Distribution and Abundance. Blackwell Publishing, Oxford. [ Links ]
Ameryk A, Hahnke RL, Gromisz S, Kownacka J, Zalewski M, Szymanek L, Calkiewicz J, Dunalska J, Harder J. 2014. Bacterial community structure influenced by Coscinodiscus sp. in the Vistula river plume. Oceanologia 56: 825-856. http://dx.doi.org/10.5697/oc.56-4.825 [ Links ]
Aylward FO, Eppley JM, Smith JM, Chavez FP, Scholin CA, DeLong EF. 2015. Microbial community transcriptional networks are conserved in three domains at ocean basin scales. Proc. Natl. Acad. Sci. USA 112: 5443-5448. http://dx.doi.org/10.1073/pnas.1502883112 [ Links ]
Baker GC, Smith JJ, Cowan DA. 2003. Review and re-analysis of domain-specific 16S primers. J. Microbiol. Methods 55: 541-555. http://dx.doi.org/10.1016/j.mimet.2003.08.009 [ Links ]
Barberá A, Fernádez-Guerra A, Bohannan BJM, Casamayor EO. 2012. Exploration of community traits as ecological markers in microbial metagenomes. Mol. Ecol. 21: 1909-1917. http://dx.doi.org/10.1111/j.1365-294X.2011.05383.x [ Links ]
Biddle JF, Lipp JS, Lever MA, Lloyd KG, Sorensen KB, Anderson R, Fredricks HF, Elvert M, Kelly TJ, Schrag DP, Sogin ML, Brenchley JE, Teske A, House CH, Hinrichs KU. 2006. Heterotrophic Archaea dominate sedimentary subsurface ecosystems off Peru. Proc. Natl. Acad. Sci. USA 103: 3846-3851. http://dx.doi.org/10.1073/pnas.0600035103 [ Links ]
Bižić-Ionescu M, Zeder M, Ionescu D, Orlic S, Fuchs BM, Grossart, H-P, Amann R. 2014. Comparison of bacterial communities on limnic versus coastal marine particles reveals profound differences in colonization. Environ. Microbiol. http://dx.doi.org/10.1111/1462-2920.12466. [ Links ]
Blaxter ML. 2004. The promise of a DNA taxonomy. Philos. Trans. R. Soc. Lond. (B Biol. Sci.) 359: 669-679. http://dx.doi.org/10.1098/rstb.2003.1447 [ Links ]
Boeuf D, Cottrell MT, Kirchman DL, Lebaron P, Jeanthon C. 2013. Summer community structure of aerobic anoxygenic phototrophic bacteria in the western Arctic Ocean. FEMS Microbiol. Ecol. 85: 417-432. http://dx.doi.org/10.1111/1574-6941.12130 [ Links ]
Bowen J, Weisman D, Yasuda M, Jayakumar A, Morrison H, Ward B. 2015. Marine oxygen-deficient zones harbor depauperate denitrifying communities compared to novel genetic diversity in coastal sediments. Microb. Ecol.: 1-11. http://dx.doi.org/10.1007/s00248-015-0582-y [ Links ]
Brady A, Salzberg SL. 2009. Phymm and PhymmBL: Metagenomic phylogenetic classification with interpolated Markov models. Nature Methods 6: 673-676. http://dx.doi.org/10.1038/nmeth.1358 [ Links ]
Brown MV, Ostrowski M, Grzymski JJ, Lauro FM. 2014. A trait based perspective on the biogeography of common and abundant marine bacterioplankton clades. Mar. Genomics 15: 17-28. http://dx.doi.org/10.1016/j.margen.2014.03.002 [ Links ]
Cai L, Ye L, Tong AHY, Lok S, Zhang T. 2013. Biased diversity metrics revealed by bacterial 16S pyrotags derived from different primer sets. PLOS ONE 8: e53649. http://dx.doi.org/10.1371/journal.pone.0053649 [ Links ]
Chen K, Pachter L. 2005. Bioinformatics for whole-genome shotgun sequencing of microbial communities. PLOS Comput. Biol. 1: e24. http://dx.doi.org/10.1371/journal.pcbi.0010024 [ Links ]
Chun J, Rainey FA. 2014. Integrating genomics into the taxonomy and systematics of the Bacteria and Archaea. Int. J. Syst. Evol. Microbiol. 64: 316-324. http://dx.doi.org/10.1099/ijs.0.054171-0 [ Links ]
