Introduction
The regulation of minimum wages (MW) has been a focus of national economic policy in Mexico for decades. However, public policies were dominated by the need to adjust to high inflation during the seventies and early-nineties, by the need to adjust to decreasing and low inflation from the early-nineties until recently and, currently, by the idea that it must be enough to pay for a basket of consumption of a family.
Economic models predict that MW can have effects on employment and on the distribution of earnings. For public policy purposes, it is important to measure the size of the effects because predictions involve redistribution and deadweight loss: some individuals can keep a job and obtain wage increases, while others become unemployed or move to the uncovered sector.
We estimate the effect of MW on the wage distribution of employment near the MW, using the events of change during the 2005-2019 period. MW policy is set at a national level and events of regional change are sparse. Thus, most of the variability in interventions comes in the form of changes over time of the national MW, and changes in regional coverage that are also defined federally. On the other hand, the social and economic conditions vary across municipalities, and the local labor markets respond differently to the same federal regulation.
To identify the effect of changes in MW on employment, we assume a stationary labor market on which relatively small interventions are applied. As suggested by Card and Krueger (1995) the variation in the response of state labor markets to nationally determined MW can allow the identification of the effect of MW on employment and the distribution of wages. However, MW changes in Mexico are predictable, employers and workers may adjust their behavior in anticipation, and the government can make MW increases a function of characteristics of the population. Available data end when MW policy moved from allowing increases that roughly matched inflation, to larger adjustments that affect a larger number of workers.
As argued by Neumark (2019) in his review of the econometrics and economics of the employment effects of MW: “[P]redicting the effects of minimum wage increases of many dollars, based on research studying much smaller increases, is inherently risky for the usual statistical reasons”. In an environment of predictable and relatively small adjustments to MW during a long period of time, as was the case in Mexico during the period under study, we expect firms and workers to adjust behavior and observe a distribution of wages dominated by long-term stability. In that environment, changes in MW perturbate the distribution of wages, and over time firms and workers adjust to return the distribution to its long-term state. The events of change are defined at the municipal level, quarterly, over the period going from 2005 to 2019 (second quarter).
Figure 1 illustrates a possible effect of an increase in MW. The solid lines are the initial distribution of wages and the MW. After an increase in MW to the level signaled by the vertical dotted line, the distribution of wages is perturbed. A potential result is that some jobs are moved from below to above the new MW; this is the result expected by policy makers that promote higher MW. The term missing jobs denotes that the number of jobs below the original distribution of wages is smaller, while the excess jobs are those that move up in the distribution. Our estimates refer to the size of these areas over time (the effect may persist or lose force gradually.

Source: Author´s elaboration
Figure 1 Hypothetical impact of minimum wages on the distribution of wages
Our main result is that MW increases do not have a significant impact on employment (thus, the total size of the missing jobs and excess jobs area in Figure 1 is small). There is little evidence of jobs being moved up the wage distribution. Instead, there seems to be some reshuffling of jobs above MW, and substantial correlation of MW changes with characteristics of the population, putting in evidence that the policy may be endogenous.
Section 1 discusses previous research on the Mexican labor market, section 2 develops the methods of the valuation and explains the data. Section 3 presents the main results and finally we explore policy issues.
I Previous research and recent history of the MW policy
Research on MW policy has been motivated by the issues of equity and inflation expectations, but there is less research on the effects on employment.
A recent calculation related to this research was published in the quarterly report of the Banco de Mexico (2019). It estimates an equation similar to our equation 1 to measure the effect on the state employment-population ratio of the “linked fraction”, defined as the share of the labor force with a salary in December 2018 that was between the 2018 MW and the higher MW in 2019. The main finding is of a negative impact of the 2019 MW increase on the employment-population ratio (a loss of 29% of job growth during the January-April 2019 period). An issue with this report is that it has only one observation to identify the effect of the policy and cyclical issues cannot be addressed.
Other research has studied mainly issues related to earnings. Castellanos, García-Verdú and Kaplan (2004: 507- 533) measure wage rigidity in formal sector contracts and evaluate the covariation between MW and the general wage distribution. While they do not investigate the relation between MW and employment, they find that a significant number of formal workers register at social security at exactly the MW, as well as high correlation between changes in MW and other wages. This is the “lighthouse effect”. The MW policy was dominated by the inflation-targeting policy during most of the period, and inflation expectations dominated the increase in both MW and the general wage (in the language of time series econometrics, both are non-stationary series and their relation is spurious or, more likely, cointegrated due to having a common cause, namely, the inflation expectations variable). Kaplan and Pérez Arce Novaro (2006: 139-173) find that the lighthouse effect became less important after 1993, compared with the 1985-1993 hyperinflationary period.
