Introduction
Carbon (C) on the planet (43 515 ± 10 000 Pg) is found mainly in geological reservoirs (identified as carbon, gas and oil [5 000 to 10 000 Pg]), oceans (87 %, with a predominance of C-inorganic), atmosphere (2 %, mainly as carbon dioxide [CO2]) and land (which includes soil and plant biomass [11 %]). Soil organic carbon (SOC) is estimated to be three times higher (1 500 Pg) than that concentrated in aboveground vegetation (450 Pg) (García-Oliva et al., 2006; Venter et al., 2021; Zhuo-Dong et al., 2021).
It has been extensively reported that SOC is determined by the quantity and vertical distribution of vegetation, intrinsic soil properties, and climate. However, the variability of SOC is influenced by anthropogenic interference, commonly carried out by soil manipulation practices (tillage, fertilization), vegetation modification (clearing, thinning, etc.) and livestock pressure, among others (Jobbagy & Jackson, 2000; García-Oliva et al., 2006; Muñoz et al., 2015; Batjes, 2016; Venter et al., 2021; Zhuo-Dong et al., 2021).
Venter et al. (2021) mention that since the beginning of livestock and agriculture (12 000 years ago), land use changes and overgrazing have caused a loss of 116 Pg of C from the topsoil up to 2 m depth, where the main ecosystems affected are grasslands, savannas and croplands (22, 22 and 21 Pg, respectively). Even when in some areas there is an increase in SOC by appropriate interventions, such as the incorporation of green manures and restrictions on vegetation removal (Parras-Alcántara et al., 2015; Mahajan et al., 2021), net balance to date is negative, because overall losses are estimated at an average of 26 % (Venter et al., 2021).
Because of the importance of SOC in soil functions and its interaction with the plant-atmosphere system, it is now considered as a universal indicator of soil safety and health (McBratney et al., 2014; Mattina et al., 2018; Food and Agriculture Organization of the United Nations [FAO], 2017a). In contrast, SOC loss is an indicator of soil degradation (Loayza et al., 2020; Mahajan et al., 2021), which is consistent with the behavior of moisture, and soil and air temperature (Gómez-Tagle et al., 2003; López-Santos et al., 2008). López-Santos et al. (2008) found that in soils poor in organic matter (< 3 %) and without vegetation protection, the behavior of energy in the topsoil (8 cm depth) is unpredictable, which is manifested in the increase in sensible heat.
In the Target 15.3 of the Sustainable Development Goals (SDGs) (FAO, 2017b), the SOC is considered as an indicator of biodiversity and resilience to climate change; this under the premise of promoting and achieving Land Degradation Neutrality (LDN), which is one of the three indicators considered as “trends in carbon stocks” (Orr et al., 2017; Laban et al., 2018). However, as mentioned above, due to multiple biotic and abiotic factors, including soil type, land use, and climatic conditions (Galicia et al., 2016), SOC stocks are not static, because they are subject to constant cycling among various sinks, in soil, vegetation, ocean, and atmosphere.
From the local, to the global scale, it is complicated to quantify SOC stocks. Therefore, FAO (2017c) compiled statistical methods based on Geographic Information Systems (GIS) to generate spatially distributed digital SOC maps (SOCDM), namely, to assess SOC considering uncertainty. This effort was aimed to support developing countries, such as those located in Latin America, because in several of them a scarcity or, in extreme cases, absence of soil data was detected (Guevara et al., 2018).
Guevara et al. (2018), as part of integration efforts of the SOC world map (http://54.229.242.119/GSOCmap/), elaborated the SOCDM of Latin America with a spatial resolution of 5 x 5 km. These authors used 11 268 estimates (n) from 19 countries, out of the 46 that integrate the region. The largest contribution of soil data (88.1 %) was from Brazil (5 616 n) and Mexico (4 321 n); other countries such as Argentina (231 n), Colombia (166 n) and Venezuela (1.45 n) had a joint contribution of less than 5 %. For this purpose, they used the SCORPAN model previously described by McBratney et al. (2003). Guevara et al. (2018) mention that from that model they combined quantitative and qualitative empirical descriptions to establish the relationship between soils and spatially distributed predictors: Sc = ꬵ(S,C,O,R,P,A,N) and Sa = f(S,C,O,R,P,A,N); where Sc corresponds to soil classes and Sa can be any soil attribute or attributes (such as SOC), which will depend on the characteristics of soil (S), climate (C), organisms (O), relief (R), parent material (P), time (A) and physical space (N).