Chun J , Lee JH, Jung Y, Kim M, Kim S, Kim BK, Lim YW. 2007. EzTaxon: A web-based tool for the identification of prokaryotes based on 16S ribosomal RNA gene sequences. Int. J. Syst. Evol. Microbiol. 57: 2259-2261. http://dx.doi.org/10.1099/ijs.0.64915-0 [ Links ]
Clemente JC, Jansson J, Valiente G. 2011. Flexible taxonomic assignment of ambiguous sequencing reads. BMC Bioinformatics 12: 8. http://dx.doi.org/10.1186/1471-2105-12-8 [ Links ]
Cock JM, Tessmar-Raible K, Boyen C, Viard F. 2010. Introduction to Marine Genomics, Advances in Marine Genomics 1. Springer, Dordrecht, 399 pp. [ Links ]
Cole JR, Chai B, Farris RJ, Wang Q, Kulam SA, McGarrell DM, Garrity GM, Tiedje JM. 2005. The Ribosomal Database Project (RDP-II): Sequences and tools for high-throughput rRNA analysis. Nucleic Acids Res. 33: D294-D296. http://dx.doi.org/10.1093/nar/gki038 [ Links ]
Cole JR, Wang Q, Fish JA, Chai B, McGarrell DM, Sun Y, Brown CT, Porras-Alfaro A, Kuske CR, Tiedje JM . 2014. Ribosomal Database Project: Data and tools for high throughput rRNA analysis. Nucleic Acids Res. 42: D633-642. http://dx.doi.org/10.1093/nar/gkt1244 [ Links ]
Crab R, Defoirdt T, Bossier P, Verstraete W. 2012. Biofloc technology in aquaculture: Beneficial effects and future challenges. Aquaculture 356-357: 351-356. http://dx.doi.org/10.1016/j.aquaculture.2012.04.046 [ Links ]
Curtis TP, Sloan WT, Scannell JW. 2002. Estimating prokaryotic diversity and its limits. Proc. Natl. Acad. Sci. USA 99: 10494-10499. http://dx.doi.org/10.1073/pnas.142680199 [ Links ]
Fuhrman JA, Cram JA, Needham DM. 2015. Marine microbial community dynamics and their ecological interpretation. Nature Rev. Microbiol. 13: 133-146. http://dx.doi.org/10.1038/nrmicro3417 [ Links ]
Gibbons SM, Caporaso JG, Pirrung M, Field D, Knight R, Gilbert JA. 2013. Evidence for a persistent microbial seed bank throughout the global ocean. Proc. Natl. Acad. Sci. USA 110: 4651-4655. http://dx.doi.org/10.1073/pnas.1217767110 [ Links ]
Giovannoni SJ, Tripp HJ, Givan S, Podar M, Vergin KL, Baptista D, Bibbs L, Eads J, Richardson TH, Noordewier M, Rappé MS, Short JM, Carrington JC, Mathur EJ. 2005. Genome streamlining in a cosmopolitan oceanic bacterium. Science 309: 1242-1245. http://dx.doi.org/10.1126/science.1114057 [ Links ]
Gobet A, Boetius A, Ramette A. 2014. Ecological coherence of diversity patterns derived from classical fingerprinting and Next Generation Sequencing techniques. Environ. Microbiol. 16: 2672-2681. http://dx.doi.org/10.1111/1462-2920.12308 [ Links ]
Hahnke RL, Probian C, Fuchs BM, Harder J. 2013. Variations in pelagic bacterial communities in the North Atlantic Ocean coincide with water bodies. Aquat. Microb. Ecol. 71: 131-140. http://dx.doi.org/10.3354/ame01668 [ Links ]
Handelsman J. 2004. Metagenomics: Application of genomics to uncultured microorganisms. Microbiol. Mol. Biol. Rev. 68: 669-685. http://dx.doi.org/10.1128/mmbr.68.4.669-685.2004 [ Links ]
Hatosy SM, Martiny JBH, Sachdeva R, Steele J, Fuhrman JA, Martiny AC. 2013. Beta diversity of marine bacteria depends on temporal scale. Ecology 94: 1898-1904. http://dx.doi.org/10.1890/12-2125.1 [ Links ]
Havelsrud OE, Haverkamp TH, Kristensen T, Jakobsen KS, Rike AG. 2011. A metagenomic study of methanotrophic microorganisms in Coal Oil Point seep sediments. BMC Microbiology 11: 221. http://dx.doi.org/10.1186/1471-2180-11-221 [ Links ]