To simulate the effect of an increase in MW, Campos (2015: 90-106) proposed that “the most compelling evidence points to a null impact on employment of a minimum wage increase if the increase is modest and the original minimum wage is low.” He performed simulations of the impact of a large change in the MW. His benchmark scenario proposes a 51% increase in MW and results in a decrease in employment of 4.6%. Campos, Esquivel and Santillan (2017) study the effect on wages and employment of the increase in MW in 2012 in only some municipalities. They estimate an increase in earnings due to an increase in hours, with no increase in hourly wages and no effect on employment.
Thus, previous research mainly documents: (i) a lighthouse effect, which may be due to the use of the MW as a device to regulate inflationary expectations; and, (ii) correlation between MW and earnings inequality, with little evidence on the causality between MW and the distribution of earnings. There is little research on the relation between MW and employment.
History of MW policy
Between the seventies and the eighties, MW policy was dominated by the high-inflation macro policy; the government frequently updated the MW to keep up with inflation. Starting by the late eighties and until approximately 2016, MW policy became part of the inflation targeting strategy MW increases followed inflation targets, and inflation forecast errors were more often positive than negative, inducing ever lower real MW. By 2016, a political wave took shape to promote real MW increases.
For approximately 10 years (2005-2014), the real minimum wage was kept at an approximately constant value (Figure 2). Figures in tables and graphs are in Mexican pesos, indexed at values of the second quarter of 2019. Real increments were between 2 and 3% from 2016 to 2018, and the 2019 change was 11%. These figures refer to the MW applied in “Zone A” municipalities, which historically had a higher level. I calculate in 37% the average increase weighted by the size of the states’ labor force.
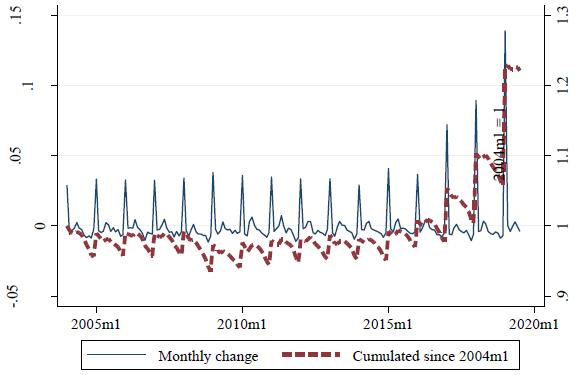
Source: Calculated with data from CONASAMI and National Consumer Price Index
Figure 2 Monthly and cumulated increase in mínimum wage
Figure 3 shows the distribution of labor income in $100 bins for the second quarter of 2019: it shows frequencies and kernel densities, and with vertical lines the MW in 2019 and the inflation adjusted 2005 MW. As a visual aid, in this and the following graphs, the sample is truncated at $40,000 (approximately US$2,000 in 2019). Wage distributions peak above MW, and there are spikes for men and women at the MW. Visually, the vertical lines marking the 2005 and 2019 MW are not very different.

Source: authors calculations using INEGI (2019) Note: vertical lines show MW in 2005 and 2009
Figure 3 Distribution of labor income by grouped bins tq(2019q2)
Minimum salaries are regulated federally. There were three areas before December 2012; two areas between December 2012 and September 2015, and only one between October 2015 and December 2018. Starting in January 2019, a higher wage area was defined, comprising municipalities near the border with the United States.
Using the sample of workers with positive incomes, Table 1 provides a general description of the working population ages 15 to 54 in relation to MW. The columns divide the population among those earning below the MW, up to $300 above, between $300 and $599 above, or $600 or more above. It may be noted there is not an accumulation of individuals right at or nearly above the MW. Women and youths often earn below the MW. Considering those working 20 hours or more per week does not reduce the fraction below the MW in an important way. On the other hand, working for a medium to large employer (including the public sector) does: only 5.5% earn less than the minimum, and 90.1% are $600 or more above the MW (roughly, $600 is 20% of the MW) Rural and low-education workers also earn below MW more often.
Table 1 Distribution of workers by earnings in relation to minimum wage, second quarter of 2019
Earnings difference relative to MW | ||||
Below MW | MW-$299 | $300-$599 | $600+ | |
Age | ||||
15-18 | 37.3 | 3.1 | 7.9 | 51.8 |
19-22 | 22.3 | 3.3 | 7.1 | 67.3 |
23-54 | 16.8 | 2.5 | 3.6 | 77.2 |
Other characteristics | ||||
Women | 26.8 | 3 | 5.2 | 65 |
No secondary education | 26.2 | 3.5 | 4.5 | 65.8 |
Rural | 28.1 | 3.9 | 5 | 63 |
Works 20 hours+ | 22 | 3.4 | 8 | 66.5 |
Medium to large employer | 5.5 | 2 | 2.3 | 90.1 |
Medium to large employer and woman | 7.4 | 2.6 | 3.4 | 86.5 |
Small or no employer | 26.8 | 3 | 5.2 | 65 |
Source: calculations using INEGI (2019, second quarter).