However, in the report by Guevara et al. (2018), SOC estimates for Latin America and the Caribbean still present uncertainties reflected in standard deviations above the mean values (Brazil = 3.23 ± 9.18 kg de SOC·m-2). Mexico reports an average SOC storage of 2.56 ± 1.49 kg·m-2 (in a range from 0 to 35.55 kg·m-2), which represents a national average of about 7.68 t·ha-1 of SOC considering a depth of 30 cm. Undoubtedly, these results are a breakthrough, so regional studies could reinforce the global effort from the local (Segura-Castruita et al., 2005), especially for arid and semi-arid regions where C sinks have been underestimated (Montaño et al., 2016).
Therefore, the objectives of the present study were to estimate the magnitude of SOC stocks in the Medio Aguanaval River sub-basin (ScRMA) and to analyze the sensitivity of four interpolation methods to minimize the digital mapping error for the ScRMA.
Materials and methods
Description of the study unit
The ScRMA was considered as the study unit, located in the Mesa del Centro province and in the sub-provinces Sierra and Llanuras del Norte, and Sierras and Lomeríos de Aldama and Río Grande, in an altitudinal range from 1 400 to 2 600 m a. s. l. The ScRMA is part of hydrological region 36, with an area of 8 348 km2 and located in the east of the state of Durango, northwest of the state of Zacatecas and southeast of the state of Coahuila (24.632° N and 103.260° W) (Comisión Nacional para el Conocimiento y Uso de la Biodiversidad [CONABIO], 1998) (Figure 1).
The ScRMA includes part of the municipalities of Cuencamé, General Simón Bolívar, Santa Clara and San Juan de Guadalupe in Durango; Juan Aldama and Miguel Auza in Zacatecas, and Torreón and Viesca in Coahuila. The climate in this area is arid, with five subtypes: very arid semi-warm (BWhw), arid semi-warm (BSohw), semiarid temperate (BS1kw), arid temperate (BSokw) and semiarid semi-warm (BS1hw) (García, 1998). There is dominance of scrubland (41 %) and rainfed agriculture, which is practiced in 20 % of the territory (Instituto Nacional de Estadística y Geografía [INEGI], 2016). Precipitation and annual temperature in this area range from 125 to 600 mm and from 16 to 22° C, respectively (Cuervo-Robayo et al., 2014). The ScRMA territory includes 12 main soil groups (MSG) and 39 subgroups, where Phaeozem, with loam or medium texture, stands out in more than 80% of the area (INEGI, 2014a). The presence of topoforms such as mountain ranges, rolling hills, plateaus, plains, valleys and relieves is observed (Lugo-Hubp & Aceves-Quezada, 1992).
Stages of the research
Once the ScRMA was defined, delimited and characterized, the study considered five stages: 1) search, download and analysis of soil data, 2) data processing, 3) selection of verification sites, 4) laboratory analysis and 5) processing of data from verification sites.
Search and download of soil data
Soil data were collected from the national set consisting of 4 418 soil profiles (composed of 14 349 horizons or soil layers) classified by the World Reference Base for Soil Resources (WRB) and adapted by INEGI (2014b) as Series II. This vector set at a scale of 1:250 000 corresponds to historical data for more than 30 years (1970 to 2005), updated to 2010 (https://www.inegi.org.mx/temas/edafologia/#Descargas). From the national set, 40 profiles (0.9 %) were extracted and analyzed in terms of density (n), horizontal (x, y) and vertical (z) distribution. To extract the representative information of the sub-basin, the limit defined by the ScRMA watershed, which is from 0 to 30 cm depth, was considered.
Data processing
The SOC value of the ScRMA was determined based on the procedure described by Bautista et al. (2016), using the Soil & Environment® (S&E) program (Skiu: https://www.actswithscience.com), which allows the calculation of C stocks, as well as related environmental functions (such as soil quality and fertility). This program includes a template, where the information of the selected soil profiles was downloaded. The profile data represented the period from April 1992 to November 2005. The vector file of the profiles does not have data for bulk density (BD), so it was obtained by means of a hydraulic property estimator. For this purpose, the percentage values of clay and sand for each horizon of the profile were entered. Organic matter (OM) was calculated according to the Official Mexican Standards NOM-021-RECNAT-2000 (Secretaría de Medio Ambiente y Recursos Naturales [SEMARNAT], 2002). Soil properties used in the S&E program to calculate CO were volume of thick fragments (%), horizon thickness (dm), bulk density (g·cm-3) and SOC content (%). Equation 1 was used to estimate SOC (Gallegos-Tavera et al., 2014).
where SOC is the organic carbon of the complete soil profile (t·ha-1), BDi is the bulk density of horizon i (g·cm-3), THi is the thickness of horizon i (dm), CRi is the volume of thick fragments of horizon i (%) and C i is the percentage of organic carbon of horizon i (%).
