1. Introduction
The international oil industry in its beginnings expanded rapidly at the end of World War II, after having discovered the largest of the oil reserves with very low production costs in Venezuela and a group of countries in the Middle East, by a group of American and European companies (Parra, 2005).
The oil sector is currently going through a time of crisis as in all hydrocarbon-producing countries, due the irreversible changes in extraction projects and the unfair supply in its commercialization, which is challenging the world oil industry, and the consequence is the fall in the price of crude oil (Salgado, 2017). In addition to the global oil crisis, Lajous (2008) mentions that the Mexican oil industry faces a critical juncture, this due to the fact that the expansion phase of the crude oil production cycle started in Mexico and ended in 1996.
There has been an increasing need to investigate the oil industry to make proposals to improve them all their processes and generate higher profits. Some studies have focused on the analysis of efficiency using parametric and non-parametric methods in the upstream area, allowing the identification of areas of opportunity to improve them (Grassian, Bahatem, Scott, and Olsen, 2017; Idowu, Iledare, Omowumi, Echendu and Achi, 2019).
It is also presented proposals to measure the efficiency applying Data Envelopment Analysis (DEA) methodology as well as it has also measured the Total Factor Productivity (TFP) in the oil sector through Malmquist index. With this methodology it is possible to disaggregate the change in TFP into technological change and efficiency change, which allows to analyze the behavior that has occurred over time and thus be able to determine what caused the improvements or setbacks in TFP (Chidi and Hyunjung, 2014; Wang, Zhu and Liu, 2019).
Other studies are also presented where authors have analyzed technical and economic efficiency using the DEA methodology in oil and gas sector. In these models they have included economic variables (prices and profits), being able to identify a decrease in efficiency in 2008 due to the drop in prices in world oil markets (Cosme, Marques, and Rodrigues de Almeida, 2017; Oke and Kareem, 2013; Vikas and Basal, 2018; Zhang, Wu., Li, Xu and Song, 2017).
Studies have also been conducted on the oil sector and its relationship to economic growth, examining the role of oil in the world economy. They carried out studies where they analyzed oil revenues as well as the impact of oil on different economic sectors and explained the reasons for the disturbances in oil prices and their relationship with the reduction in economic growth. For this, econometric models such as time series are used where they perform unit root tests, cointegration model, as well as the error correction model (ECM), to analyze the long and short-term models. They have also presented panel data models applying FMOLS and DOLS. The variables normally used for these models are gross domestic product (GDP), oil revenues (OREV), public spending, the attraction of foreign direct investment and foreign exchange reserves (Al-Mawalil, Mohamad and Al-Busaidi, 2016; Difiglio, 2014; Donwa, Mgbame and Ekpulu, 2015; Humbatova and Qadim-Oglu, 2019; Nweze and Edame, 2016).
Following the importance of efficiency analysis studies in oil sector, this research aims to determine the economic efficiency in the main upstream1 oil producing countries in the world -United States, Russia, Canada, China, United Arab Emirates, Kuwait, Brazil, Kazakhstan, Mexico, Angola, Venezuela, Algeria, United Kingdom, Netherlands, France and Spain- in the period 2010-2017. The hypothesis raised is that the main upstream oil producing countries in the world were not economically efficient during 2010-2017 period. To carry out this study, the methodology of Data Enveloped Analysis (DEA) is used, where technical efficiency, allocative efficiency and economic efficiency are calculated.
The importance of this research is that, although oil industry worldwide, and mainly in Mexico has had a series of ups and downs, it continues to be the main source of energy worldwide, and according to projections from the main private and state oil companies will continue to be for at least fifty more years, which is why studying and identifying the efficiency levels of this industry becomes more relevant. In methodological terms, it becomes more important since, to date, no studies have been conducted on economic efficiency of oil industry worldwide through the application of DEA model.
This paper is structured as follows. In the first, there is an introduction; in the next section, a description of the oil industry in the world is presented; in the third, there is the section on materials and methods; in the fourth section, there is the development of the model; in the fifth, the results and discussion are available; and, in the sixth, the conclusions are presented.
2. Oil Industry in the World
Currently, many countries have managed to develop thanks to the oil industry, since this industry has a great influence on their respective economies because it occupies one of the main places in the generation of foreign exchange (OPEC, 2020a). This industry has had both increasing and decreasing behavior throughout history, going through moments of great economic abundance, as well as great crises that have affected many countries, both producers and dependent on this resource (Rabasa, 2013). This due to its own nature exposed to fluctuating conditions and to the economic policies that the producing countries have adopted towards this industry.