There is important variation in MW coverage across the states. As pointed out by Card and Krueger (1995), having a national wage policy applied to distinct local labor markets can be helpful to identify the effect of increases in MW on employment and earnings. Table 2 shows that the percentage of workers earning below the minimum in the second quarter of 2019 went from 6.9 in Nuevo Leon (which borders with Texas), to 41.1 in Chiapas (north of Guatemala). The pattern cannot be fully summarized in North-South, industrial-rural and other dichotomies. Yet, as an approximation, southern, less industrialized states have higher shares of workers earning below MW.
Table 2 Distribution of workers by earnings in relation to minimum wage by state
Earnings difference relative to MW | ||||
Below MW | MW-$300 | $300-$599 | $600+ | |
Aguascalientes | 9.6 | 0.7 | 3.7 | 86 |
Baja California | 23.1 | 5.9 | 0.7 | 70.2 |
Baja California Sur | 8.9 | 0.7 | 2.2 | 88.3 |
Campeche | 20.6 | 1.9 | 5.5 | 72 |
Coahuila | 11.6 | 1.9 | 3.2 | 83.3 |
Colima | 14.1 | 1.6 | 3 | 81.2 |
Chiapas | 41.4 | 3.5 | 5.1 | 50 |
Chihuahua | 18.1 | 4.2 | 1.6 | 76.2 |
CDMX | 13.7 | 1.2 | 3.7 | 81.3 |
Durango | 13.2 | 1.4 | 4.2 | 81.2 |
Guanajuato | 13.0 | 1.1 | 3.8 | 82.1 |
Guerrero | 26.9 | 2.6 | 4.9 | 65.7 |
Hidalgo | 26.2 | 2.3 | 4.9 | 66.6 |
Jalisco | 10.2 | 0.9 | 3.4 | 85.5 |
Mexico | 13.3 | 3.2 | 4.5 | 78.9 |
Michoacan | 16.4 | 2.3 | 5.8 | 75.4 |
Morelos | 22.9 | 5 | 5.1 | 67 |
Nayarit | 16.8 | 1.3 | 3.7 | 78.3 |
Nuevo Leon | 6.9 | 0.5 | 2 | 90.7 |
Oaxaca | 31.0 | 2.6 | 3.8 | 62.6 |
Puebla | 25.2 | 3.1 | 6.4 | 65.3 |
Queretaro | 8.1 | 1.3 | 2.8 | 87.8 |
Quintana Roo | 10.2 | 1.2 | 3.1 | 85.5 |
San Luis Potosí | 18.4 | 3.4 | 4 | 74.2 |
Sinaloa | 11.4 | 1.2 | 3.9 | 83.5 |
Sonora | 19.4 | 3.8 | 2.2 | 74.6 |
Tabasco | 25.0 | 4.1 | 5.4 | 65.5 |
Tamaulipas | 29.7 | 4.9 | 3.6 | 61.8 |
Tlaxcala | 24.4 | 2.6 | 7.8 | 65.1 |
Veracruz | 23.6 | 4.2 | 5.6 | 66.6 |
Yucatan | 20.3 | 2 | 6 | 71.7 |
Zacatecas | 20.5 | 2.8 | 4.1 | 72.6 |
Total | 18.2 | 2.6 | 4.1 | 75.1 |
Source: calculations using INEGI (2019, second quarter).
The MW was stationary during most of the period under study and so was the share of the labor force earning below MW up to 2016. For youths 15 to 18, the percentage earning below the MW increased in 14 points after the new policy, and for women with small or no employer, the increase was 11 points. Income in inflation-adjusted pesos does not have a positive trend since 2005 for any of the groups shown in the table. Table 3 also shows data for prime-aged workers, underlining the difference between men in medium and large firms, and women with small or no employer; among the first very few earn below MW, while among the second one third earned below the minimum in 2019.
The government has increased the MW well above the general growth in wages since 2016. However, there are other tools for the government to support incomes of workers. A main policy up to 2007 was to reduce the tax load on labor; since 2008, the policy reverted, and taxes have increased at all income levels.