The properties analyzed in the laboratory that are indispensable for working with S&E are C, CIC, Ca, Mg, Na, K and pH (Gallegos & Bautista, 2015). SOC values were obtained, when integrating the template with the necessary data per profile into the program. These values were ordered and, together with the general information of each profile, an Excel database was created. Considering the number of soil profiles according to the surface of the ScRMA, an interpolation of SOC data was carried out with the four geostatistics available in the ArcGIS 10.1 program (IDW, Kriging, Natural Neighbor and Spline), and it was analyzed which of them generated the best interpolation based on their statistics. Finally, a map of the SOC distribution in the ScRMA was created.
Selection of verification sites
By using different thematic layers in ArcGIS 10.1 (such as land use, vegetation, soil types, climates and municipalities), in addition to accessibility, a greater representativeness was considered for the distribution of the SOC estimation verification sites. A total of 4 kg of soil were collected at each site, and unaltered samples were taken using steel cylinders to determine bulk density.
Laboratory analysis
The variables analyzed in the soil from the verification sites were pH (potentiometer), BD (kerosene), texture (Bouyoucos), electrical conductivity (EC, conductivity meter), cation exchange capacity (CEC, titration with NaCl) and organic matter (OM, Walkley and Black). The analyses were carried out in accordance with NOM-021-RECNAT-2000 (SEMARNAT, 2002).
Processing of data from verification sites
SOC was estimated from soil OM, determined by the method proposed by Walkley and Black (1934). The Van Benmelen factor of 1.724 was used for the estimation, which results from the assumption that OM contains 58 % carbon (1/0.58 = 1.724). Subsequently, the estimate of total organic carbon (TOC) was determined from the following equation:
Finally, the template was filled with the data from the verification sites, as explained in the data processing stage, to obtain the SOC value for each site. The database from the verification sites was built to create a thematic map (raster type) with SOC content based on an interpolation using the ArcGIS 10.1 program.
Results and discussion
SOC values obtained for the ScRMA ranged from 9 to 133 t·ha-1, with a mean value of 36.31 t·ha-1, and a standard deviation of 23.83 t·ha-1, (Table 1). These values were obtained from information of soil profiles with depths of 12 to 30 cm; considered based on previous regional studies for the same depth range, which is where the highest SOC concentrations are found (Burke et al., 1989).
Table 1 Soil organic carbon, location and characterization of soil profiles (1992 to 2005).
Site | SOC (t·ha-1) | West longitude | North latitude | Municipality | Type of climate | WRB1 classification | Elevation (m a. s. l.) | Pp (mm) | Vegetation | Slope |
---|---|---|---|---|---|---|---|---|---|---|
1 | 28.92 | 102° 43’ 57.42’’ | 25° 00’ 56.50’’ | Viesca | Very arid, semiwarm | Cambisol | 1 500 | 300 | Scrubland | 3 |
2 | 13.53 | 103° 03’ 28.76’’ | 25° 05’ 41.04’’ | Viesca | Very arid, semiwarm | Calcisol | 1 450 | 300 | Scrubland | 3 |
3 | 50.08 | 102° 50’ 27.44’’ | 25° 04’ 35.88’’ | Viesca | Very arid, semiwarm | Calcisol | 1 450 | 300 | Scrubland | 3 |
4 | 20.27 | 103° 19’ 53.49’’ | 24° 55’ 29.31’’ | Gral. Simón Bolívar | Very arid, semiwarm | Calcisol | 1 450 | 300 | Scrubland | 1 |
5 | 31.28 | 102° 54’ 11.69’’ | 24° 54’ 25.27’’ | Viesca | Very arid, semiwarm | Calcisol | 1 550 | 300 | Scrubland | 1 |