Figure 1 shows the behavior of the average price of oil set by the Organization of Petroleum Exporting Countries (OPEC, 2020b) in the period 1960-2019, where it is observed that in the first decade of the period analyzed, the price per barrel remained stable with a value around 1 dollar. However, from the 1970s it begins to show a considerable increase, reaching the highest price in 1980 with an average price of $ 35.52 per barrel.
In the following years, the price began to drop until the 2000s, when the price of oil rose again considerably, reaching a value of $ 94.1 in 2008, to drop the following year with an average value of $ 60.86, due to the financial crisis that occurred worldwide. In the following years, there is an unstable behavior in prices with highs and lows, reaching the maximum price in 2012 with $ 109.45 and closing the year of 2019 with an average of $ 63.92 p / barrel.
Oil gains are not the same for all countries as they influence their operating expenses, production and energy policies. Oil rent is the difference between the value of crude oil production at world prices and total production costs (World Bank, 2020). In figure 2, it is observed the evolution of oil rents worldwide as a percentage of GDP, where for the year 1970 it represented 0.46%, having an increasing behavior in the following years, reaching the peak in 1980 with 4.51%. Subsequently, it shows a considerable drop in successive years with a slight recovery in 2008, again showing a drop in 2009 due mainly to the financial crisis, and for 2017 it closed with 1.08% as a percentage of GDP.
For Mexico, oil has played a determining role in the development of its economy over the years, its public finances, the development and growth of industry, technological development, trade balance, relations with the exterior, etc. In Mexico, 88% of consumed energy throughout the country, comes from hydrocarbons (INEGI, 2018).
Historically, oil revenues have been a fundamental base for the growth and economic development of the country with important contributions to the Gross Domestic Product (GDP). Figure 3 shows the evolution and importance of the contribution of oil revenues to the Mexican budget revenue.
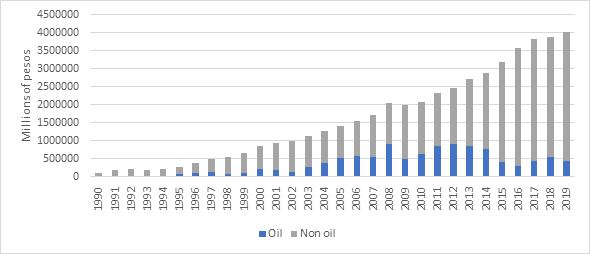
Source: Own elaboration based on SHCP, 2020
Figure 3 Oil and Non-Oil Budget Revenues 1990-2019 (Millions of pesos)
As can be seen in figure 3, oil revenues have played a very important role for Mexican economy. Currently oil revenues have fallen due to production problems and oil prices worldwide, among other factors. For 2020, oil revenues were $431,922.6 millions of pesos, which represents a 10.78% contribution to GDP (SHCP, 2020).
3. Materials and Methods
Stiglitz (1981) mentions that efficiency is considered according to the Pareto Optimum, if there is no feasible alternative allocation of resources that can improve one individual without worsening another. For Coll and Blasco (2006), efficiency is considered as the proper use of resources. Efficiency can be defined as the relationship between the inputs used and outputs obtained.
Farrell (1957), was the first to develop the theoretical and methodological basis of efficiency, disaggregating it into technique and allocative. Although it is important to obtain technical efficiency it is neccesary to take into account price efficiency, “one also needs a measure of the extent to which a firm uses the various factors of production in the best proportions, in view of their prices” (Farrell, 1957, p. 254). In the measurement of technical efficiency, inputs and outputs of the different decision-making units (DMUs) are used and in the case of allocative efficiency, costs or prices are required according to the orientation. The economic or global efficiency is obtained by the calculations made of the technical and allocative efficiency
3.1 Concept of technical efficiency
The first formal definition of technical efficiency said that “a vector composed of inputs and outputs will be technically efficient if it is technologically impossible to increase any output or reduce any input, without simultaneously reducing another output or increase another input” (Koopmans, 1951, p. 460). Likewise, Debreu (1951) and Shephard (1953) contributed in the measurement of technical efficiency although with different orientation (output and input, respectively). Subsequently, Farrell in 1957, made a great contribution where he proposed to visualize efficiency from a real and not an ideal perspective, where each firm or productive unit is evaluated in relation to others taken from a homogeneous representative group. In this way, the measurement of efficiency will be relative and not absolute, where the achieved value of efficiency for a given firm corresponds to an expression of the deviation observed with respect to those considered efficient.