Since the late eighties, the tax code includes a subsidy to low-income workers. It was termed “wage subsidy” up to 2007, and “employment subsidy” since then. The income tax table that applies to individuals had 28 steps in 1986, and the rates were between 3.1 and 55%, with no subsidy. By 2007 there were only five steps the top rate was 28% and the wage subsidy was introduced. In 2008 the wage subsidy is substituted by the employment subsidy. Since 2008, the table of subsidies has not been adjusted by inflation, which means that the benefits accrue to lower real incomes every year. Figure 4 shows the marginal tax rates for 1997, 2007 and 2019, calculated using the tables in the Federal Income Tax Law, indexed by the National Consumer Price Index. The left-hand panel shows the schedule for all levels of income. The right-side panel zooms to low income levels: taxes declined from 1997 to 2007 and have rebounded since then. The vertical line indicates a yearly income equivalent to 2 MW. Earners below 2MW paid in 2019 approximately the same taxes as in 1997, and at a level of only $100,000 (approximately 5,000 dollars) taxes were higher than in 1997.
Table 3 Distribution of workers by earnings in relation to minimum wage and other variables
2005 | 2010 | 2015 | 2016 | 2017 | 2018 | 2019 | |
15-18 years | |||||||
Relation to MW | |||||||
<MW | 23.3 | 23.6 | 23.2 | 29.7 | 27.3 | 33.2 | 37.3 |
$0-$299 above | 4.1 | 5.4 | 8.3 | 1.6 | 9 | 0.7 | 3.1 |
$300-$599 above | 3.4 | 3 | 4.3 | 9.1 | 0.6 | 8.7 | 7.9 |
600+ above | 69.2 | 67.9 | 64.2 | 59.6 | 63.1 | 57.4 | 51.8 |
Other variables (means) | |||||||
Schooling (years) | 8.1 | 8.4 | 8.7 | 8.8 | 8.8 | 9.1 | 9.1 |
Income (2019 pesos) | 4070.7 | 3940.8 | 3766.4 | 3873.2 | 3877.5 | 3964.2 | 4109.8 |
Hours-worked per week | 43 | 40 | 39 | 40 | 39 | 38 | 39 |
19-22 years | |||||||
Relation to MW | |||||||
<MW | 10.6 | 9.6 | 10.4 | 12.4 | 11.3 | 13.9 | 16.8 |
$0-$299 above | 1.8 | 2.4 | 3.3 | 1.1 | 4.6 | 0.6 | 2.5 |
$300-$599 above | 1.5 | 2.4 | 2 | 4.5 | 0.5 | 4.6 | 3.6 |
600+ above | 86 | 85.7 | 84.3 | 82 | 83.5 | 80.9 | 77.1 |
Other variables (means) | |||||||
Schooling (years) | 9.3 | 9.6 | 9.8 | 9.9 | 10 | 10.1 | 10.2 |
Income (2019 pesos) | 7795 | 7279 | 6767 | 6856 | 6807 | 6845 | 6867 |
Hours-worked per week | 43.9 | 42.7 | 43 | 43.8 | 42.6 | 43 | 43 |
23-54 years women, small or no employer | |||||||
Relation to MW | |||||||
<MW | 21.9 | 19.3 | 21.7 | 25.1 | 23.2 | 28.7 | 32.5 |
$0-$299 above | 3.6 | 4.1 | 6.3 | 1.6 | 8.2 | 0.8 | 3 |
$300-$599 above | 2.7 | 4.6 | 3.5 | 7.6 | 0.8 | 7.3 | 5.4 |
600+ above | 71.9 | 71.9 | 68.5 | 65.6 | 67.8 | 63.2 | 59.1 |
Other variables (means) | |||||||
Schooling (years) | 8.9 | 9.3 | 9.7 | 9.8 | 9.9 | 10.1 | 10.2 |
Income (2019 pesos) | 5799 | 5451 | 5009 | 5145 | 5122 | 5139 | 5224 |
Hours-worked per week | 37.7 | 36.6 | 36.1 | 36.9 | 35.6 | 35.7 | 36.4 |
23-54 years men, medium or large employer | |||||||
Relation to MW | |||||||
<MW | 1.1 | 0.7 | 0.9 | 0.8 | 0.8 | 1.4 | 3.6 |
$0-$299 above | 0.3 | 0.3 | 0.5 | 0.5 | 0.8 | 0.3 | 1.5 |
$300-$599 above | 0.7 | 0.5 | 0.8 | 0.6 | 0.3 | 1.3 | 1.2 |
600+ above | 97.9 | 98.5 | 97.8 | 98.1 | 98.1 | 97 | 93.7 |
Other variables (means) | |||||||
Schooling (years) | 10.8 | 11.2 | 11.3 | 11.4 | 11.5 | 11.6 | 11.6 |
Income (2019 pesos) | 10257 | 9987 | 9257 | 9406 | 9180 | 9257 | 9267 |
Hours-worked per | 48.3 | 47.2 | 47.2 | 48.9 | 47.2 | 47.6 | 47.6 |
Source: calculations using INEGI (2019, second quarter).