6 | 39.23 | 103° 14’ 08.05’’ | 24° 54’ 10.28’’ | Gral. Simón Bolívar | Very arid, semiwarm | Calcisol | 1 350 | 300 | Irrigation | 1 |
7 | 21.98 | 103° 03’ 14.32’’ | 24° 49’ 07.67’’ | Torreón | Very arid, semiwarm | Calcisol | 1 450 | 300 | Scrubland | 2 |
8 | 17.71 | 102° 53’ 58.21’’ | 24° 49’ 26.67’’ | Viesca | Very arid, semiwarm | Calcisol | 1 500 | 300 | Scrubland | 1 |
9 | 42.64 | 103° 11’ 24.19’’ | 24° 45’ 16.90’’ | Gral. Simón Bolívar | Very arid, semiwarm | Kastañozem | 1 450 | 300 | Irrigation | 1 |
10 | 12.64 | 103° 13’ 51.42’’ | 24° 41’ 55.09’’ | Gral. Simón Bolívar | Arid, semiwarm | Calcisol | 1 550 | 300 | Irrigation | 1 |
11 | 53.06 | 103° 30’ 40.76’’ | 24° 33’ 03.28’’ | Santa Clara | Arid, semiwarm | Chernozem | 1 800 | 400 | Rainfed | 2 |
12 | 9.03 | 103° 33’ 45.44’’ | 24° 07’ 43.88’’ | Miguel Auza | Semiarid, temperate | Planosol | 2 150 | 500 | Gallery vegetation | 2 |
13 | 21.24 | 103° 14’ 52.94’’ | 24° 58’ 38.80’’ | Torreón | Very arid, semiwarm | Leptosol | 1 325 | 300 | Scrubland | 40 |
14 | 30.12 | 103° 25’ 41.33’’ | 24° 57’ 23.53’’ | Cuencamé | Very arid, semiwarm | Calcisol | 1 470 | 300 | Scrubland | 1 |
15 | 55.77 | 103° 06’ 31.95’’ | 24° 44’ 07.77’’ | Gral. Simón Bolívar | Very arid, semiwarm | Calcisol | 1 470 | 300 | Scrubland | 2 |
16 | 36.39 | 103° 19’ 45.77’’ | 24° 32’ 02.44’’ | Santa Clara | Arid, semiwarm | Leptosol | 1 748 | 400 | Scrubland | 2 |
17 | 133.72 | 103° 32’ 46.08’’ | 24° 42’ 37.61’’ | Cuencamé | Arid, temperate | Leptosol | 2 007 | 400 | Scrubland | 30 |
18 | 87.48 | 103° 28’ 56.13’’ | 24° 25’ 21.60’’ | Santa Clara | Arid, semiwarm | Vertisol | 1 823 | 400 | Grassland | 1 |
19 | 45.66 | 103° 11’ 44.63’’ | 24° 23’ 08.86’’ | Juan Aldama | Semiarid, temperate | Luvisol | 1 946 | 400 | Scrubland | 1 |
20 | 16.87 | 103° 38’ 04.23’’ | 24° 08’ 42.46’’ | Cuencamé | Semiarid, temperate | Umbrisol | 2 105 | 600 | Rainfed | 1 |
21 | 29.30 | 103° 35’ 22.82’’ | 23° 57’ 30.91’’ | Sombrerete | Semiarid, temperate | Luvisol | 2 250 | 500 | Rainfed | 1 |
22 | 10.83 | 102° 53’ 00.43’’ | 23° 56’ 44.56’’ | Gral. Fco. R. Murguía | Arid, semiwarm | Calcisol | 1 843 | 400 | Scrubland | 10 |
23 | 28.60 | 103° 31’ 12.49’’ | 25° 11’ 53.45’’ | Gral. Simón Bolívar | Very arid, semiwarm | Calcisol | 1 250 | 300 | Scrubland | 5 |
24 | 20.48 | 103° 08’ 46.07’’ | 25° 17’ 34.75’’ | Viesca | Very arid, semiwarm | Calcisol | 1 200 | 200 | Scrubland | 4 |
25 | 12.28 | 102° 38’ 17.09’’ | 24° 39’ 17.18’’ | San Juan de Guadalupe | Very arid, semiwarm | Regosol | 1 600 | 300 | Scrubland | 2 |
26 | 65.85 | 103° 42’ 20.67’’ | 24° 32’ 22.29’’ | Cuencamé | Semiarid, temperate | Chernozem | 2 100 | 500 | Rainfed | 1 |
27 | 41.27 | 103° 46’ 02.88’’ | 24° 23’ 35.07’’ | Cuencamé | Semiarid, temperate | Phaeozem | 2 100 | 500 | Irrigation | 1 |
28 | 25.84 | 102° 59’ 07.95’’ | 24° 22’ 32.92’’ | Gral. Fco. R. Murguía | Arid, semiwarm | Leptosol | 1 800 | 400 | Scrubland | 15 |
29 | 18.58 | 103° 18’ 14.89’’ | 24° 06’ 22.75’’ | Miguel Auza | Semiarid, temperate | Luvisol | 2 150 | 500 | Rainfed | 1 |
30 | 25.12 | 102° 38’ 47.63’’ | 24° 59’ 30.29’’ | San Juan de Guadalupe | Very arid, semiwarm | Calcisol | 1 413 | 300 | Scrubland | 1 |
31 | 13.00 | 102° 40’ 48.31’’ | 24° 42’ 35.30’’ | San Juan de Guadalupe | Very arid, semiwarm | Solonetz | 1 526 | 300 | Scrubland | 0 |
32 | 42.16 | 102° 43’ 41.64’’ | 24° 38’ 09.46’’ | San Juan de Guadalupe | Very arid, semiwarm | Leptosol | 1 589 | 300 | Scrubland | 50 |
33 | 26.69 | 103° 39’ 37.26’’ | 24° 49’ 01.00’’ | Cuencamé | Arid, semiwarm | Leptosol | 1 675 | 400 | Scrubland | 4 |
34 | 63.86 | 103° 47’ 02.75’’ | 24° 44’ 54.21’’ | Cuencamé | Arid, temperate | Phaeozem | 1 822 | 400 | Scrubland | 20 |