The technical efficiency measures inspired by the proposals of Farrell (1957) and Debreu (1951) are radial. The radial nature alludes to the fact that they measure the maximum equiproportional reduction of all the factors that would be compatible with the same production level or, alternatively, the greater equiproportional increase in the products that could be obtained using the factors in the same quantity. They contemplate increases in the products or reductions in the factors, but all of them in the same proportion, so they are invariant when faced with changes in the units of measurement.
Later Charnes, Cooper and Rhodes (1978) continued studies of Farrell (1957); they used linear programming under constant returns to scale (CRS), which mentions that when a change is made in the input levels, a change is also generated in the same proportion in the outputs. The model formula with constant returns, input orientation in its enveloping form is as follows (Zhu and Cook, 2007):
Where
Banker, Charnes, and Cooper (1984) modify the assumption of constant returns to scale considered in the CRS model and develop the model with variable returns to scale (VRS), in which they included a restriction. This is because there are various factors such as restrictions on access to financing sources, imperfect competition and markets, etc., and this causes that the units does not work at an optimal scale. In this way the original CRS model is modified and the following restriction is added to the linear programming formula: N1´λ = 1. It is presented the model of variable returns to scale with input orientation:
The evaluated unit will be classified as efficient, according to the Pareto-Koopmans definition, if and only if in the optimal solution
3.2 Concept of allocative efficiency
For Gravelle and Rees (1981), allocative efficiency is understood as price signals must be economically efficient, that is, they must approach an optimal Pareto allocation. They consider that exist three basic conditions must be met to achieve allocation efficiency:
Economic efficiency. It implies technological efficiency and uses a combination of factors that reduce costs.
Consumer efficiency. This situation happens when consumers fail to improve by redistributing their budgets.
Equality of marginal cost. It is the cost that is generated after producing an additional unit of product.
Marginal social benefit. It refers to the value of the benefit of an additional unit of consumption, which includes external benefits.
Yarad (1990) mentions that allocative efficiency is the minimum monetary expenditure possible to be applied to all the inputs used according to their prices to produce a specific good.
González-Páramo (1995) mentions that for there to be allocative or pricing efficiency, a company must minimize its costs or maximize its profits.
It is considered that there is allocative efficiency when a company has managed to work optimally in its production, using a combination of factors that allows it to minimize the costs generated to produce a quantity of specific goods (Bosch, Navarro and Giovagnoli, 1999).
Inspired by the works of Debreu and Koopmans, Farrell (1957) obtains a measure of total efficiency, made up of two elements: technical efficiency and allocative efficiency. This author considers a firm that uses two factors of production (input) to produce a single product (output), under conditions of constant returns to scale. The assumption of constant returns to scale allows all relevant information to be presented in one isoquant. This restriction is later abandoned, when working an alternative model where it measures efficiency under conditions of non-constant returns to scale (Farrell and Fieldhouse, 1962).
In figure 1, point P represents the inputs of the two factors per unit of product that the company needs. The isoquant SS 'represents the possible combinations of the two factors that the most efficient company could require to produce a unit of product (Farrell, 1957).
Point Q represents an efficient company that uses both factors in the same proportion as company P. The OQ / OP ratio defines the technical efficiency of company P (Farrell, 1957). In addition, a measure is needed to explain when a company uses the factors of production in the best proportions, according to its prices (the AA´ isocost line). The OR / OQ ratio measures allocative efficiency and as such refers to the appropriate (or inappropriate) selection of the input mix. The economic or global efficiency is the product of allocative efficiency and technical efficiency (Farrell, 1957).
Given that the efficient production function will be represented by isoquants, Farrell's problem is to estimate the isoquant of the most efficient company. This author estimates it by introducing a line (like the one shown in figure 4), which wraps around the points located on the production plane. It assumes that the efficient isoquant is convex to the origin and that, if in practice it is possible to reach two of those points, it is also possible to obtain a point that represents the weighted average of two of the most efficient firms; the weight of each point is determined in such a way that the desired factor ratio is obtained.