Hebert PDN, Cywinska A, Ball SL, deWaard JR. 2003. Biological identifications through DNA barcodes. Proc. R. Soc. Lond. (B) 270: 313-321. http://dx.doi.org/10.1098/rspb.2002.2218 [ Links ]
Hellweger FL, van Sebille E, Fredrick ND. 2014. Biogeographic patterns in ocean microbes emerge in a neutral agent-based model. Science 345: 1346-1349. http://dx.doi.org/10.1126/science.1254421 [ Links ]
Hong S, Bunge J, Leslin C, Jeon S, Epstein SS. 2009. Polymerase chain reaction primers miss half of rRNA microbial diversity. ISME J. 3: 1365-1373. http://dx.doi.org/10.1038/ismej.2009.89 [ Links ]
Huse SM, Dethlefsen L, Huber JA, Welch DM, Relman DA, Sogin ML. 2008. Exploring microbial diversity and taxonomy using SSU rRNA hypervariable Tag sequencing. PLOS Genet. 4: e1000255. http://dx.doi.org/10.1371/journal.pgen.1000255 [ Links ]
Huson DH, Auch AF, Qi J, Schuster SC. 2007. MEGAN analysis of metagenomic data. Genome Res. 17: 377-386. http://dx.doi.org/10.1101/gr.5969107 [ Links ]
Huws SA, Edwards JE, Kim EJ, Scollan ND. 2007. Specificity and sensitivity of eubacterial primers utilized for molecular profiling of bacteria within complex microbial ecosystems. J. Microbiol. Meth. 70: 565-569. http://dx.doi.org/10.1016/j.mimet.2007.06.013 [ Links ]
Jeon YS, Park SC, Lim J, Chun J, Kim BS. 2015. Improved pipeline for reducing erroneous identification by 16S rRNA sequences using the Illumina MiSeq platform. J. Microbiol. 53: 60-69. http://dx.doi.org/10.1007/s12275-015-4601-y [ Links ]
Kämpfer P, Glaeser SP. 2012. Prokaryotic taxonomy in the sequencing era: The polyphasic approach revisited. Environ. Microbiol. 14: 291-317. http://dx.doi.org/10.1111/j.1462-2920.2011.02615.x [ Links ]
Karl DM. 2007. Microbial oceanography: Paradigms, processes and promise. Nature Rev. Microbiol. 5: 759-769. http://dx.doi.org/10.1038/nrmicro1749 [ Links ]
Karl DM, Church MJ. 2014. Microbial oceanography and the Hawaii Ocean time-series programme. Nature Rev. Microbiol. 12: 699-713. http://dx.doi.org/10.1038/nrmicro3333 [ Links ]
Karsch-Mizrachi I, Nakamura Y, Cochrane G. 2012. The International Nucleotide Sequence Database Collaboration. Nucleic Acids Res. 40: D33-D37. http://dx.doi.org/10.1093/nar/gkr1006 [ Links ]
Karsenti E, Acinas SG, Bork P, Bowler C, De Vargas C, Raes J, Sullivan M, Arendt D, Benzoni F, Claverie JM, Follows M, Gorsky G, Hingamp P, Iudicone D, Jaillon O, Kandels-Lewis S, Krzic U, Not F, Ogata H, Pesant S, Reynaud EG, Sardet C, Sieracki ME, Speich S, Velayoudon D, Weissenbach J, Wincker P, the Tara Oceans Consortium. 2011. A holistic approach to marine eco-systems biology. PLOS Biol. 9: e1001177.http://dx.doi.org/10.1371/journal.pbio.1001177 [ Links ]
Kato S, Ikehata K, Shibuya T, Urabe T, Ohkuma M, Yamagishi A. 2015. Potential for biogeochemical cycling of sulfur, iron and carbon within massive sulfide deposits below the seafloor. Environ. Microbiol. 17: 1817-1835. http://dx.doi.org/10.1111/1462-2920.12648 [ Links ]
Kim M, Lee KH, Yoon SW, Kim BS , Chun J , Yi H. 2013. Analytical tools and databases for metagenomics in the next-generation sequencing era. Genomics Inform. 11: 102-113. http://dx.doi.org/10.5808/GI.2013.1L3.102 [ Links ]