The purpose of tax-credits to low-income workers is to give them a higher after-tax than before-tax income. Figure 5 shows real income levels in 2007 and 2019 before and after taxes (including subsidies). The x-axis measures average tax rates, and the vertical axis measures income. The main message is that the subsidy was larger in 2007 than in 2019. In 2007 it took incomes near $100,000 for the before and after curves to cross, while in 2019 the threshold was at $50,000. Also, the crossing was at a rate of 12% in 2007, while it was at 8% in 2019.

Source: author´s calculacions using tax tables from SHCP (several years) and National Consumer Price Index
Figure 5 Income before and after taxes and subsidies, and average tax rate
While the public debate has centered around the MW, the analysis shows that low income workers have been on the losing side of tax reform. To the extent that workers are motivated to exchange formal for informal jobs, and firms are motivated to employ less but better paid workers, the effectiveness of the MW regulation becomes moot.
II.Methods and data
The changes in the distribution of wages around dates of change in MW are used to measure their impact on employment. The distribution of salaries is partitioned in small peso-intervals ($100 bins), and each bin is associated with a share of the employed in the total population. The assumed causality relation goes from changes in the MW to the distribution of salaries. Focusing on low-wage jobs around MW levels we refine the measurement of the effect of the regulation.
Our approach to the issue follows Cengiz, Dube, Lindt and Zipperer (2019). The distribution of wages is modeled as a function of current, past and future changes in MW at each location. The main hypothesis is that changes in MW are exogenous to the distribution of wages, and thus changes in MW can be modelled as perturbations of the wage distribution. At any given point in time, some workers earn something close to the MW, and an increase forces their employers to take a decision on keeping the contract at the higher wage or firing the worker. This research follows the basic predictions of price theory: MW increase the cost of labor and in competitive segments of the labor market induce lower employment, while in monopsonistic segments employment can increase (Stigler, 1946). The aggregate effect of a small general increase in MW can be positive in search-theoretic models if search frictions extend the monopsonistic power to many employers (Burdett and Mortensen, 1998). In any case, an increase in MW tends to destroy jobs below the new MW and to create jobs above it. These arguments inspire the "bunching" method in Cengiz, Dube, Lindet and Zipperer (2019) that we apply here.
Medium and large employers largely pay wages above the MW, and perhaps the large increases observed since 2016 have a stronger bite on that segment in the short run, but there are relatively few MW workers in those firms.
Many small employers pay below MW, and independent workers cannot force their sources of income to increase their earnings to MW levels. Thus, it is not easy to take a prior expectation on what the results will show. However, Cengiz, Dube, Lindet and Zipperer (2019) argue that when only a small fraction of the workforce is affected by the MW, the study of changes in the distribution of wages near the MW provides an approximation to the wage effects on employment. Additionally, they expect a “ripple effect” of wage increases above the new MW (also known in the literature as spillover effect).
For the Mexican economy, we saw above that many work at below MW, and while there is some bunching at the MW, it seems reasonable to take as initial assumption that the distribution of wages is largely exogenous, and that the MW works mainly affecting the relatively small set of workers near the MW in firms that comply with the regulation. While we follow the cited methodology, there are differences in our application. First, we measure the effect quarterly, and not yearly. Second, they study relatively small local changes that occur infrequently in a large national labor market, while we study a national policy that predictably adjusts at least every year.
To study the effect of MW changes on the distribution of wages, this distribution is partitioned into small bins to measure the change in the number of jobs near below and above the MW. When the increase in MW shifts some jobs to levels at or above the new MW, including a spillover to higher wage levels, the comparison between the observed distribution and a counterfactual constructed with historical behavior provides a measure of “excess jobs”. Similarly, the number of “missing jobs” is the difference between the observed distribution and a counterfactual constructed with the historical distribution of jobs up to a few bins above the new MW. Behind this approach is the assumption that the distribution of wages is stationary, and the MW perturbates it, and the calculations are seen only as a partial effect of the policy. In this context, stationarity means that MW policy can perturbate the wage distribution around the MW, but after several periods the market washes out the perturbation and the MW has no long-term effect. We keep the language of excess and missing jobs for ease in the comparison with other research, but it the signs resulting from calculations can point to positive missing jobs and negative excess jobs.