Allocative efficiency can have different modalities; when you have the inputs cost, allocative cost efficiency can be calculated. When you have the income of the outputs, you can calculate the revenue efficiency; and when you have both: inputs costs and output income, then you can get profit efficiency. The allocative efficiency of the outputs is realized first calculating the technical efficiency, then the output prices are incorporated and the income efficiency is calculated (Thanassoulis, 2001).
The income function R (x, p) represents the maximum income that can be obtained from the factors x = (x 1 …x n ), taking into account the prices p=(p 1, …,p m ) at which the products are sold y = (y 1 …y m ).
It is required to calculate R j0 ,, which maximizes income, taking into account the prices P rj of the outputs y rj (r=1,…,s) of each DMU j 0 given a level of inputs x ij (r=1,…,m) and is obtained through of the following model (Ghansoli’s, 2001):
where:
j = dmu,
X ij = inputs,
Y rj = outputs,
P rj = outputs prices.
In the last step, allocative efficiency is calculated as the ratio of income efficiency (IE) and technical efficiency (TE):
The maximization of income requires that production be carried out with technical efficiency, that is, that companies achieve the highest achievable production with the factors used, but it is also necessary that the final combination of production obtained is that recommended by the respective prices. For sale, in order to get the highest income; in this case, we speak of allocative efficiency in production. Economic efficiency or Global efficiency is the product of technical efficiency and allocative efficiency (Farrell, 1957).
3.3 Global efficiency or economic efficiency
Global efficiency, also known as economic efficiency, is the line that goes from the origin to the point that represents the analyzed unit. It is obtained by the quotient between the length of the line that goes from the origin to the projected point on the efficient isocost line and the length of the line that goes from the origin to the point that represents the considered unit (Coll and Blasco, 2006). Farrell (1957) decomposed the global efficiency in the following way (see figure 1):
4. Model Development
It is proposed to develop a DEA model of technical efficiency with variable returns to scale (VRS) and output orientation, since it is intended to maximize outputs. Subsequently, the calculation of the allocative efficiency is carried out, where a model based on the prices of the outputs is considered, since the objective is to maximize income. Finally, once the technical efficiency and the allocative efficiency have been obtained, the global or economic efficiency is calculated during the period 2010-2017.
To develop the model, the main countries in terms of oil production in 2017 (OPEC, 2018) were selected as decision-making units (DMUs), obtaining the final sample based on the availability of data from those countries.
Therefore, the countries considered as DMU's of the model are the following: United States, Russia, Canada, China, United Arab Emirates, Kuwait, Brazil, Kazakhstan, Mexico, Angola, Venezuela, Algeria, United Kingdom, Netherlands, France and Spain
For the selection of variables, a review of the literature was carried out first, once the variables most used in this sector had been identified, a statistical analysis of Pearson's Correlation was carried out. The purpose of this study is to identify the correlation of the variables. Since for the use of DEA methodology, it is necessary to corroborate that the correlation between the inputs is low and on the opposite side it must be verified that the correlation between inputs and outputs is high.
Once the calculations shown in Table 1, it can be observed that inputs: oil reserves, labor and platforms are highly correlated with the output: barrels produced, specifically oil reserves input were the showed a higher correlation with 0.883, followed by labor with 0.876 and finally platforms with 0.667. On the other hand, it is also observed that the correlation between the inputs is low. With these results, it is verified that the variables used are adequate for the model developed in this investigation.
Table 1 Pearson Correlation
Input OilReserv | Input Labor | Input Platforms | Output Production | ||
---|---|---|---|---|---|
OilReserv | Pearson correlation | 1 | .109 | .073 | .883** |
Sig. (bilateral) | .689 | .788 | .001 | ||
N | 16 | 16 | 16 | 16 | |
Labor | Pearson correlation | .109 | 1 | .536** | .876** |
Sig. (bilateral) | .689 | .000 | .004 | ||
N | 16 | 16 | 16 | 16 | |
Platforms | Pearson correlation | .073 | .536** | 1 | .667** |
Sig. (bilateral) | .788 | .000 | .005 | ||
N | 16 | 16 | 16 | 16 | |
Production | Pearson correlation | .883** | .876** | .667** | 1 |
Sig. (bilateral) | .001 | .004 | .005 | ||
N | 16 | 16 | 16 | 16 |
**. The correlation is significant at the 0.01 level (2 tails).
Source: Own elaboration based on calculations made with SPSS software.
