Servicios Personalizados
Revista
Articulo
Indicadores
-
Citado por SciELO
-
Accesos
Links relacionados
-
Similares en SciELO
Compartir
Problemas del desarrollo
versión impresa ISSN 0301-7036
Prob. Des vol.52 no.205 Ciudad de México abr./jun. 2021 Epub 23-Ago-2021
https://doi.org/10.22201/iiec.20078951e.2021.205.69710
Articles
Innovation and regional economic growth: evidence from Mexico
aUniversidad Autónoma de Coahuila, Mexico. Email address: * vicentegerman@uadec.edu.mx, ** mauro.soto.r@gmail.com, *** luis.gutierrez@uadec.edu.mx.
This article explores the hypothesis that innovation is an important driver of growth, using an interregional dynamic panel model that controls for endogeneity and captures cumulative effects. In Mexico, the evidence based on regional criteria (geography, income, and innovation stimulus intensity) suggests that innovation has positive effects on growth, as has been theoretically hypothesized, but with gradual reductions from 2008 and significant variations by region and sector. Furthermore, public support for innovation produced different effects between areas with varying degrees of economic development, with greater elasticity in less-economically developed areas. This reality could facilitate the reduction of regional inequalities. Support for innovation should prioritize intensive high-tech projects, include more diverse economic agents, and have a regional perspective.
Keywords: Mexico; innovation; economic growth; economic regions; sectors; dynamic panel model
En el presente texto se aborda la hipótesis respecto a que la innovación es importante para el crecimiento desde un modelo de panel dinámico interregional que controla endogeneidad y capta efectos acumulados. En México, la evidencia basada en tres criterios de región (geográfico, ingresos e intensidad de estímulos a la innovación) señala efectos positivos, como sugiere la teoría, pero con reducciones graduales desde 2008 y variaciones significativas por región y sector. Además, el apoyo público a la innovación impactó de diferentes maneras entre regiones económicamente más y menos avanzadas, con mayor elasticidad en estas últimas, lo que podría favorecer la reducción de desigualdades regionales. Los apoyos en innovación deben priorizar proyectos intensivos en alta tecnología, ser más incluyentes de los diversos agentes económicos y con perspectiva regional.
Palabras clave: México; innovación; crecimiento económico; regiones económicas; sectores; modelo de panel dinámico
Clasificación JEL: O31; O38; O47; P48
1. INTRODUCTION
Research on innovation and economic growth has received much interest from academic circles in recent deades. The relationship between them is not accidental; it depends on factors and decisions that interact for extended periods to shape economic development. The importance of innovation in contemporary societies lies in the fact that innovation constitutes a global strategy to increase productivity, which in turn raises the level of welfare. Some theories see this association as an evolutionary process through which economies somehow pass (evolutionary theories); others emphasize forces that are exogenous and endogenous to the economic system that can be influenced by public policies (classical and neoclassical growth theories). There are also the arguments of spatial agglomeration forces (new economic geography) that modify this relationship, especially at subnational levels.
The consolidation of a creative society system transcended additional approaches which privileged economic aspects such as institutional factors, political factors, the legal framework, and agglomeration forces (Aghion et al., 2015), while the accumulation of productive factors combines to foster long-term sustained growth, given the needs of an ever-increasing population. These productive factors are spillover effects, human capital, infrastructure spending, investment in research and development (R&D), and, finally, innovation and technological change (Romer, 1986; Lucas, 1988; Barro, 1990; Sala-i-Martin, 2000). Within this dynamic, it is crucial for innovation processes to compensate for the diminishing returns of productive factors, expand the production frontier, and avoid the interruption of growth.
In the current context, innovation is vital to an economy (Lucas, 1988; Romer, 1990; Grossman and Helpman, 1991; Aghion and Howitt, 1992), as it allows the production frontier to shift, while generating incentives for the accumulation of physical capital (Romer, 1990). In recent decades, countries that observed higher levels of innovation and growth rates were also those that invested more resources in R&D (Organization for Economic Co-operation and Development [OECD], 2015; Ulku, 2004; Hasan and Tucci, 2010; Agénor and Neanidis, 2015), leading to widening differences in output per capita, technology, innovation capacity, and well-being levels (Fagerberg et al., 2010; Yanhui et al., 2015).