Kim M, Oh HS, Park SC, Chun J. 2014. Towards a taxonomic coherence between average nucleotide identity and 16S rRNA gene sequence similarity for species demarcation of prokaryotes. Int. J. Syst. Evol. Microbiol. 64: 346-351. http://dx.doi.org/10.1099/ijs.0.059774-0 [ Links ]
Kim OS, Cho YJ, Lee K, Yoon SH, Kim M, Na H, Park SC, Jeon YS, Lee JH, Yi H, Won S, Chun J. 2012. Introducing EzTaxon-e: A prokaryotic 16S rRNA gene sequence database with phylotypes that represent uncultured species. Int. J. Syst. Evol. Microbiol. 62: 716-721. http://dx.doi.org/10.1099/ijs.0.038075-0 [ Links ]
Klindworth A, Mann AJ, Huang S, Wichels A, Quast C, Waldmann J, Teeling H, Glõckner FO. 2014. Diversity and activity of marine bacterioplankton during a diatom bloom in the North Sea assessed by total RNA and pyrotag sequencing. Mar. Genomics 18B: 185-192. http://dx.doi.org/10.1016/j.margen.2014.08.007 [ Links ]
Konstantinidis KT, Tiedje JM. 2005. Genomic insights that advance the species definition for prokaryotes. Proc. Natl. Acad. Sci. USA 102: 2567-2572. http://dx.doi.org/10.1073/pnas.0409727102 [ Links ]
Koonin EV. 2003. Comparative genomics, minimal gene-sets and the last universal common ancestor. Nature Rev. Microbiol. 1: 127-136. http://dx.doi.org/10.1038/nrmicro751 [ Links ]
Kress WJ, Erickson DL. 2012. DNA Barcodes: Methods and Protocols. Methods in Molecular Biology 858. Humana Press/Springer, New York, 470 pp. [ Links ]
Larsen MV, Cosentino S, Lukjancenko O, Saputra D, Rasmussen S, Hasman H, Sicheritz-Pontén T, Aarestrup FM, Ussery DW, Lund O. 2014. Benchmarking of methods for genomic taxonomy. J. Clin. Microbiol. 52: 1529-1539. http://dx.doi.org/10.1128/jcm.02981-13 [ Links ]
Laverock B, Tait K, Gilbert JA, Osborn AM, Widdicombe S. 2014. Impacts of bioturbation on temporal variation in bacterial and archaeal nitrogen-cycling gene abundance in coastal sediments. Environ. Microbiol. Rep. 6: 113-121. http://dx.doi.org/10.1111/1758-2229.12115 [ Links ]
Lebonah DE, Dileep A, Chandrasekhar K, Sreevani S, Sreedevi B y Kumari PJ. 2014. DNA barcoding on bacteria: A review. Adv. Biol. 2014: 541787. http://dx.doi.org/10.1155/2014/541787 [ Links ]
Ley RE, Peterson DA, Gordon JI. 2006. Ecological and evolutionary forces shaping microbial diversity in the human intestine. Cell 124: 837-848. http://dx.doi.org/10.1016/j.cell.2006.02.017 [ Links ]
Lipsewers YA, Bale NJ, Hopmans EC, Schouten S, Sinninghe Damste JS, Villanueva L. 2014. Seasonality and depth distribution of the abundance and activity of ammonia oxidizing microorganisms in marine coastal sediments (North Sea). Front. Microbiol. 5: 472. http://dx.doi.org/10.3389/fmicb.2014.00472 [ Links ]
Liu Z, Lozupone C, Hamady M, Bushman FD, Knight R. 2007. Short pyrosequencing reads suffice for accurate microbial community analysis. Nucleic Acids Res. 35: e120. http://dx.doi.org/10.1093/nar/gkm541 [ Links ]
Liu Z, DeSantis TZ, Andersen GL, Knight R. 2008. Accurate taxonomy assignments from 16S rRNA sequences produced by highly parallel pyrosequencers. Nucleic Acids Res. 36: e120-e120. http://dx.doi.org/10.1093/nar/gkn491 [ Links ]
Logares R, Sunagawa S, Salazar G, Cornejo-Castillo FM, Ferrera I, Sarmento H, Hingamp P, Ogata H, de Vargas C, Lima-Mendez G, Raes J, Poulain J, Jaillon O, Wincker P, Kandels-Lewis S, Karsenti E, Bork P, Acinas SG. 2014. Metagenomic 16S rDNA Illumina tags are a powerful alternative to amplicon sequencing to explore diversity and structure of microbial communities. Environ. Microbiol. 16: 2659-2671. http://dx.doi.org/10.1111/1462-2920.12250 [ Links ]
