Introduction
In the 1980s, Bill Lidicker proposed conceptual models to explain the semi-regular population fluctuations (aka cycles) of arvicoline (= microtine) rodents. These models invoked several potential drivers that were extrinsic to the voles themselves, including landscape structure, food supply, and predation, as well as several that were intrinsic, including aggressive behavior, dispersal dynamics, and physiological stress. He called his approach a multi-factor perspective (Lidicker 1988), and predicted that the extrinsic and intrinsic factors he invoked would vary in strength, or even appear and disappear, at different times, both seasonally and between years. The factors were also expected to interact. For instance, variation in aggressive behavior might cause changes in dispersal rates, the effects of which would depend on landscape structure (e. g., the ratio of optimal to marginal patch area, or ROMPA; Lidicker 2000). Lidicker’s goal was to explain a type of pattern - population fluctuations with two to five years between peaks - that varies in its specific manifestations both within and between species. The pattern of population fluctuations can vary in magnitude and timing of peaks, as well as the rate of population growth and decline. Thus, his conceptualization of the problem was holistic, in the sense that he defined “cycles” as complex and varying phenomena, and his approach to addressing the problem was also holistic, in the sense that he saw specific mechanisms interacting in complex, hierarchical ways (Lidicker 1988).
Lidicker’s approach was criticized by researchers who saw arvicoline cycles as a homogeneous phenomenon rather than a variable but recognizable pattern (Gaines et al. 1991; Lidicker 1991). Critics with a simpler, more unitary definition of the problem developed hypotheses in which a single factor (e. g., heritable aggression or specialist predators) by itself explained cycles. Such a focus was more reductionist, and usually neglected the interactions between variables. Hypotheses arising from single-factor, reductionist approaches are simpler, more tractable experimentally, and appealing for many researchers. Although critics were vociferous in the 1980s and 1990s, by the 2000s most research on arvicoline cycles incorporated multi-factor, holistic perspectives (Krebs 2013).
We were both Ph. D. students under Lidicker’s guidance, Ostfeld in the 1980s and Keesing in the 1990s. Although we have both focused on the ecology of small mammals, we have paid less attention to the causes of fluctuations in population size and more attention to the consequences of those fluctuations for other ecological phenomena. Changes in the abundance of small mammals through time or across space often affect the transmission of the pathogens and parasites of those mammals. Rodents in particular are important hosts for zoonotic pathogens, those that vertebrate animals share with humans (Keesing and Ostfeld 2021).
Infectious disease ecology has its roots firmly anchored in population biology, just as arvicoline ecology does. A central question in disease ecology is what causes populations of pathogens, and the diseases they cause, to fluctuate through time or across space. As in arvicoline ecology, defining disease dynamics narrowly, e. g., by focusing on changes from endemic to epidemic phases, or simply by studying a particular outbreak, often elicits a search for simple explanations or hypotheses. For example, if one defines a disease system as limited to one host species and one pathogen, one will tend to seek answers in a reductionistic way, without reference to a broader context and the interactions that arise from that context. Perhaps what Lidicker called a multifactorial perspective could instead be termed an inclusive perspective, in the sense of broadening the conception of the phenomenon to be explained, as well as of the cause-effect relationships, to include the potential for complex manifestations and contingencies to play important roles. Below we describe three areas of disease ecology that we have addressed in our own research, highlighting the ways a more inclusive perspective, or Lidickerian view, helps advance understanding compared to a more traditional approach.
Replacing the single-reservoir model for most zoonoses. Broadly defined, emerging infectious diseases (EIDs) are those that are caused by pathogens that have recently evolved, recently spilled over into humans, suddenly increased in incidence, or suddenly expanded in geographic range (Morse 1995; Rosenthal et al. 2015). Most of the diseases of humans that are classified as emerging are caused by zoonotic pathogens. The vertebrate hosts in which the pathogens originated can be responsible for any and all of these defining aspects of emergence. Identifying the source of the emerging pathogen is expected to increase our understanding of the cause of disease emergence and suggest pathways to disease control and prevention. Consequently, much attention has been paid to determining the vertebrate source of emergence event(s).