In analyses of the Mexican economy’s innovation and growth, there is a positive relationship. At the level of federal entities, the findings of Mendoza et al. (2008) and Beltrán-Morales et al. (2018) are supported by panel data, while Guzmán and Gómez (2010) and Guzmánet al. (2012) study the long-term relationship between patents and output, concluding that cointegration exists. For their part, Mungaray et al. (2015) and Ríos-Flores and Ocegueda (2018) address an index of innovative capacity in a dynamic panel. Mexico’s economic growth over the last 30 years has been discouraging. In the 1990s, growth was below 1.6% on average per year; in the 2000s it was only 0.12%; while from 2010 to 2017 it stood at 1.5%. In 2019 it fell by -0.3% and forecasts for 2020 announced an even greater decline.1
What role did innovation and research spending play in this situation? The data show that innovation yields (0.67 applications per 100,000 inhabitants) are very low in relation, for example, to the average of OECD member countries (45 applications per 100,000 inhabitants). Furthermore, the country concentrates regional asymmetries in almost every economic and social variable. In innovation alone, Mexico City averages just over three patents per 100,000 inhabitants, while the state of Guerrero has 0.04 patents per 100,000 inhabitants.2
This article examines the innovation-growth relationship using an interregional dynamic panel data model estimated with the GMM-System methodology proposed by Arellano and Bond (1991 and 1998) and Blundell and Bond (1998). The panel is integrated by 32 cross-sectional observations (entities of the country) and 24 temporal observations, corresponding to annual data from 1994-2017. The Arellano-Bond method allows us to select instrumental variables that improve the precision of the estimators, reduce the endogeneity problem, and control for serial correlation.
The Mexican regional context poses some interesting questions, such as: how important is innovation for regional growth? Will public policy designed to support innovation (Innovation Stimulus Program [Programa de Estímulos a la Innovación,PEI]) have any influence? Do sectoral differences affect the innovation-growth relationship? Will there be substantial differences between sectors and regions of the country? The hypothesis is that innovation, measured via the accumulation of patents, has a positive impact on the level of per capita income in the different states, and that public incentive programs reinforce this association.
This article contributes to the discussion of the innovation-growth issue in several ways. First, by using regionalization criteria based on the PEI initiative, it is possible to evaluate the effectiveness of this public policy.3Second, the relative size of the economic sectors, as a regionalization criterion, makes it possible to understand the influence of sectoral weight on the relationship. Third, the evidence is based on a distributed lags approach that captures cumulative processes of the effects of the variables. Finally, a theoretical model is presented whose estimation equation combines ideas from evolutionary growth theory, classical growth theory, and economic geography.
The findings highlight positive effects, consistent with theoretical predictions. However, these positive effects show a gradual tendency to decrease after the 2008 international crisis, which is a reflection of the multinational component of Mexican patenting, given that this great depression was felt more intensely in the more globalized areas of the economies. It is also found that the innovation stimulus policy led to a reduction in regional inequalities, as the regions classified as South and PEI-Low (generally made up of less economically-developed states) estimate a higher growth-innovation elasticity compared to the Center, North, PEI-Middle, and PEI-High regions, i.e., more economically-advanced regions.
In addition to the introduction, this article consists of six further sections. The second section reviews the literature on the subject; the third section presents the theoretical and methodological framework, while the fourth section describes the data and variables. The fifth section estimates and analyzes the results and, finally, the sixth section offers some conclusions.
2. LITERATURE REVIEW
Two theoretical pillars of the innovation-growth relationship are evolutionary economics and the new economic growth theory (Acs and Varga, 2002; Antonelli, 2017). The former argues that economic growth is impelled by innovations, the product of entrepreneurial investments incentivized by the search for profit. This theory also emphasizes institutional and political-regional aspects, as well as microeconomic elements that impact economic growth, including company behavior and size (Aghion et al., 2015).
For evolutionary theory, the innovation-growth relationship has dynamic implications. It is explained by systemic mechanisms present in the process, but also by the presence of random behaviors that generate or renew some variation in the factors that affect innovation and technological change (Nelson and Winter, 1982; Dosi and Nelson, 1994, among others).
The evolutionary aspect in Aghion and Howitt's (1992) model is based on by Schumpeter’s (1942) concept of "creative destruction." According to this model, economic growth is the result of technological progress, while technological progress, in turn, is the product of competition between firms engaged in innovation research. For Aghion and Howitt (1992), innovation is a vertical process that displaces previous technology and favors new and improved technologies.
The new theory of economic growth, on the other hand, values the most important productive factors (Sala-i-Martin, 2000). Some key elements are the knowledge spillover effects derived from physical investment (Romer, 1986), human capital (Lucas, 1988), public infrastructure capital (Barro, 1990), and R&D expenditure (Romer, 1990).
For Romer (1990) in particular, economic growth is driven by technological change that generates incentives for continuous capital accumulation, motivated by profit maximization decisions. This explains much of the increase in productivity that leads to growth. Romer (1990) is based on a horizontal view of innovation; existing technology does not disappear, but is added to the inventions that are gradually introduced into the market. By incorporating the non-rivalry of ideas, the model enters the realm of increasing returns to scale, thus prioritizing monopolistic competition.