MacDonald NJ, Parks DH, Beiko RG. 2012. Rapid identification of high-confidence taxonomic assignments for metagenomic data. Nucleic Acids Res. 40: e111. http://dx.doi.org/10.1093/nar/gks335 [ Links ]
Meier-Kolthoff JP, Klenk HP, Gõker M. 2014. Taxonomic use of DNA G+C content and DNA-DNA hybridization in the genomic age. Int. J. Syst. Evol. Microbiol. 64: 352-356. [ Links ]
Miller C, Baker B, Thomas B, Singer S, Banfield J. 2011. EMIRGE: Reconstruction of full-length ribosomal genes from microbial community short read sequencing data. Genome Biol. 12: R44. http://dx.doi.org/10.1186/gb-2011-12-5-r44 [ Links ]
Miller CS, Handley KM, Wrighton KC, Frischkorn KR, Thomas BC, Banfield JF. 2013. Short-read assembly of full-length 16S amplicons reveals bacterial diversity in subsurface sediments. PLOS ONE 8: e56018. http://dx.doi.org/10.1371/journal.pone.0056018 [ Links ]
Mohit V, Archambault P, Toupoint N, Lovejoy C. 2014. Phylogenetic differences in attached and free-living bacterial communities in a temperate coastal lagoon during summer, revealed via high-throughput 16S rRNA gene sequencing. Appl. Environ. Microbiol. 80: 2071-2083. http://dx.doi.org/10.1128/aem.02916-13 [ Links ]
Moore ER, Mihaylova SA, Vandamme P, Krichevsky MI, Dijkshoorn L. 2010. Microbial systematics and taxonomy: Relevance for a microbial commons. Res. Microbiol. 161: 430-438. http://dx.doi.org/10.1016/j.resmic.2010.05.007 [ Links ]
Mueller RS, Bryson S, Kieft B, Li Z, Pett-Ridge J, Chavez F, Hettich RL, Pan C, Mayali X. 2015. Metagenome sequencing of a coastal marine microbial community from Monterey Bay, California. Genome Announcements 3: e00341-00315. http://dx.doi.org/10.1128/genomeA.00341-15 [ Links ]
Myers EW, Miller W. 1988. Optimal alignments in linear space. Comput. Appl. Biosci. 4: 11-17. http://dx.doi.org/10.1093/bioinformatics/4.1.11 [ Links ]
[NRC] National Research Council (US). 2007. The New Science of Metagenomics: Revealing the secrets of our microbial planet. National Academies Press, Washington, DC, 158 pp. [ Links ]
Nawrocki EP, Eddy SR. 2013. Infernal 1.1: 100-fold faster RNA homology searches. Bioinformatics 29: 2933-2935. http://dx.doi.org/10.1093/bioinformatics/btt509 [ Links ]
Nawrocki EP, Kolbe DL, Eddy SR. 2009. Infernal 1.0: Inference of RNA alignments. Bioinformatics 25: 1335-1337. http://dx.doi.org/10.1093/bioinformatics/btp157 [ Links ]
Nelson KE. 2011. Metagenomics of the Human Body. Springer Science + Business Media, LLC, New York, 351 pp. [ Links ]
Nemec A, De Baere T, Tjernberg I, Vaneechoutte M, van der Reijden TJ, Dijkshoorn L. 2001. Acinetobacter ursingii sp. nov. and Acinetobacter schindleri sp. nov., isolated from human clinical specimens. Int. J. Syst. Evol. Microbiol. 51: 1891-1899. http://dx.doi.org/10.1099/00207713-51-5-1891 [ Links ]
Olsen LA, Choffnes ER, Mack A. 2012. The Social Biology of Microbial Communities. Workshop Summary. National Academies Press, Washington, D.C., xxviii, 603 pp. [ Links ]
Pace NR, Stahl DA, Lane DJ, Olsen GJ. 1986. The analysis of natural microbial populations by ribosomal RNA sequences. In: Marshall KC (ed.), Advances in Microbial Ecology. Springer, US, pp. 1-55. [ Links ]