The field of infectious disease ecology has traditionally been dominated by the “single-pathogen, single-host” paradigm, whereby it is assumed that pathogens have evolved to infect a single host species. Cross-species transmission, including transmission from a reservoir host to humans, is considered anomalous, as illustrated by use of terms like “spillover” or “species jump” to describe transmission from the focal host to some other host. Consequently, the search for the reservoir for a particular zoonotic pathogen that causes a particular EID typically focuses on identifying a single reservoir host. Such a focus is exemplified by the ongoing search for the reservoir responsible for the initial zoonotic transmission of SARS CoV-2 from some wildlife species to humans.
In reality, pathogens span a continuum of host-specificity, from those that truly infect only a single host species, to those that typically infect few species, to those that are highly promiscuous (Figure 1). Zoonotic pathogens, by definition, must infect at least two species of host - a vertebrate reservoir and humans. Pathogens that undergo a species jump from an animal reservoir to humans tend to be more promiscuous (Keesing and Ostfeld 2021), frequently being shared with numerous host species. After all, these are the “jumpers”. Occurrence in multiple species of hosts appears to be a general characteristic of the pathogens that cause EIDs in humans, and indeed, sequential infection of different host species can result in genetic changes that increase transmission to, or virulence in, new hosts, as exemplified by influenza viruses.

Figure 1 The paradigm (a) and the reality (b) for research on the transmission of zoonotic pathogens from vertebrate animals to humans. (a) The paradigm emphasizes a single animal host species for a zoonotic pathogen and an original spillover event, though the event and the species are rarely identified. (b) In reality, most zoonotic pathogens have multiple host species whose specific roles in transmission to and from humans are rarely known. Adapted from a figure in Keesing and Ostfeld (2021).
Focusing on a single reservoir host for a zoonotic pathogen, although appealingly simple, can be misleading and detrimental to mitigating disease. For instance, attempts to manage a reservoir host may be inefficient or even counterproductive if other reservoirs go unrecognized and unmanaged. Recognizing that multiple vertebrate species are involved in cross-species transmission of a given pathogen, including to humans, raises an inconvenient truth - that identifying pathways of zoonotic spillover involves quantifying transmission from several or many hosts rather than just one. Nevertheless, such research is likely to be critical for predicting and mitigating disease emergence (Keesing and Ostfeld 2021). A Lidickerian expansion of causal pathways is further required by the observation that some hosts play a protective role, reducing transmission rates of zoonotic pathogens by regulating abundance of pathogens (e. g., by absorbing but not onwardly transmitting them) or by regulating the reservoir species themselves via predation and competition (Keesing et al. 2009; Levi et al. 2012; Ostfeld et al. 2018; Keesing and Ostfeld 2021).
Broadening the focus to include multiple, interacting pathogens. Just as most pathogens infect more than one species of host, hosts are virtually always infected by more than one species of pathogen. Again, the reductionist adoption of a one host-one pathogen framework fails. There is room in such a framework to explore infection dynamics of a given pathogen as a function of a host individual (e. g., immune status) or of a host population (e. g., density), but there is no room to explore the potential effects of coinfecting pathogens. Two examples from the literature on small mammal populations illustrate the importance of explicit recognition of coinfecting pathogens within hosts.
Telfer et al. (2010) studied four fluctuating populations of the field vole, Microtus agrestis, in the UK, repeatedly taking blood samples from individual voles to track changes in the presence of the pathogens Bartonella spp. (transmitted by fleas), Babesia microti (transmitted by ticks), Anaplasma phagocytophilum (transmitted by ticks), and antibodies to cowpox virus (transmitted directly between hosts). Despite the potential for pathogen species within a host to interact, either directly via resource competition or indirectly via suppression or activation of immune pathways, the dynamics of pathogens have typically been addressed in isolation from each other (Telfer et al. 2010). Telfer et al. analyzed the probability of infection occurring between time steps (vole captures) for each pathogen as a function of traditional host and environmental variables, as well as of the presence of the other pathogens in the individual host.
Strikingly, Telfer et al. (2010) found that the presence of other pathogens generally had stronger effects on infection risk than did “factors related to exposure risk and host condition, such as age and season” (p. 244). For example, infection with cowpox virus consistently increased susceptibility to the other pathogens approximately two-fold. Voles infected with A. phagocytophilum were less likely to become infected with B. microti. And, voles infected with B. microti showed reduced susceptibility to Bartonella spp. but increased susceptibility to A. phagocytophilum. Telfer et al. argued that these four groups of pathogens (which notably constitute a subset of those infecting these voles) occur as an interaction web, affecting the host’s susceptibility to other pathogens with predictably strong magnitude.