Both Romer (1990) and Aghion and Howitt (1992) consider R&D investment to be the determining factor of innovation and, consequently, of sustained economic growth. Accumulated expenditure and technological improvements increase the stock available for other innovations (Romer, 1990; Stokey, 1995), which generates spillovers that benefit not only those who generate innovation, but also the rest of the productive agents, as posited by Griliches (1990).
Even far from the technological frontier, innovation can drive growth in developing regions and countries (Fagerberg et al., 2010), as well as allowing for a deeper understanding of the innovative options that help emerging economies to grow (Ahlstrom, 2015).
There is abundant recent empirical evidence confirming these hypotheses, both internationally and regionally. Bilbao-Osorio and Rodriguez-Pose (2004) analyze this connection in a sample of EU countries. Innovation, measured as the number of patent applications per million inhabitants, generates positive effects that vary according to the country’s level of development. Ulku (2004) obtained the same finding in two exercises on OECD and non-OECD countries. Using a dynamic panel model estimated by the Arellano-Bond general method of moments (GMM) for the period 1981-1997, Ulku finds that innovation (patent applications) has positive impacts on GDP per capita in both samples. Among the results, Ulku highlights that market size and income level are positively associated with innovation. In a 2007 study, Ulku arrives at similar results when testing the hypothesis on four manufacturing sectors in 17 OECD countries (including Mexico).
Rodríguez-Pose and Crescenzi (2008) find positive elasticities between the growth rate and R&D expenditure in 15 European Union countries and conclude that GDP per capita, as an indicator of the level of regional productivity, is lower the greater the distance from the technological frontier. Hasan and Tucci (2010) point out that countries with higher quality patenting firms, as well as those that have increased patenting, experience higher growth. In Poland, Hungary, and the Czech Republic the effects are also positive, although moderate (Pece et al., 2015), the same as in a sample of 38 countries during 1981-2008 (Agénor and Neanidis, 2015). However, Feki and Mnif (2016) report a negative effect in the short run that offsets and becomes positive over the long term in a set of 35 developing nations.
The authors Kacprzyk and Doryń (2017), find no significant impacts between old and new members of the European Union, but a positive relationship when only the group of new countries is considered, meaning that the less an economy resembles a steady-state-economy, the stronger the relationship. Technological progress is the main trigger for productivity growth in certain Chinese provinces, as noted by Wu et al. (2017) and Zhou and Luo (2018). Investment in innovation can create new markets that are not only the basis for sustained economic growth, but also a way for poorer countries to achieve prosperity (Christensen et al., 2019).
Institutions can also encourage or stifle productive entrepreneurial and innovation activities (Acemoglu and Robinson, 2012; Rodrik et al., 2004, among others), boost market creation (Tomizawa et al., 2019), and improve intellectual property regulation to protect innovative agents (Peng et al., 2017). For Hu (2015), South Korea's shift from technological imitation to innovation was not linear; policies and institutions were required depending on the stage of economic and technological development.
Causality has also been the subject of analysis, as there may be bidirectionality between innovation and growth. Maradana et al. (2017 and 2019) use cointegration techniques and autoregressive models to suggest unidirectional causality in some cases and bidirectional in others. Avila-Lopez et al. (2019) detect bidirectional causality in several Latin American countries. In addition to encouraging innovation, states should also encourage investment that increases the level of per capita income.
In Mexico, the innovation-growth hypothesis has revealed positive relationships, with the direction of causality going from innovation to growth. Gould and Gruben (1995) emphasize positive and market structure-sensitive relationships, as the link tends to be stronger in more open regions with robust intellectual property schemes. The importance of trade integration is also supported in Ríos-Flores and Ocegueda (2018). Mendoza et al. (2008) find positive yet moderate evidence using regional indicators. The connection is also favorable in Torres-Preciado et al. (2014) and is reinforced by the presence of positive innovation externalities (measured from patents). Mungaray et al. (2015) find positive impacts from innovative capacity to income and productivity of exports of high-tech goods. Beltrán-Morales et al. (2018) find a positive relationship between the national patent series (per employee) and output per capita during 1988-1998.
3. THEORETICAL MODEL AND ESTIMATING EQUATION
Theoretical model
In the traditional production function, final output (Y) is represented as the combination of inputs that increases when the marginal contribution of capital (FK) is positive (Solow, 1956):
wheresis the saving rate,Kis capital, andLis labor. Given that constant returns are assumed, the continuous accumulation of capital is necessary to prevent the level of output from declining. Technology (with its innovation and patenting activity) is an essential element for achieving this outcome. Improvements in technology (A) stimulate capital and output accumulation (Romer, 1990). This factor, therefore, must be endogenous:
However, understanding the role of technology has not been straightforward. In some cases, it has been treated as dependent on the stock of capital, through learning and experience, as in Arrow (1962); other authors have represented it as human capital, following Lucas (1988); while for Romer (1986) and his followers the role of technology is constituted from the research efforts of firms to develop knowledge (technology), which then becomes public. For Romer (1990), the problem lies in conceiving of technology as a public good, when in fact it behaves as a partially excludable good, given that it is first exploited as a patent system before becoming a common good.