Pedros-Alio C. 2006. Genomics and marine microbial ecology. Int. Microbiol. 9: 191-197. [ Links ]
Prabavathi R, Mathivanan V. 2012. Isolation of genomic DNA and construction of metagenomic library from marine soil sediments. Int. J. Pharma Bio Sci. (IJPBS) 3: B1-B9. [ Links ]
Pruesse E, Peplies J, Glõckner FO. 2012. SINA: Accurate high-throughput multiple sequence alignment of ribosomal RNA genes. Bioinformatics 28: 1823-1829. http://dx.doi.org/10.1093/bioinformatics/bts252 [ Links ]
Ramasamy D, Mishra AK, Lagier J-C, Padhmanabhan R, Rossi M, Sentausa E, Raoult D, Fournier P-E. 2014. A polyphasic strategy incorporating genomic data for the taxonomic description of novel bacterial species. Int. J. Syst. Evol. Microbiol. 64: Microbiol. Infect. 15: 60-62. http://dx.doi.org/10.1111/j.1469-0691.2008.02692.x [ Links ]
Rappé MS, Giovannoni SJ. 2003. The Uncultured Microbial Majority. Annu. Rev. Microbiol. 57: 369-394. http://dx.doi.org/10.1146/annurev.micro.57.030502.090759 [ Links ]
Rodicio MDR, Mendoza MDC. 2004. Identificación bacteriana mediante secuenciación del ARNr 16S: Fundamento, metodología y aplicaciones en microbiología clínica. Enfermedades Infecciosas y Microbiología Clínica 22: 238-245. [ Links ]
Rosselló-Mora R, Amann R. 2001. The species concept for prokaryotes. FEMS Microbiol. Rev. 25: 39-67. http://dx.doi.org/10.1111/j.1574-6976.2001.tb00571.x [ Links ]
Roussel EG, Konn C, Charlou JL, Donval JP, Fouquet Y, Querellou J, Prieur D, Cambon Bonavita MA. 2011. Comparison of microbial communities associated with three Atlantic ultramafic hydrothermal systems. FEMS Microbiol. Ecol. 77: 647-665. http://dx.doi.org/10.1111/j.1574-6941.2011.01161.x [ Links ]
Rusch DB, Halpern AL, Sutton G, Heidelberg KB, Williamson S, Yooseph S, Wu D, Eisen JA, Hoffman JM, Remington K, Beeson K, Tran B, Smith H, Baden-Tillson H, Stewart C, Thorpe J, Freeman J, Andrews-Pfannkoch C, Venter JE, Li K, Kravitz S, Heidelberg JF, Utterback T, Rogers Y-H, Falcón LI, Souza V, Bonilla-Rosso G, Eguiarte LE, Karl DM, Sathyendranath S, Platt T, Bermingham E, Gallardo V, Tamayo-Castillo G, Ferrari MR, Strausberg RL, Nealson K, Friedman R, Frazier M, Venter JC. 2007. The Sorcerer II Global Ocean Sampling Expedition: Northwest Atlantic through Eastern Tropical Pacific. PLOS Biol 5: e77. http://dx.doi.org/10.1371/journal.pbio.0050077 [ Links ]
Santamaria M, Fosso B, Consiglio A, De Caro G, Grillo G, Licciulli F, Liuni S, Marzano M, Alonso-Alemany D, Valiente G, Pesole G. 2012. Reference databases for taxonomic assignment in metagenomics. Brief. Bioinformatics 13: 682-695. http://dx.doi.org/10.1093/bib/bbs036 [ Links ]
Satinsky BM, Crump BC, Smith CB, Sharma S, Zielinski BL, Doherty M, Meng J, Sun S, Medeiros PM, Paul JH, Coles VJ, Yager PL, Moran MA. 2014. Microspatial gene expression patterns in the Amazon River Plume. Proc. Natl. Acad. Sci. USA 111: 11085-11090. http://dx.doi.org/10.1073/pnas.1402782111 [ Links ]
Savolainen V, Cowan RS, Vogler AP, Roderick GK, Lane R. 2005. Towards writing the encyclopaedia of life: An introduction to DNA barcoding. Philos. Trans. R. Soc. Lond. (B Biol. Sci.) 360: 1805-1011. http://dx.doi.org/10.1098/rstb.2005.1730 [ Links ]
Schleifer KH. 2009. Classification of Bacteria and Archaea: Past, present and future. Syst. Appl. Microbiol. 32: 533-542. http://dx.doi.org/10.1016/j.syapm.2009.09.002 [ Links ]