The benchmark model relates changes in minimum wage to the distribution of employment
by wage, as a ratio of working age population (equation 1). Nst is the
population of working age at date t in state s
(there are 32 states in Mexico). Esjt is employment in bin
j of the wage distribution. The dummy variables
Thus, the left-hand side is the share of bin j in employment.
Coefficients ατκ measure the change in employment throughout the wage
distribution after a change in minimum wage. We set as benchmark a model that allows
for effects to run up to five quarters since adoption and affecting 4 bins below and
17 bins above the MW level. Thus, there are τκ coefficients for
each bin-date pair. For example, when the time frame covers one year before and one
year after, τ = 9, and if κ = 22 bins are defined,
and there are 198 dummy variables. A priori, we do not prefer a specific time frame
or on how far the ripple effects can reach. The terms
The key causality assumption is that
Following Cengiz, et al. (2019), Table 4 summarizes the main estimates to be
developed. The variable
Table 4 Main estimates
Estimate | Description |
|
Excess jobs above MW |
|
Missing jobs below MW |
|
Average after five quarters of excess jobs |
|
Average after five quarters of missing jobs |
|
Percentage change in employment due to MW increase |
|
Share of workers earning below MW in the quarter before change |
%ΔAffected employment =Δe= | Percentage of workers earning below the MW affected by the change |
|
Source: Cengiz, et al. (2019).
The excess jobs above MW (
Figure 6 illustrates the effect expected from a policy to increase the MW. A priori, we do not expect this hypothesis to hold (that is why we measure), but any proposal to increase the MW expects something like the behavior illustrated in this graph. A solid line shows the density of wages before the increase, and a dashed line the distribution after. After the increase (vertical solid line to dashed line), there are less jobs closely below the MW, and more jobs at an above the MW; the difference between the distributions are the missing (Δb) and excess jobs (Δa). The econometric analysis aims to measure the total effect on employment (Δe = Δa + Δb), including the persistence over time and the reach over the distribution of wages. The debate on MW policy hinges on whether the total employment effect is small or near zero.
Thus, the model sets a time frame to evaluate the impact of the policy and relates changes in MW at the state and municipal levels with changes in employment at the state level. Mainly, comparisons are made between employment after the change with employment before. The estimates are difference-in-difference estimates because changes are measured within and between states. We can control for additional economic and social conditions at the state level. Thus, the main assumption associated with this framework is that the changes in MW are not correlated with unobservable state-level factors.
We estimate equation 1 with no additional regressors (X st ), using only the dummy variables for change in MW, and the time and location fixed effects. This estimation is consistent with an assumption of strict exogeneity of changes in MW with respect to the error. For example, if the distribution of wages shifts due to an exogenous factor (e.g. the USA imposes tariffs in an unexpected and temporary way, affecting the distribution of wages), that event does not affect the probability of a MW change today or in the future.
We also estimate equation 1 with additional regressors: age, years of education, sex, dummy variables for having a medium or large employer or the government, and affiliation to social security. Observations are at the bin-state level, so we use averages at that level. If these variables are not correlated with MW policy, they are control variables that reduce the error in the estimation. However, if they are correlated with the dummy variables for MW changes, estimates will show bias in the previous estimation with no additional regressors.
Data and sample
The National Occupation and Employment Survey (ENOE) provides quarterly
information on individuals, starting in 2005 and ending the second quarter of
2019. We use information on employment status and income from work, at the state
level (there are 32 states in Mexico), for persons with positive monetary income
from work and ages 16 to 55. However, to calculate the
To calculate the distribution of wages we use the sample of employed individuals with positive earnings, and the total population is defined for the ages between 15 and 55. Wages are adjusted by the national consumer price index, taking may of 2019 as base period (the mid-point of the second quarter of 2019).
To define the dummy variables I, the wage bins are calculated first using $20 intervals (at 2019 exchange rates, roughly $1 US dollar). We use only observations with earnings between 0.25 and 15 times the 2019 MW (2341 bins touching a maximum of $46,822). It may be noted that ENOE measures monthly income values, and $20 is 0.64% of a 2019 monthly MW. The information on date of change of minimum salaries is monthly and used at the level of municipality. Dummy variables were constructed for each individual observation and quarter. Most changes coincided with the start of a quarter; when minimum salaries were changed at an intermediate point of the quarter, the change was assigned to the next quarter.
The variable Esjt is calculated by assigning a bin number to individuals at each quarter and counting the individuals for each state, quarter and bin; we use the expansion factors (sampling weights) to obtain numbers at the population level. Variable Nst is the sum of the expansion factors for each state and quarter. For the regression model we group bins in $100 intervals (five bins).