Nevertheless, there are other factors to be taken into account. For the new economic geography, economic activity is concentrated in certain regions, but not in others (Krugman, 1993 and 1995); although there is also an institutional framework influencing the innovation process (productive efficiency) (Nelson and Winter, 1982). Here, innovation results from the production or transformation of knowledge to create other products and processes, resulting in the creation of evolutionary environments. Understood in this way, future innovation depends on past innovation and creative destruction (Schumpeterian economics).
Assuming separate additive effects on output to relax the assumption of perfect substitutes (Romer, 1990), institutional and spatial elements such as regional concentration are added and, finally, (3) is expressed in per capita terms:
In equation (3),Ecaptures productive efficiency (institutional framework), which changes slowly and is therefore assumed to be constant;C t represents economic concentration (agglomeration processes), which varies over time;A t is an available stock of technology (technological knowledge) with 𝜙 > 0 , wherek t is the stock of capital per capita with γ >1. The part in parentheses can be thought of as productivity which is a function of the institutional framework, agglomeration, and differences in technology. If equation (3) is linearized, the random error term (ε) is added and we define In E= αthen:
Output per capita is a combination of the level of technological progress, the stock of capital per capita, and agglomeration processes. Due to diminishing returns to capital, the dynamic behaviors ofA t andC t are key in the fluctuations of output per capita.
Finally, to analyze sets of economies the model in equation (4) is represented as a panel structure and control variables on specific characteristics are added:
where Χ i,t is a matrix of control variables, β j a vector of coefficients to be estimated and 𝜂 i and 𝜑 t are individual fixed effects.
Methodology
A distributed lags model is defined to test the previous approach. The model also seeks to factor in the fact that the effects of innovation could accumulate indefinitely, although progressively decreasing, and to capture variations between regions and sectors. The standard equation with an unknown number of lags of the explanatory variables is defined as follows:
whereLis the polynomial lag operator. To overcome the condition of an unlimited number of lags, the Koyck criterion is assumed, i.e., the effects of lags fall geometrically (Greene, 2008). Thus, we consider a fixed factor, λ, of successive reductions in previous periods:
Subtracting equation (7) from (6) and rearranging terms:
where
Thus, the model can be extended to the case ofk-variables multiple regression (Greene, 2008):
Two measures of interest are the average value and median of the lags, as they give an idea of the speed at which y responds to a change in x (Greene, 2008):
Average lag:
Finally, expressing equation (9) as a dynamic panel structure:
To solve problems of endogeneity and inconsistency in the estimates, the GMM-System method is used, which contemplates lags of the endogenous variable as instruments, either in levels and/or in differences (Arellano and Bond, 1991 and 1998; Blundell and Bond, 1998). The validity of the instruments is checked using Sargan's overidentification test, while serial non-correlation is verified with the tests of orders 1 and 2 suggested in Arellano and Bond (1998).
Empirical model, variables, and data source
Innovation is measured via the number of patent applications reported by IMPI and standardized per 100 thousand inhabitants (inventiveness coefficient). The dependent variable is GDP per state from the National Institute of Statistics and Geography (El Instituto Nacional de Statistical y Geografía, INEGI) and is measured per person. State population data are obtained from the projections made by the National Population Council (Consejo Nacional de Población, CONAPO). The empirical model includes variables such as physical capital accumulation, agglomeration, and relative size of state economies, as well as region and sector of economic activity dummies. For accumulated capital, we use the industrial capital stock estimated in Germán-Soto (2008). This indicator is attractive given the absence of real investment figures by state, as it is an accumulation of investment at constant prices of the most productive capital-intensive sectors (mining, electricity, gas and water, construction, and manufacturing). Population density per square kilometer is used to measure agglomeration, (state area is obtained from INEGI). More densely populated economies tend to have higher incomes. Authors such as Romer (1990) point out that the model can be extended to include some measure of human capital. In this case, however, the use of human capital indicators is unsatisfactory, due to the collinearity present with agglomeration.
During the period of analysis, population density maintained a 98% correlation with education, measured as average years of schooling. Some authors find that when market size (population density) increases, so does investment in human capital (Duranton and Puga, 2004), or that human capital correlates strongly with population (Sedgley and Elmslie, 2001 and 2004). Therefore, agglomeration, apart from being useful in capturing intrinsic characteristics of regional economies, is also advantageous when inferring the potential role of human capital.4The relative size of state economies is measured by the state to national output ratio.