Selama O, James P, Nateche F, Wellington EMH, Hacene H. 2013. The world bacterial biogeography and biodiversity through databases: A case study of NCBI nucleotide database and GBIF database. BioMed Research International 2013, 11 pp. http://dx.doi.org/10.1155/2013/240175 [ Links ]
Shao S, Luan X, Dang H, Zhou H, Zhao Y, Liu H, Zhang Y, Dai L, Ye Y, Klotz MG. 2014. Deep-sea methane seep sediments in the Okhotsk Sea sustain diverse and abundant anammox bacteria. FEMS Microbiol. Ecol. 87: 503-516. http://dx.doi.org/10.1111/1574-6941.12241 [ Links ]
Simon HM, Smith MW, Herfort L. 2014. Metagenomic insights into particles and their associated microbiota in a coastal margin ecosystem. Front. Microbiol. 5: 466. http://dx.doi.org/10.3389/fmicb.2014.00466 [ Links ]
Sjöstedt J, Martiny JBH, Munk P, Riemann L. 2014. Abundance of broad bacterial taxa in the Sargasso Sea explained by environmental conditions but not water mass. Appl. Environ. Microbiol. 80: 2786-2795. http://dx.doi.org/10.1128/aem.00099-14 [ Links ]
Smith MW, Allen LZ, Allen AE, Herfort L, Simon HM. 2013. Contrasting genomic properties of free-living and particle-attached microbial assemblages within a coastal ecosystem. Front. Microbiol. 4: 120. http://dx.doi.org/10.3389/fmicb.2013.00120 [ Links ]
Stackebrandt E, Ebers J. 2006. Taxonomic parameters revisited: Tarnished gold standards. Microbiol. Today 33: 152-155. [ Links ]
Sun DL, Jiang X, Wu QL, Zhou NY. 2013. Intragenomic heterogeneity of 16S rRNA genes causes overestimation of prokaryotic diversity. Appl. Environ. Microbiol. 79: 5962-5969. http://dx.doi.org/10.1128/AEM.01282-13 [ Links ]
Sunagawa S, Coelho LP, Chaffron S, Kultima JR, Labadie K, Salazar G, Djahanschiri B, Zeller G, Mende DR, Alberti A, Cornejo-Castillo FM, Costea PI, Cruaud C, d'Ovidio F, Engelen S, Ferrera I, Gasol JM, Guidi L, Hildebrand F, Kokoszka F, Lepoivre C, Lima-Mendez G, Poulain J, Poulos BT, Royo-Llonch M, Sarmento H, Vieira-Silva S, Dimier C, Picheral M, Searson S, Kandels-Lewis S, coordinators TO, Bowler C, de Vargas C, Gorsky G, Grimsley N, Hingamp P, Iudicone D, Jaillon O, Not F, Ogata H, Pesant S, Speich S, Stemmann L, Sullivan MB, Weissenbach J, Wincker P, Karsenti E, Raes J, Acinas SG, Bork P. 2015. Structure and function of the global ocean microbiome. Science 348. http://dx.doi.org/10.1126/science.1261359 [ Links ]
Tanabe AS, Toju H. 2013. Two new computational methods for universal DNA barcoding: A benchmark using barcode sequences of bacteria, archaea, animals, fungi, and land plants. PLOS ONE 8: e76910. http://dx.doi.org/10.1371/journal.pone.0076910 [ Links ]
Tang K, Liu K, Jiao N, Zhang Y, Chen CT. 2013. Functional metagenomic investigations of microbial communities in a shallow-sea hydrothermal system. PLOS ONE 8: e72958. http://dx.doi.org/10.1371/journal.pone.0072958 [ Links ]
Tindall BJ, Rosselló-Mora R, Busse HJ, Ludwig W, Kampfer P. 2010. Notes on the characterization of prokaryote strains for taxonomic purposes. Int. J. Syst. Evol. Microbiol. 60: 249-266. http://dx.doi.org/10.1099/ijs.0.016949-0 [ Links ]
Tringe SG, Hugenholtz P. 2008. A renaissance for the pioneering 16S rRNA gene. Curr. Opin. Microbiol. 11: 442-446. http://dx.doi.org/10.1016/j.mib.2008.09.011 [ Links ]