35 | 22.89 | 103° 40’ 12.78’’ | 24° 38’ 44.46’’ | Cuencamé | Arid, temperate | Leptosol | 1 953 | 500 | Scrubland | 15 |
36 | 53.55 | 103° 42’ 39.16’’ | 24° 43’ 08.51’’ | Cuencamé | Arid, semiwarm | Phaeozem | 1 805 | 400 | Scrubland | 30 |
37 | 50.17 | 103° 49’ 50.58’’ | 24° 25’ 55.69’’ | Cuencamé | Semiarid, temperate | Phaeozem | 2 088 | 500 | Rainfed | 1 |
38 | 56.67 | 103° 41’ 16.07’’ | 24° 21’ 12.38’’ | Cuencamé | Semiarid, temperate | Phaeozem | 2 052 | 500 | Grassland | 1 |
39 | 47.27 | 103° 50’ 04.87’’ | 24° 15’ 45.29’’ | Cuencamé | Semiarid, temperate | Phaeozem | 2 131 | 600 | Rainfed | 1 |
40 | 30.19 | 102° 54’ 06.67’’ | 24° 16’ 19.65’’ | Gral. Fco. R. Murguía | Arid, semiwarm | Leptosol | 1 705 | 300 | Scrubland | 25 |
1WRB = World Reference Base for Soil Resources. Source: Instituto Nacional de Estadística y Geografía (INEGI, 2014a).
SOC concentrations varied in the ScRMA; 84.5 % of its extension values ≤ 50 t·ha-1 were obtained, while 14.5 % of the surface area varied from 51 to 100 t·ha-1, and almost 1 % had concentrations > 100 t·ha-1. The first range of SOC concentrations (10 to 50 t·ha-1) was distributed in 49.80 % of the scrubland area located in the central-northern portion of the sub-basin, specifically northeast of the municipality of Santa Clara, west of San Juan de Guadalupe, south of Viesca, southeast of Torreón and Simón Bolívar, and in a small portion north of Gral. Fco. R. Murguía. 19.63 % of the same concentration occurred in annual rainfed agricultural areas found in the southeastern part of the sub-basin, south of the municipality of Cuencamé, almost in the entirety of Miguel Auza, south of Simón Bolívar and in the center of Juan Aldama. The 11.5% was found in areas of secondary scrubland vegetation, in some parts of the municipalities of Viesca, Torreón, San Juan de Guadalupe and Simón Bolívar. Finally, 10 % occurred in secondary grassland vegetation, in some parts of Juan Aldama, south of Simon Bolivar, Viesca, Cuencamé and Santa Clara. The remaining 9.66% corresponds to land use and vegetation with a distribution of less than 1 % each.
The second range of SOC (51 to 100 t·ha-1) was found in the west of the sub-basin, where areas with secondary grassland vegetation had this concentration in 42.5 % of their surface, especially in the central-eastern part of Cuencamé and central-western part of Santa Clara. The 24.9 % was distributed in areas with annual rainfed agriculture, in the center-west of Santa Clara, and 22.9 % was in scrubland in the north-northwest part of Santa Clara. Finally, the third range (> 100 t·ha-1) was located west of the ScRMA, in areas of grassland and secondary grassland vegetation.
When comparing interpolators of the ArcGIS 10.1 program, it was found that IDW and Kriging had the best interpolation based on the basic statistics of mean and standard deviation (Table 2).
Table 2 Comparison of interpolators to determine soil organic carbon (SOC).
No. profiles | Minimum SOC (t·ha-1) | Maximum SOC (t·ha-1) | Interpolator | Minimum estimated SOC (t·ha-1) | Maximum estimated SOC (t·ha-1) | Mean (t·ha-1) | Standard Deviation |
---|---|---|---|---|---|---|---|
40 | 9.03 | 133.72 | IDW | 9.03 | 133.72 | 37.56 | 15.63 |
KRIGING | 15.35 | 113.82 | 38.51 | 12.72 | |||
NATURAL NEIGHBOR | 9.48 | 132.12 | 39.59 | 18.09 | |||
SPLINE | -5.33 | 156.45 | 40.93 | 27.18 |
In the comparison of the powers of the IDW and Kriging interpolators, it was determined that exponential ordinary Kriging (KO) best represented the SOC values of the ScRMA based on the statistics obtained (Table 3).