The chosen values of the wage and time intervals to perform the estimations are τ
ε {-4,4} and κ ε {-4,17}. This defines 22 wage-bin levels over which effects are
measured, during an interval covering one previous year and one posterior year
to the change. In turn, this results in 198 dummy variables
III.Results
Figure 6 shows the impact of minimum wages on
the wage distribution. It shows the average over the five quarters after the
adoption of the new MW, for 22 wage levels around the MW

Source: estimates explained in Table 4
Y-axis: difference between actual and counterfactual employment count raltive to the pre-treatment total employment.
Bars show change at bin and 95% confidence intervals; solid line shows cumulated impact
Figure 6 Impact of minimum wages on the wage distribution
The right-hand side of Figure 6 adds the additional regressors and shows also a negative impact for bins 0 and 1 (just above the new MW). Bins between minus 4 and 2 (equivalent to minus 13 to plus 6% of MW) show losses in employment (solid line), most of them statistically significant, and leading to a cumulated loss around 1% in employment at those levels. Losses begin to reverse around bin 9 ($900, or 30% above MW) and disappear around bin 15 ($1,500 or around 1.5 times the MW). Thus, there is some support to a story where missing jobs near the MW are compensated by excess jobs above, but statistical significance is an issue.
If increases in MW had an independent impact on earnings, the solid line should not be very different between both panels of Figure 6. The systematically different results between the model with only MW change dummies and the model with all regressors says that there is an omitted variable problem. I tested the model adding each additional regressor separately, but none is individually capable of producing the full change between the graphs. Thus, the change is produced by the mixed effect of age, education, affiliation to social security and having a medium or large employer. I also tested different specifications of the time variable, mainly to separate trend and seasonal effects, but that path produced no significant changes.
To explore the nature of the bias, we can start by applying mechanically the formula for omitted variable bias, assuming that the whole set of additional regressors is moved by one factor. The no-additional regressors specification has a positive bias in the measurement of the impact of MW changes on employment, which means MW changes have a negative correlation with the error. Thus, if that common factor inducing more education, age, working for a large employer or having social security, is positively correlated with MW increases, we obtain the result of upward bias. Alternatively, there seems to be a factor that relates positively with higher education, social security affiliation, employment in medium and large firms or the government, and age, and also promotes higher MW. It can be mentioned that the model with all regressors, the additional regressors all have negative coefficients with very low p-values, except for the sex dummy variable (equal to 1 for males), which has a positive coefficient (table not shown). Thus, there is evidence of common covariation of those variables.
To measure the impact over time of MW changes, Figure 7 shows the average excess and missing jobs the four quarters previous and the five quarters after the change. Recall that the language of missing refers to the possibility that the MW pushes some workers to higher wage levels, which generates a negative change in the distribution of wages below the MW. Similarly, the language of excess jobs relates to the possibility of having relatively many jobs right above the MW.

Source: Estimates explained in Table 4
Y-axis: difference between actual and counterfactual employment count relative to the pre-treatment total employment.
Figure 7 Impact of minimum wages on the missing and excess jobs over time.
In the model with no additional regressors, excess jobs are never significantly different from zero. Missing jobs are statistically significative in a non-homogenous way: (i) in quarter τ = 0, they are negative, supporting the hypothesis that the MW eliminates jobs below the MW; (ii) in quarters τ = -4, 2, 3, they are positive, which implies that MW increases send more workers below the minimum. In the model with all regressors, missing and excess jobs follow the same pattern than in the model with no additional regressors, except for excess jobs in τ = 4, when the measured effect is positive.
The post-change five-quarter summary measurements ∆a and ∆b are both positive; for the United States, Cengiz, Dube, Lindet and Zipperer (2019) found that these quantities have opposite signs, justifying the term excess and missing jobs. For Mexico, excess jobs average 0.4%, and missing jobs average 0.1%, for a net effect ∆e of 0.6%; that is, some job gains after one year, but distributed above and below the MW and not only above, as the policymakers would like to see. However, the total effects are not statistically significative. Alternatively, we read Table 5 using the definitions in Table 4 in the following way: Δaτ measures the excess jobs in period τ for the five bins ($0 to $500) above the new MW, and Δbτ measures the jobs missing in the four bins below (-$400 to $0), and Δa and Δb measure the result over five periods. In summary, there are short lived employment effects, but no consistent, statistically significant evidence of jobs disappearing below the new MW and appearing above. There is no evidence either of job losses. For the period under study, MW policy seems to only reshuffle jobs with no large or permanent effect on employment or the wage distribution.