The inputs and outputs that are used for the economic efficiency model are:
5. Results and Discussion
It can be seen in Table 2 that, for the 16 countries studied, technical efficiency reached the highest values throughout the study period with an average of 0.842. While allocative efficiency obtained a lower value, its average for the years reviewed was 0.246. The behavior of allocative efficiency can be mainly explained by the high sensitivity of countries to oil price volatility. These results consequently affect economic efficiency, where the average for the years reviewed was 0.207, which shows that the maximum income is not being obtained based on the resources used and barrels produced.
It is the year 2014, where there are the highest levels of technical efficiency, the average of all the countries studied for this year was 0.881. In the case of allocative efficiency, its highest level was found in 2010 with an average efficiency of 0.300. Regarding economic efficiency, as with technical efficiency, it was in 2014 when the highest level was reached with 0.254.
Table 2 Technical, Allocative and Economic Efficiency of the Upstream Oil Industry, 2010-2017
Year | technical efficiency | Allocative efficiency | Economic efficiency |
---|---|---|---|
2010 | 0.826 | 0.300 | 0.248 |
2011 | 0.846 | 0.214 | 0.181 |
2012 | 0.845 | 0.231 | 0.195 |
2013 | 0.838 | 0.264 | 0.221 |
2014 | 0.881 | 0.289 | 0.254 |
2015 | 0.864 | 0.202 | 0.175 |
2016 | 0.832 | 0.214 | 0.178 |
2017 | 0.805 | 0.257 | 0.207 |
Average | 0.842 | 0.246 | 0.207 |
Source: Own elaboration based on the calculations made with DEA methodology.
Considering the behavior that each of the countries had during the study period in technical, allocative and economic efficiencies, it is observed in table 3, that it was Russia that obtained the highest values in terms of economic efficiency with 0.974. This is explained by its being one of the main oil and gas producing and exporting countries in the world, which contributes significantly to that country's GDP (OPEC, 2018).
Russian oil and gas companies are a very strong sector that suffered more of a half of the country's profits (Simola and Solanko, 2017). The next country that reached the highest levels of economic efficiency was the United States with 0.767, which is understandable since this country is the main oil producer (OPEC, 2019). Currently, the United States oil industry is one of the most important in the world, being the most relevant and important companies in the market for its level of production and income, Exxon Mobil, Chevron and ConocoPhillips (World Energy Trade, 2019).
The country with the lowest level of economic efficiency was France with 0.036, which is mainly explained by having very low incomes based on the inputs used.
Table 3 Technical, Allocative and Economic Efficiency of the Main Upstream Oil Countries, 2010-2017
Country | Technical efficiency | Allocative efficiency | Economic efficiency |
---|---|---|---|
Russia | 1 | 0.974 | 0.974 |
United States | 1 | 0.767 | 0.767 |
Angola | 1 | 0.228 | 0.228 |
Mexico | 1 | 0.217 | 0.217 |
Brazil | 0.942 | 0.202 | 0.190 |
China | 0.694 | 0.263 | 0.182 |
Spain | 1 | 0.172 | 0.172 |
United Arab Emirates | 1 | 0.163 | 0.163 |
Kuwait | 0.939 | 0.160 | 0.150 |
Venezuela | 0.449 | 0.268 | 0.121 |
United Kingdom | 1 | 0.079 | 0.079 |
Algeria | 0.524 | 0.149 | 0.078 |
Canada | 0.965 | 0.059 | 0.057 |
Kazakhstan | 0.444 | 0.107 | 0.047 |
Neatherlands | 0.499 | 0.080 | 0.040 |
France | 1 | 0.036 | 0.036 |
Source: Own elaboration based on the calculations made with DEA methodology.
The allocative efficiency presents a behavior similar to the economic efficiency for all the countries and for the entire period under analysis Moreover, it is the allocative or price efficiency that determines the evolution of economic efficiency. In both indicators there are two groups of countries, in the first with high levels of efficiency are Russia and the United States, the first is very close to being efficient with a 0.974. In the second group, there are the rest of the countries with very low levels in both allocative efficiency and economic efficiency. Even in this last group of countries, in a very delicate situation, the United Kingdom, Algeria, Canada, Kazakhstan, the Netherlands and France stand out.