Table 3 Comparison between interpolator powers to determine soil organic carbon (SOC).
Interpolator | Power | Minimum estimated SOC (t·ha-1) | Maximum estimated SOC (t·ha-1) | Mean (t·ha-1) | Standard Deviation |
---|---|---|---|---|---|
IDW | P1 | 11 | 122 | 37.64 | 10.81 |
P2 | 9 | 133 | 37.50 | 15.58 | |
P3 | 9 | 133 | 37.67 | 19.28 | |
P4 | 9 | 133 | 37.93 | 21.67 | |
Kriging | Spherical K. O. | 15 | 113 | 38.51 | 12.71 |
Circular K. O. | 22 | 73 | 37.88 | 11.12 | |
Exponential K. O. * | 10 | 129 | 38.17 | 12.35* | |
Gaussian K. O. | 16 | 106 | 38.10 | 8.67 | |
Linear K. O. | 23 | 65 | 37.89 | 10.26 | |
Linear K. U. with linear derivative | 16 | 61 | 35.07 | 9.24 | |
Linear K. U. with quadratic derivative | 9 | 101 | 41.16 | 16.93 |
Villatoro et al. (2008) compared IDW and Kriging interpolators, because they are the most used in spatial variation analysis studies, in an area of 2 467 m2 and georeferenced 61 points at a distance of 3.5 m from each other; soil samples were obtained at a depth of 0 to 15 cm at each point. The authors used the GS+ program for Windows for variogram analysis, interpolation and cross-validation. Interpolations were performed with soil pH, Ca, CEC and phosphorus (P) values, and they determined that, although both interpolators had similar performance, Kriging was superior in predicting better the variation of pH, Ca and CEC, while IDW was better with P.
Interpolation was represented in nine classes to better visualize the SOC distribution (Figure 2). It was observed that values higher than 50 t·ha-1 are distributed from the central zone towards the west, belonging, in a larger area, to the state of Durango, where lithosol, xerosol and chernozem soil types are found. This zone is dominated by desert microphyllous and rosetophyllous scrub, natural grassland and secondary natural grassland vegetation, with a rainfall of 400 to 600 mm.
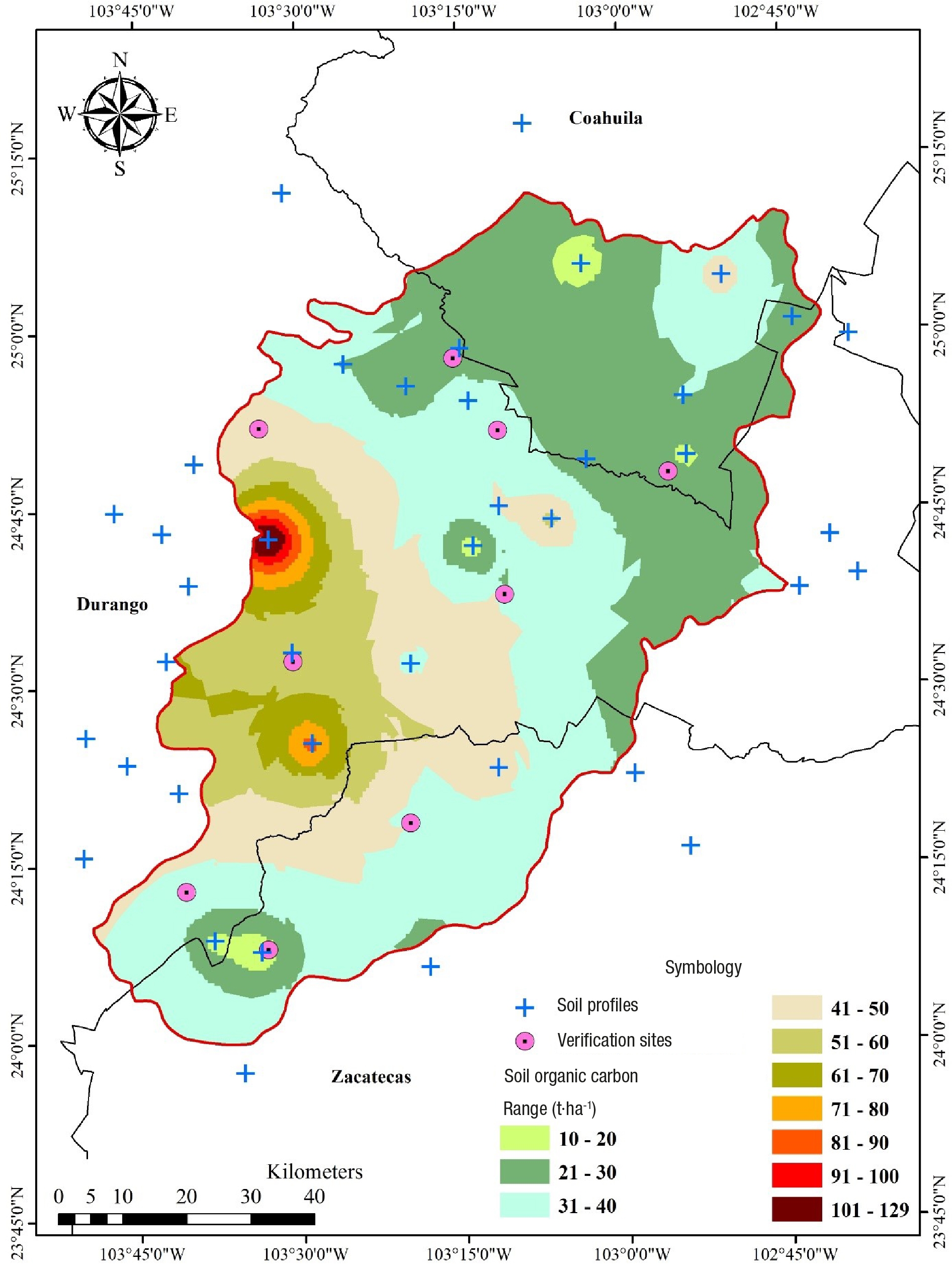
Figure 2 Soil organic carbon (SOC) in the Medio Aguanaval river sub-basin obtained using the Kriging interpolator.
The lowest SOC concentration is distributed in the south part of Coahuila and the northeast part of Zacatecas. Vela-Correa et al. (2012) reviewed the reported SOC concentrations to have a general reference and be able to classify them into high and low, according to their values. These authors concluded that there is no reference where a general hierarchy of SOC is established, so they considered the limits of the intervals according to the maximum and minimum values of C concentration obtained in the soil analyses.