Hersh et al. ( 2014) addressed the frequency of coinfection in blacklegged ticks (Ixodes scapularis) in the northeastern United States with multiple tick-borne pathogens and sought to determine its cause. Coinfection in ticks with more than one zoonotic pathogen can result in simultaneous transmission of multiple pathogens to humans, with medical and epidemiological consequences. Ticks must acquire these pathogens from blood feeding on wildlife hosts, as the pathogens are not vertically transmitted. Hersh et al. found that coinfection of individual ticks with B. microti and the agent causing Lyme disease, Borrelia burgdorferi, was about twice as frequent as expected if the two pathogens were acquired independently (Figure 2). Moreover, they determined that ticks that had previously fed from specific small mammal hosts, especially white-footed mice (Peromyscus leucopus) and eastern chipmunks (Tamias striatus), were much more likely to be coinfected than were ticks that had fed from other hosts.
These two examples indicate that less inclusive approaches to pathogen infection in hosts and vectors - those that treat each pathogen in isolation from others - can overlook important factors driving infection risk and disease dynamics. A more inclusive approach that recognizes broader contexts is more accurate and potentially more useful for disease management.
Incorporating concepts from food webs into dynamics of pathogen and vector populations. In his paper arising from the C. Hart Merriam Award from the American Society of Mammalogists, Lidicker (1988) provided a conceptual diagram of the known and suspected factors affecting population size of voles (Figure 2 in Lidicker 1988). An inner, proximate, zone of factors included demographic processes that together produce population dynamics. The outer layers emphasized multiple drivers extrinsic to the vole population, including food resources, predators, competitors, and parasites. His conceptual model therefore subsumed several of the “single-factor” hypotheses that were ascendant at that time, including the hypotheses that time delays in the interactions between voles and their food supply (vegetation) and between voles and their predators were responsible for driving cyclic fluctuations (Krebs 2013). Lidicker’s advance was to explicitly recognize that different extrinsic causal pathways could operate - simultaneously or sequentially - and by acting on one or more intrinsic factors, result in a recognizable population cycle.
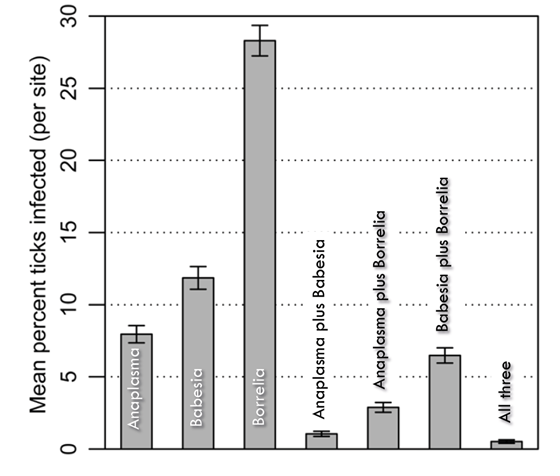
Figure 2 Mean percentage of infection for 4,368 ticks at forested sites. Ticks were coinfected with multiple pathogens more frequently than expected by chance. Each cat egory represents the overall prevalence of a pathogen - for example, the “Anaplasma” bar represents not just single infections but also ticks co-infected with Anaplasma and either or both of the other two pathogens. Error bars represent standard error. Adapted from a figure in Hersh et al. (2014).
Much of our research in disease ecology in recent decades has focused on understanding what causes the risk of human exposure to zoonotic disease to vary in time and space. Many medical entomologists studying tick population dynamics have tended to invoke simple models in which ambient temperature and/or measures of available moisture (e. g., relative humidity, vapor pressure deficit) cause patterns of tick abundance by affecting tick mortality (Sonenshine and Roe 2014). These expectations arise from extension of simple, laboratory experiments in which ticks succumb to both high and low temperatures and low humidity (Ostfeld and Brunner 2015) . Other tick experts have focused on the abundance of specific vertebrate hosts for ticks, such as white-tailed deer (Odocoileus virginianus), as the primary driver of blacklegged tick abundance. This expectation arises from observations of large numbers of adult blacklegged ticks on hunter-killed deer during the autumn hunting season, followed by the supposition that more deer leads to greater numbers of adult female ticks surviving and reproducing, which consequently drives population dynamics (Ostfeld 2011). Both of these simple conceptual models could be true in theory, but neither is supported by direct evidence (Ostfeld 2011), and basic natural history observations suggests they are likely to be inadequate. For example, blacklegged ticks live in environments in the upper Midwestern United States and Canada, where ambient conditions are more extreme than those that predictably kill ticks in the laboratory (Ostfeld and Brunner 2015). In addition, blacklegged ticks are extreme host generalists, parasitizing dozens of species of vertebrates within local areas, with pronounced variation in host associations between life stages (larvae, nymphs, adults; Ostfeld 2011). Consequently, strong dependence of tick population dynamics on a single host species might not be expected.