Once the set of indicators is defined, the final empirical model is:
whereGDPpcis per capita income,INNis the measure of innovation,AGLOis the agglomeration index,SCis the capital stock, andTAMis the relative size of the economies.
Three regionalization criteria are defined to capture the empirical evidence: level of per capita income (high-, middle-, and low-income regions), degree of participation in the innovation stimulus program (PEI-High, PEI-Medium, and PEI-Low regions), and geographic location (three macro-regions: North, Central, and South). The per capita income criterion was defined based on the average of this variable between 2009 and 2017, while for the PEI we measured the intensity with which each federative entity participated in the innovation stimulus program in the same period. In both cases, the idea is to have a classification without the bias caused by the performance of any particular year. In the case of geographic regionalization, the North-Central-South configuration was followed as far as possible. The three classes of regions can be represented in the same regression equation, simultaneously, to form an interregional model:
whereDUM r is the dummy of the rthregion. Equation (12) is estimated in restricted and unrestricted form, the latter assuming differential effects by economic sector (primary, secondary, and tertiary). The definition of equation (12) allows for interregional comparative analysis, a crucial aspect when conducting regional studies.
4. STATISTICAL-DESCRIPTIVE ANALYSIS
The generation of patents by federative entity shows asymmetric behavior and is concentrated in the lower intervals of the distribution (see Figure 1). 65% of cases are located in the range of 1 to 20 applications, while only 4.9% of the total registrations are observed at the higher level, (greater than 100).

Source: compiled by the authors using data from IMPI (2018).
Figure 1 Distribution of patent applications
Meanwhile, there is a positive relationship between patents per 100 thousand inhabitants and GDP per capita in 2017 (the end of the analysis period). The slope, however, is not very steep (see Figure 2). The accentuated asymmetric feature becomes more visible with the analysis of basic statistics (see Table 1). The large distance between maximum and minimum values stands out. The state GDP per capita averages the highest figure in the state of Campeche in 2003 (MXN$1,413 million),5an income similar to that of countries such as Norway and Finland and higher than that of the US.
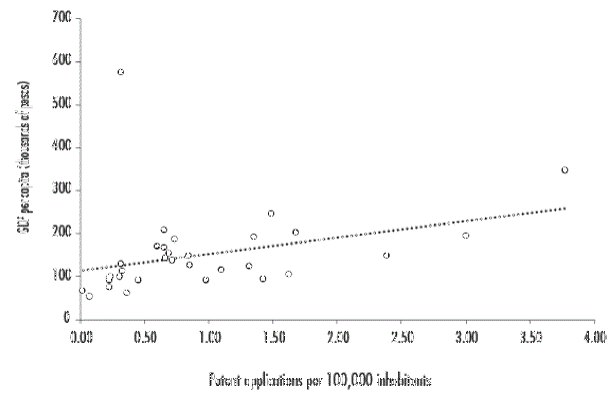
Source: compiled by the authors using data from INEGI (2018) and IMPI (2018).
Figure 2 Relationship between the inventiveness coefficient and GDP per capita, 2017
Table 1 Descriptive statistic of basic variables, 1994-2017
Observations | Average | Standard devation | Minimum value | Maximum value | |
GDP per capita (Y) | 736 | 147 897 | 180 007 | 49 185 | 1 413 430 |
Inventiveness coefficient (INN) | 736 | 5.25 | 7.81 | 0.05 | 66.09 |
Size of economy (TAM) | 736 | 3.13 | 3.22 | 0.51 | 17.89 |
Capital stock per capita (SC) | 736 | 70 278 | 86 985 | 87 094 | 734 545 |
Population density (AGLO) | 736 | 286 | 1 027 | 5 | 5 993 |
Source: compiled by the authors.
However, Campeche's income comes primarily from oil extraction activities, and these monetary resources do not remain in the state. Therefore, a more suitable parameter for comparison is the per capita income of Mexico City, whose maximum value is MXN$345,558 million annually, an amount similar to that of countries such as Barbados, Lithuania, and Slovakia.
The lowest figure in the panel is observed in the state of Oaxaca, in 1995 (MXN$49,185 million), similar to that of countries such as Nicaragua or Ghana.6Population density also shows a large variance. Mexico City has the highest value in 2004, with 5,993 inhabitants per km2; while the state with the lowest density is Baja California Sur, with five inhabitants per km2 in 1994.