Segura-Castruita et al. (2005) estimated the SOC content in the surface horizon (0 to 20 cm) of 4 583 soil samples and generated a raster map by interpolation. They found values ranging from 0.2 to 493 t·ha-1, which they divided into five classes. The values corresponding to the sub-basin area ranged from 25 to 50 t·ha-1, which agrees with the results of this study, with a mean of 36 t·ha-1. Similarly, the results agree with a study by FAO (2017a), where they produced a global organic carbon map with one-kilometer resolution using samples obtained at a depth of 0 to 30 cm. In that study, ScRMA presented SOC values of 25 to 40 t·ha-1, and in this study a mean of 24.4 t·ha-1 was obtained in the analysis of ScRMA verification sites (Table 4).
Table 4 Soil organic carbon (SOC) at verification sites (October 2019).
Site | SOC (t·ha-1) | West longitude | North latitude | Municpality | Type of climate | WRB1 classification | Elevation (m a. s. l.) | Pp (mm) | Vegetation | Slope |
---|---|---|---|---|---|---|---|---|---|---|
1 | 11.00 | 103° 33’ 09.80’’ | 24° 07’ 56.20’’ | Miguel Auza | Semiarid, temperate | Phaeozem | 2 156 | 300 | Scrubland vegetation with natural grassland | 1 |
2 | 21.84 | 103º 40’ 40.80’’ | 24° 12’ 51.60’’ | Cuencamé | Semiarid, temperate | Umbrisol | 2 170 | 300 | Scrubland vegetation of natural grassland | 1 |
3 | 27.35 | 103° 30’ 38.80’’ | 24° 32’ 16.30’’ | Cuencamé | Semiarid, temperate | Chernozem | 1 652 | 300 | Rainfed | 2 |
4 | 11.65 | 103° 10’ 59.30’’ | 24° 37’ 46.00’’ | Simón Bolívar | Arid, semiwarm | Phaeozem | 1 664 | 300 | Shurbland | 1 |
5 | 6.37 | 103° 33’ 33.80’’ | 24° 51’ 59.50’’ | Cuencamé | Arid, semiwarm | Calcisol | 1 610 | 300 | Scrubland | 1 |
6 | 8.92 | 102° 55’ 42.10’’ | 24° 47’ 59.80’’ | Simón Bolívar | Very arid, semiwarm | Calcisol | 1 476 | 300 | Veg. Sec. Arb. de matorral | 1 |
7 | 40.10 | 103° 19’ 56.50’’ | 24° 18’ 32.50’’ | Juan Aldama | Semiarid, temperate | Chernozem | 2 023 | 300 | Rainfed | 1 |
8 | 41.84 | 103° 11’ 27.30’’ | 24° 51’ 38.80’’ | Simón Bolívar | Very arid, semiwarm | Calcisol | 1 386 | 300 | Mesquite forest | 1 |
9 | 50.53 | 103° 15’ 30.90’’ | 24° 57’ 47.10’’ | Torreón | Very arid, semiwarm | Leptosol | 1 404 | 300 | Scrubland | 3 |
1WRB = World Reference Base of Soil Resources. Source: Instituto Nacional de Estadística y Geografía (INEGI, 2014a).