Venter JC, Remington K, Heidelberg JF, Halpern AL, Rusch D, Eisen JA, Wu D, Paulsen I, Nelson KE, Nelson W, Fouts DE, Levy S, Knap AH, Lomas MW, Nealson K, White O, Peterson J, Hoffman J, Parsons R, Baden-Tillson H, Pfannkoch C, Rogers Y-H, Smith HO. 2004. Environmental Genome Shotgun Sequencing of the Sargasso Sea. Science 304: 66-74. http://dx.doi.org/10.1126/science.1093857 [ Links ]
Vigneron A, Cruaud P, Roussel EG , Pignet P, Caprais J-C, Callac N, Ciobanu M-C, Godfroy A, Cragg BA, Parkes JR, Van Nostrand JD, He Z, Zhou J, Toffin L. 2014. Phylogenetic and functional diversity of microbial communities associated with subsurface sediments of the Sonora Margin, Guaymas Basin. PLOS ONE 9:e104427. http://dx.doi.org/10.1371/journal.pone.0104427 [ Links ]
Vilo C, Dong Q. 2012. Evaluation of the RDP classifier accuracy using 16S rRNA gene variable regions. Metagenomics 1: 1-5. http://dx.doi.org/10.4303/mg/235551 [ Links ]
Vos M. 2011. A species concept for bacteria based on adaptive divergence. Trends Microbiol. 19: 1-7. http://dx.doi.org/10.1016/j.tim.2010.10.003 [ Links ]
Wang Q, Garrity GM, Tiedje JM, Cole JR. 2007. Naive Bayesian classifier for rapid assignment of rRNA sequences into the new bacterial taxonomy. Appl. Environ. Microbiol. 73: 5261-5267. http://dx.doi.org/10.1128/aem.00062-07 [ Links ]
Wang Y, Qian PY. 2009. Conservative fragments in bacterial 16S rRNA genes and primer design for 16S ribosomal DNA amplicons in metagenomic studies. PLOS ONE 4: e7401. http://dx.doi.org/10.1371/journal.pone.0007401 [ Links ]
Wang Y, Sheng HF, He Y, Wu JY, Jiang YX, Tam NF, Zhou HW. 2012. Comparison of the levels of bacterial diversity in freshwater, intertidal wetland, and marine sediments by using millions of illumina tags. Appl. Environ. Microbiol. 78: 8264-8271. http://dx.doi.org/10.1128/aem.01821-12 [ Links ]
Wood D, Salzberg S. 2014. Kraken: Ultrafast metagenomic sequence classification using exact alignments. Genome Biol. 15: R46. http://dx.doi.org/10.1186/gb-2014-15-3-r46 [ Links ]
Yarza P, Richter M, Peplies J, Euzeby J, Amann R, Schleifer KH, Ludwig W, Glockner FO, Rossello-Mora R. 2008. The All-Species Living Tree project: A 16S rRNA-based phylogenetic tree of all sequenced type strains. Syst. Appl. Microbiol. 31: 241-250. http://dx.doi.org/10.1016/j.syapm.2008.07.001 [ Links ]
Yarza P, Ludwig W, Euzeby J, Amann R, Schleifer KH, Glockner FO, Rossello-Mora R. 2010. Update of the All-Species Living Tree Project based on 16S and 23S rRNA sequence analyses. Syst. Appl. Microbiol. 33: 291-299. http://dx.doi.org/10.1016/j.syapm.2010.08.001 [ Links ]
Yarza P, Yilmaz P, Pruesse E, Glockner FO, Ludwig W, Schleifer KH, Whitman WB, Euzeby J, Amann R, Rossello-Mora R. 2014. Uniting the classification of cultured and uncultured bacteria and archaea using 16S rRNA gene sequences. Nat. Rev. Microbiol. 12: 635-645. http://dx.doi.org/10.1038/nrmicro3330 [ Links ]
Yilmaz P, Parfrey LW, Yarza P, Gerken J, Pruesse E, Quast C, Schweer T, Peplies J, Ludwig W, Glõckner FO. 2014. The SILVA and "All-species Living Tree Project (LTP)" taxonomic frameworks. Nucleic Acids Res. 42: D643-D648. http://dx.doi.org/10.1093/nar/gkt1209 [ Links ]
Zinger L, Gobet A, Pommier T. 2012. Two decades of describing the unseen majority of aquatic microbial diversity. Mol. Ecol. 21: 1878-1896. http://dx.doi.org/10.1111/j.1365-294X.2011.05362.x [ Links ]
Received: November 2014; Accepted: September 2015