Regarding the relative size of state economies, the highest value is found in Mexico City in 1995, with 17.89% of the national economy, while the lowest is attributed to Colima in 2005, with 0.51%. As for the stock of industrial capital per capita, the two main oil-producing entities, Campeche and Tabasco, present the highest levels of the entire period. The minimum value of industrial capital stock belongs to Quintana Roo, in 2017, an entity with little industry, but with a strong tourist sector.
Figures 3, 4, and 5 classify the states of the country based on the previously defined macro regionalization criteria. The concentration of the highest levels of income and resources executed within the PEI is mainly in the north of the country, Mexico City, Jalisco, and Querétaro.

Source: compiled by the authors.
Figure 4 Regionalization by level of resources exercised within the PEI
5. RESULTS
The GMM estimations apply the two-stage approach.7The results are presented in Tables 2, 3, and 4. Table 2 is a non-regionalized analysis. For every 1% increase in the inventiveness coefficient, a 0.063% increase in revenue is generated. The estimate is statistically significant and higher than the estimated relationship for middle-income countries using a comparable innovation and growth variable.
Table 2 Growth-innovation: findings from the absolute sample
Variable | Absolute sample | |
ln(Yt-1) | 0.432 *** | (41.40) |
ln(INN) | 0.063 *** | (17.20) |
ln(SC) | 0.030 *** | (12.00) |
ln(AGLO) | -0.189 *** | (7.15) |
ln(TAM) | 0.667 *** | (27.60) |
2008 crisis dummy | -0.016*** | (17.50) |
Constant | 6.530 *** | (62.50) |
Regression diagnostics | ||
Sargan test | 31.84 | [0.97] |
Serial correlation (order 1) | -4.56 *** | [0.00] |
Serial correlation (order 2) | 0.18 | [0.86] |
Long-term effects | ||
Median of lags | 0.826 | |
Average lag | 0.761 | |
Elasticity of innovation | 0.111 |
Notes: t values in parentheses and p-values in square brackets. The superscript *** indicates significance at 1%.
Source: compiled by the authors.
Pece et al. (2015) highlight values of 0.1% (Poland), 0.5% (Czech Republic), and 0.6% (Hungary). For a sample of 58 countries, Hasan and Tucci (2010) estimate an elasticity of GDP per capita to patents close to 0.04% in high- and low-income economies, while Feki and Mnif (2016) estimate an effect of 0.02% in 35 developing countries.
Ulku (2004) finds that the elasticity between patent applications and GDP per capita for several subsamples of OECD countries ranges from 0.04 to 0.06%; while Kacprzyk and Doryń’s (2017) estimates for the European range from 0.02 to 0.08%, using patent definitions from the EPO system, and from 0.003 to 0.12% for those established using the USPTO.8 Ríos and Castillo (2015), meanwhile, estimate variations ranging from 0.02 (with patents) to 0.04% (inventiveness coefficient) in a pooled sample of developed and developing countries. This first approximation is consistent, both with theory and with several national and international studies that use similar methodologies and variables.
In Mexico, Ríos-Flores and Ocegueda (2018) do not find patents to be significant, but when they test with the inventiveness coefficient they obtain a statistically significant elasticity of 0.03%. When considering the relation in an interregional model, the innovation-growth elasticities fluctuate between 0.03 and 0.06%, between high-, middle-, and low-income regions, values very close to this paper’s findings and which may be comparable when using similar estimation and regionalization techniques. Mungaray et al. (2015) estimate effects that raise GDP per capita by 0.15% for each percent increase in Mexico's innovative capacity, a value higher than that of OECD and European Union countries. Finally, Beltrán-Morales et al. (2018) estimate elasticities between 0.03 and 0.05% during 1998-2008, when using the number of patents or patents relativized by wages and salaries.
The size of the economy and the stock of physical capital also exert positive and significant effects on income. Furthermore, the dummy variable on the 2008 international crisis was negative and significant, so this event has contributed to weakening growth. It should be remembered that innovation in Mexico, as in other developing countries, has a strong multinational component, as this activity is dominated by foreign companies that seek to exploit the increasing returns generated by a new invention. In Mexico, a significant proportion of patents (close to 90%) are of foreign origin; therefore, in the face of a global crisis such as that of 2008, multinational activity is significantly reduced, which is reflected in patenting and, consequently, regional spillovers are negatively affected.
The model does not present over-identification problems (Sargan test), i.e., the conditions of moments have been correctly selected. The Arellano-Bond test holds that in the second order there is no longer serial autocorrelation, which is correct. The median of the lags in Table 2 is 0.87, i.e., 50% of the total change in per capita income is achieved in just under one period. The median lag value is 0.76 and represents a weighted average of the lags. Finally, the long-term innovation multiplier indicates that a sustained 1% increase in the inventiveness coefficient leads to a 0.11% increase in state per capita income. Tables 3 and 4 report the regional and sectoral evidence. The first column of Table 3 corresponds to income-based regionalization criterion. The highest innovation-growth elasticity is observed in the high-income region (0.079%), while the smallest effect is found in the lowest income region (0.038%). This suggests hierarchical effects according to income level.