We have focused on the abundance of blacklegged ticks that are infected with zoonotic pathogens as a primary indicator of human risk (Ostfeld and Keesing 2000; Allan et al. 2003). Thirty years of monitoring the dynamics of blacklegged tick populations in New York State reveals that the abundance of infected nymphs is significantly correlated with the prior abundance of rodents, specifically white-footed mice and eastern chipmunks. The effect of deer abundance is weak or absent (Ostfeld et al. 2006, 2018). Furthermore, population density of both of these rodent species is correlated with prior abundance of acorns produced by local oak trees (Quercus spp.), which is an important source of food over winter (Ostfeld et al. 1996; Jones et al. 1998). Such observations lead to a multifactor model dominated by bottom-up forcing, from resources (acorns) to consumers (rodents) to parasites on those consumers (ticks and tick-borne pathogens; Figure 3). More recently, another pathway has materialized as an important contributor to tick and pathogen dynamics. Strong spatial patterns in the prevalence of infection in tick populations, whereby some tick populations have very high and others very low prevalence of zoonotic pathogens, are statistically associated with specific guilds of mammalian predators. Ticks in sites in eastern New York with diverse guilds of predators, including red foxes (Vulpes vulpes), raccoons (Procyon lotor), Virginia opossums (Didelphis virginiana), and striped skunks (Mephitis mephitis) have significantly lower infection prevalence than do sites with less diverse predator assemblages (Ostfeld et al. 2018). These depauperate predator assemblages appear to occur when coyotes (Canis latrans) supplant other predators (Levi et al. 2012). The diverse group of predators appears to reduce tick infection prevalence by multiple pathways, including reducing the number of rodent hosts responsible for transmitting infection (i. e., the reservoir hosts), by deflecting tick meals away from rodents onto the predators, all of which are poorer reservoirs (LoGiudice et al. 2003), and by killing ticks directly via more fastidious grooming than occurs with the rodents (Keesing et al. 2009). Statistically, neither bottom-up nor top-down forces explain more than half of the variation in human exposure risk, but together they provide a predictive understanding of changing risk in space and time.
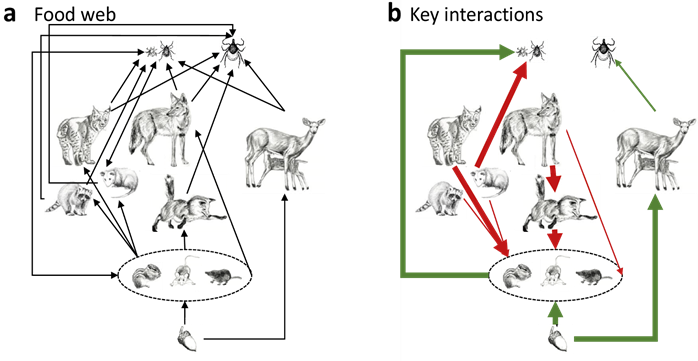
Figure 3 Webs of connections among species in the forests of eastern North America. (a) Trophic relationships, with arrows drawn from resource to consumer. (b) Key interactions, with arrow width representing the strength of relationships, and green and red representing positive and negative effects, respectively. Adapted from a figure in Ostfeld et al. (2018).
Synthesis. These studies have taken a holistic perspective to invoke multiple, interacting factors as drivers of variation in zoonotic risk. Investigating the dynamics of these complex systems sometimes requires exploring factors in isolation, e. g. creating an acorn pulse (Jones et al. 1998), while recognizing that each factor may interact with others, change in strength, experience feedback, and depend on factors outside the study system. Studying systems with so many interacting components is challenging but not intractable - these systems can be explored using experimentation, comparative or correlative approaches, and modeling. One key acknowledgment is that the very phenomena being explored may in fact be variable, showing similar patterns that are perhaps caused by different underlying processes that vary in space and time. Lidicker’s multifactor approach to arvicoline population dynamics continues to inform and enlighten other research areas in ecology, including the study of infectious diseases.