Table 5 Estimate of average excess and missing jobs Estimates and 95% confidence intervals
No additional regressors | All regressors | |||||
Estimate | Lower | Upper | Estimate | Lower | Upper | |
Excess | ||||||
Δa1 | -0.003 | -0.013 | 0.006 | -0.001 | -0.01 | 0.008 |
Δa2 | 0.007 | -0.001 | 0.014 | 0.005 | -0.003 | 0.012 |
Δa3 | 0.003 | -0.004 | 0.01 | 0.004 | -0.003 | 0.011 |
Δa4 | 0.004 | -0.007 | 0.015 | 0.003 | -0.007 | 0.014 |
Δa5 | 0.008 | -0.001 | 0.016 | 0.009 | 0.001 | 0.017 |
Mising | ||||||
Δb1 | -0.008 | -0.015 | 0.002 | -0.009 | 0.016 | -0.002 |
Δb2 | 0.004 | -0.002 | 0.009 | 0.002 | -0.003 | 0.007 |
Δb3 | 0.006 | -0.001 | 0.010 | 0.006 | 0.001 | 0.01 |
Δb4 | -0.002 | -0.009 | 0.005 | -0.003 | -0.01 | 0.005 |
Δb5 | 0.009 | -0.003 | 0.015 | 0.009 | 0.003 | 0.016 |
Δa | 0.004 | -0.003 | 0.011 | 0.004 | 0.003 | 0.011 |
Δb | 0.002 | -0.002 | 0.007 | 0.001 | 0.004 | 0.006 |
Δe | 0.006 | -0.002 | 0.014 | 0.006 | 0.003 | 0.014 |
Source: Own calculations.
Note: model with date collapsed by quarter, state and bin. Refer to Table 4 for definition of estimated statistics
Elasticities and coverage
A calculation of the elasticity of employment relative to the minimum wage is obtained by dividing the change in employment (Δe in Table 5) by the average change in MW over the 2005-2019 period, which was 2.3%:
However, as was shown in Table 5, the best evidence is that this is not statistically significant.
The percentage of affected employment is defined as the change in employment
divided by share of the workforce earning below the minimum wage the quarter
before treatment (
To calculate the own-wage elasticity of labor demand we calculate also the change in the affected wage. The percent change in the average hourly wage for affected workers is obtained as the wage bill collected by workers earning below the minimum wage to the number of the affected workers. This average is 1.2%. Given that the calculated change in the percent change in affected employment is positive, we obtain an elasticity larger than one in all calculations. However, the interpretation of this as a calculation of the elasticity is questionable because there are large changes in the affected population and effect are not statistically significant.
The left-hand side of Figure 8 shows the ratio of average wages of workers earning below the MW to others, and the relation between their respective wage bills (total wages paid). Between 2005 and 2015, MW relative to average wages were below 20%, and the wage mass of those below MW was between 2 and 3% of the total. The right-hand side shows that 12 to 14% earned below the minimum (i.e., 12-14% of workers earned 2-3% of total wages). With policies to increase the MW substantially, roughly one in five workers earned below the MW in 2019 and their share in the wage mass increased above 6%. The upward trend does not arise after improvement in real wages but is a result of the increase in MW that simply sends more individuals to the statistical bins below MW. On the other hand, there can be true cyclical and historical trends moving real wage distributions; for example, the bottom reached in Figure 8 occurs during the Great Recession.
Conclusions and policy issues
Our evaluation of the employment effects of changes in MW suggest that the net effects on employment are not important (they are small and not statistically significant), but they induce reshuffling of workers above the MW in ways that are not in line with the goals of the policy: they do not shift many workers from lower to higher wage levels. There are employment losses concentrated in workers between minus 5 and plus 8 bins around MW (minus $500 and plus $800; see Figure 6, right side panel), compensated by increases in employment in bins above. Thus, total employment may not have been affected in the past by MW increases, but even the relatively small increases of most of the studied period generate small negative employment effects near the MW. Over time, effect on excess and missing jobs are small and in general statistically not significative (Figure 5). In summary, in the context of the historical discussion of the effect of MW on employment, my conclusion is that for the period under study these effects are negligible.
We conclude that MW increases seem not to have an independent effect on the wage distribution, but they correlate with the distributions of education, employer size, and social security coverage. It is useful to add that the endogeneity of policy that concerns us is not between wages, employment and hours, but between the decision of the government to increase the MW and other variables (social security coverage, education, employer size).
While much of the public policy debate in Mexico has been dominated by the tie-in of inflation targeting and minimum wages, the issues that need to be addressed are wider, among them regionalization and ties to income-tax policies.