Table 3 Growth-innovation, results of the restricted inter-regional model
Variable/Region | Income | PEI | Geography | |||
ln(Yt-1) | 0.441*** | (14.60) | 0.404*** | (20.70) | 0.510*** | (23.10) |
ln(INN)*D1 | 0.079*** | (3.53) | 0.022 | (0.87) | 0.048 | (1.37) |
ln(INN)*D2 | 0.049*** | (2.79) | 0.051** | (2.27) | 0.042** | (2.06) |
ln(INN)*D3 | 0.038*** | (3.98) | 0.053*** | (3.65) | 0.06** | (2.34) |
ln(TAM)*D1 | 0.590*** | (4.45) | 1.130*** | (5.14) | 0.577*** | (2.93) |
ln(TAM)*D2 | 0.707*** | (4.13) | 0.806*** | (6.58) | 0.473** | (2.25) |
ln(TAM)*D3 | 0.615*** | (9.77) | 0.427*** | (6.13) | 0.532*** | (6.72) |
ln(SC)*D1 | 0.034 | (1.47) | 0.017 | (0.83) | -0.025 | (-0.83) |
ln(SC)*D2 | -0.023 | (-0.62) | 0.011 | (0.38) | 0.055 | (1.49) |
ln(SC)*D3 | 0.039** | (2.09) | 0.053*** | (3.68) | 0.029 | (1.32) |
ln(AGLO)*D1 | -0.371* | (-1.66) | -0.115 | (-0.41) | -0.197 | (-0.36) |
ln(AGLO)*D2 | -0.080 | (-0.34) | 0.096 | (-0.25) | 0.004 | (0.01) |
ln(AGLO)*D3 | 0.017 | (0.06) | -0.193 | (-0.72) | -0.396 | (-0.87) |
Constant | 6.330*** | (15.60) | 6.120*** | (15.40) | 5.770*** | (11.30) |
Regression diagnosis | ||||||
Sargan test | 31.64 | [0.97] | 31.32 | [0.95] | 31.29 | [0.94] |
Serial correlation (order 1) | -4.66*** | [0.00] | -4.53*** | [0.00] | -4.032*** | [0.00] |
Serial correlation (order 2) | -0.108 | [0.91] | 0.193 | [0.85] | 0.534 | [0.59] |
Long-term effects | ||||||
Median of lags | 0.847 | 0.765 | 1.029 | |||
Average lag | 0.789 | 0.678 | 1.041 | |||
Elasticity of innovation in D1 | 0.141 | n.s. | n.s. | |||
Elasticity of innovation in D2 | 0.088 | 0.086 | 0.086 | |||
Elasticity of innovation in D3 | 0.068 | 0.089 | 0.122 |
Notes: t-values in parentheses and p-values in brakets. n.s. = "not significant". Superscripts ***, ** and * indicate significance at 1, 5 and 10°%, respectively. Dj for j = 1, 2 and 3 are dummies for the categories of each region criterion used in the interregional model and take as follows: D1 = 1 for high income, PEI-High, North; 0 otherwise. D2 = 1 for middle income, PEI-Middle, Central; 0 otherwise. D3 = 1 for low income, PEI-Low, South; 0 otherwise. Source: compiled by the authors.
These findings are similar to those obtained by Hasan and Tucci (2010) at the country level, as discussed above, but more closely resemble those of Ulku (2004) and are within the range estimated by Kacprzyk and Doryń (2017) for countries in the European area. Moreover, as in Ulku (2004), higher-income economies also showed higher elasticities.
The median lags of the regionalized model by income is 0.85, very similar to that estimated for the absolute sample (see Table 2). The long-run multiplier effect is higher in the high-income region, at 0.14%, a value slightly higher than that found for the overall sample.
The results based on PEI regions show that the short-term effect of innovation on per capita income is very similar in the PEI-Middle and PEI-Low regions, slightly higher than 0.05%. Consequently, the long-term multiplier of these regions is also similar: a sustained 1% increase in the inventiveness coefficient will increase the per capita income of the entities in these two regions by 0.09%. The results of this regionalization suggest that the PEI played a decisive role in the effect that innovation has on state income, although it was not significant for the group of entities that exercise more resources within the program; this may be because they possibly show a greater proximity to the equilibrium relationship.
In the results by geographic region, the elasticity between innovation and per capita income is greater in the Southern region (0.06%) than in the Center (0.042%), while in the North, although higher, it was not significant. These short-term differences explain why the long-term effect is also greater in the South (0.12%) than in the Center (0.09%). In itself, the elasticity intensities seem to reflect the condition of diminishing returns, the further away from potential growth, as would be the case in the South, the greater the effect.