It is important to consider that the number of sites sampled for verification was low (nine sites), although these were representative of the different soil types and uses, compared to the 40 sites analyzed from the INEGI soil profiles, where the mean obtained was 36 t·ha-1. Nevertheless, the results obtained with soil sampling in the ScRMA were no different from those reported by both FAO (2017a) and Segura-Castruita et al. (2005). Regarding the precision of the estimates made, the ECM (mean squared error) statistic was calculated for the SOC data of the analyzed profiles and verification sites (23.83 and 16.41 t·ha-1, respectively), and the coefficient of variation for the same data (65.64 and 67.27 %, respectively) (Table 5).
Table 5 Descriptive statistics of soil profiles and verification sites in the Medio Aguanaval River sub-basin (ScRMA).
Number of sites | Mean (t·ha-1) | Mean square error (t·ha-1) | Coefficient of variation (%) |
---|---|---|---|
40 (soil profiles) | 36.31 | 23.83 | 65.64 |
9 (verification sites) | 24.40 | 16.41 | 67.27 |
The highest concentration of SOC is found in natural grassland areas, annual irrigated and annual rainfed agriculture, and desert microphilous scrubland that belongs to the central part, which corresponds to the state of Durango (1 600 to 2 200 m a. s. l.).
Mondal et al. (2017) estimated the distribution of SOC in varied categories of slope, soil, and land use, and observed that SOC concentration is higher in low areas and for clay soils of lands with agricultural vocation. These authors also reported that SOC stock decreases with greater slope, and that clay has the highest carbon content in soil categories.
SOC information refers mainly to temperate and tropical regions (Segura-Castruita et al., 2005), because arid and semi-arid regions have been poorly studied. Montaño et al. (2016) and Yescas-Coronado et al. (2018) mention that spatio-temporal heterogeneity of these regions is influenced by environmental conditions, plant community type, and land use, which together affect carbon stocks and fluxes.
Several authors indicate that climate, via temperature and precipitation, plays a dominant role in SOC accumulation (Grigal & Ohmann, 1992; Batjes, 1999; Yigini & Panagos, 2016; Batjes, 2016; FAO, 2017c; FAO, 2019). Furthermore, it has been determined that soils of rainforests, mangroves and mountain mesophyll forests contribute more than 100 Mg·ha-1 of CO, while grasslands, desert and thorn forest contribute less than 30 Mg·ha-1 of CO. On the other hand, when relating the main land uses with SOC content, it was established that soils used for intensive livestock have the highest SOC content (> 80.0 Mg·ha-1), followed by forest soils (> 75.0 Mg·ha-1), while irrigated agricultural soils have the lowest amount of this compound (40.8 Mg·ha-1) (Segura-Castruita et al., 2005).
Therefore, low SOC concentrations (10 to 30 t·ha-1) in ScRMA are distributed mainly in the northeastern part of the zone, where the dominant vegetation is scrubland (desert) and secondary scrubland vegetation. Precipitation ranges from 125 to 400 mm, and the climate is very arid and semi-warm. SOC concentrations can be attributed to the low contribution of organic matter to the soil by vegetation and aridity of the area, promoting SOC loss.
Conclusions
ScRMA dominated by semiarid conditions showed an estimated mean value of 36.31 t·ha-1 of SOC. In terms of distribution, the municipalities with the highest SOC concentration were Cuencamé and Santa Clara, while the lowest records were found in Torreón. This interspatial variation depends on climatic and soil variables and the type of management of the area, where the highest SOC content is generated in sites with higher precipitation and soil depth.
The main limitation in this research was the lack of information on apparent density, which affected the precision of SOC reserve estimates. Therefore, and based on the development of this study, it was estimated that at a density of 0.6 % sampling, uncertainty decreased from 23.83 to 16.41 t·ha-1 (ECM) in this heterogeneous ecosystem.