Regarding technical efficiency, among the countries that were efficient, that is, those that obtained a value of 1, there are Russia, the United States, Angola, Mexico, Spain, the United Arab Emirates, United Kingdom and France. These countries stood out for their capacity in the processes of discovering oil wells, in drilling and production of crude oil, and in the use of skilled labor (OPEC, 2018).
Among the countries that were not technically efficient for any of the years studied, there are, on the one hand, Brazil, Kuwait and Canada with high levels of efficiency - very close to 1- but without being efficient. While on the other hand, there are Venezuela, Kazakhstan and the Netherlands with rather low levels of technical efficiency; that is, they do not use their resources optimally. In the case of Venezuela, its inefficiency is largely explained by the country's internal and external economic problems in recent years, especially with the United States, which is its main buyer of oil.
In particular, Mexico is technically efficient throughout the study period; that is, it has carried out the production and exploration processes, optimizing its resources adequately. However, when it comes to allocative and economic efficiencies, the situation is the opposite, largely because income was not maximized. According to World Bank figures (2020), Mexico's oil profitability has had a decreasing trend and the volatility of oil prices affects it significantly.
6. Conclusions
The study presents the analysis of the technical, allocative and economic efficiencies of the main oil producing countries worldwide during the period 2010-2017. To do this, three models were developed, one for each type of efficiency, implementing the DEA methodology with variable returns to scale -VRS- and output orientation.
For the selection of variables in obtaining the efficiencies under study, the literature review was carried out at first, and Pearson correlation was applied at a second stage to verify that the inputs had a high correlation with respect to the output. The variables to work in the model were then as inputs: oil reserves (thousands of barrels per day), labor (number of employees), active platforms (number of platforms) and as output: barrels of oil produced (thousands of barrels per day). In obtaining allocative efficiency, the output price was the average price per barrel produced (dollars per barrel).
In the selection of the model DMUs, the main oil producing countries for the year 2017 were considered according to OPEC (2018), as well as the availability of information. The countries that make up the DMUs were then: United States, Russia, Canada, China, United Arab Emirates, Kuwait, Brazil, Kazakhstan, Mexico, Angola, Venezuela, Algeria, United Kingdom, the Netherlands, France and Spain.
The technical efficiency that the countries achieved on average was 0.842. Russia, the United States, Angola, Mexico, Spain, United Arab Emirates, United Kingdom and France stand out as having technical efficiency - with a value of 1- throughout the study period. On the opposite side, there are Venezuela and Kazakhstan with average values throughout the period of 0.449 and 0.444 respectively.
As regards allocative efficiency, it behaves similarly to economic efficiency for all countries and, for the entire period of analysis, moreover, it is allocative or price efficiency that determines the evolution of economic efficiency. In the results presented by these two efficiencies, it is possible to locate two groups of countries, in the first, with high levels of efficiency, are Russia and the United States, the first being very close to being efficient with a 0.974. In the second group, there are the rest of the countries with very low levels in both allocative efficiency and economic efficiency. Even in this last group of countries, in a very delicate situation, the United Kingdom, Algeria, Canada, Kazakhstan, the Netherlands and France stand out.
The economic or global efficiency reached an average in the entire period of 0.207. It is Russia that obtained the highest values in this efficiency with 0.974. This is explained by its being one of the main oil and gas producing and exporting countries in the world. The next country to achieve the highest levels of economic efficiency was the United States with 0.767. The United States oil industry is one of the most important in the world, being the most relevant and important companies in the market for its level of production and income, Exxon mobil, Chevron and ConocoPhillips (World Energy Trade, 2019). While France is 0.036, the country with the lowest level of economic efficiency.
In the case of Mexico, it was technically efficient - it had values of 1- throughout the period under analysis, that is, it carried out its production and exploration processes, optimizing its resources adequately. However, with values of 0.217 in both allocative and economic efficiencies, the situation presented is the opposite, largely due to the fact that incomes were not maximized. According to World Bank figures (2020), Mexico's oil profitability has had a decreasing trend and the volatility of oil prices affects it significantly.
The hypothesis proposed is fulfilled, since the main oil countries of the world were not economically efficient because they did not maximize their income based on the combination of inputs they used and the oil production they had.
Finally, the development of this type of study is important, insofar as it allows identifying the deficiencies of the oil sector, which can contribute to the design of energy policies, especially in those countries that present low levels of efficiency, call these technical efficiency, allocative efficiency and economic efficiency.