The unrestricted hypothesis on sectoral differences (see Table 4) points to non-significant effects of the inventiveness coefficient on per capita income in the relatively larger entities of the primary sector, a result related to the low level of technology that characterizes this sector.
Table 4 Growth-innovation, results of the unrestricted inter-regional model by sector
Variable/Region | Primary | Secondary | Tertiary | |||
ln(Yt-1) | 0.430*** | (20.50) | 0.397*** | (11.00) | 0.404*** | (18.80) |
ln(INN)*D1 | 0.065 | (1.46) | 0.082*** | (4.02) | 0.080** | (2.45) |
ln(INN)*D2 | 0.064** | (2.40) | 0.069* | (1.77) | 0.085** | (2.15) |
ln(INN)*D3 | 0.065** | (2.33) | 0.054*** | (2.62) | 0.037*** | (3.47) |
ln(TAM)*D1 | 0.031 | (1.02) | 0.683*** | (7.98) | -0.002 | (-0.07) |
ln(TAM)*D2 | -0.086 | (-0.25) | 0.847*** | (2.94) | 0.015 | (0.71) |
ln(TAM)*D3 | 0.069 | (1.49) | 0.303* | (1.92) | 0.063* | (1.65) |
ln(SC)*D1 | 0.651*** | (2.70) | 0.070* | (1.72) | 0.875*** | (5.18) |
ln(SC)*D2 | 0.675*** | (7.48) | -0.007 | (-0.21) | 0.667*** | (7.71) |
ln(SC)*D3 | 0.654*** | (12.10) | 0.044* | (1.69) | 0.623*** | (9.72) |
ln(AGLO)*D1 | -0.165 | (-0.28) | -0.393* | (-1.72) | -0.282 | (-0.68) |
ln(AGLO)*D2 | -0.375 | (-0.63) | -0.386 | (-0.54) | -0.562 | (-1.15) |
ln(AGLO)*D3 | -0.224 | (-0.58) | 0.007 | (0.02) | -0.022 | (-0.09) |
Constante | 6.800*** | (13.70) | 7.130*** | (8.53) | 7.210*** | (14.00) |
Regression diagnostics | ||||||
Sargan test | 31.30 | [0.97] | 31.10 | [0.96] | 31.60 | [0.95] |
Serial correlation (order 1) | -4.42*** | [0.00] | -4.53*** | [0.00] | -4.60*** | [0.00] |
Serial correlation (order 2) | 0.36 | [0.72] | 0.19 | [0.85] | 0.21 | [0.83] |
Long-term effects | ||||||
Median of lags | 0.821 | 0.750 | 0.765 | |||
Average lag | 0.754 | 0.658 | 0.678 | |||
Elasticity of innovation in D1 | n.s. | 0.136 | 0.134 | |||
Elasticity of innovation in D2 | 0.112 | 0.114 | 0.143 | |||
Elasticity of innovation in D3 | 0.114 | 0.090 | 0.062 |
Notes: t-values in parentheses and p-values in brakets. n.s. = "not significant". Superscripts ***, ** and * indicate significance at 1, 5 and 10°%, respectively. Definition of regions, see notes in Table 3. Source: compiled by the authors.
The medium-sized and small primary sector entities present very similar short-term effects (0.064 and 0.065, respectively), suggesting that economic growth is less dependent on this group of productive activities. However, entities with larger secondary and tertiary sectors estimate higher innovation effects (around 0.08%) compared to those with smaller proportions (between 0.04 and 0.05%). This same interregional comparative result is maintained in relation to the long-term multiplier, which is between 0.06 and 0.14%.
In general, the results confirm the greater importance of the sector most related to the use of productive capital to innovate, in addition to being consistent with the hypothesis of differentiated effects not only between regions, but also between sectors of economic activity, an aspect that, in the Mexican case, is important to emphasize when designing an appropriate innovation policy (Unger, 2011).
6. CONCLUSIONS
The empirical investigation aligns with the theory and reaffirms the idea that innovation is a factor that stimulates economic growth. The effect of innovation on the per capita income level of entities is very close to that estimated in OECD and middle-income countries.
The effect of innovation on economic growth varied with the presence of public policies such as the PEI. It was greater in economically disadvantaged regions, which suggests that it could also be used to reduce regional inequalities. Growth could be boosted by strengthening programs that seek to promote innovation. Promoting innovation not only has a greater impact on the productivity of regions far away from the technological frontier, but also helps to improve the capacity to assimilate and eventually produce new technologies. In this sense, it is important to take into consideration what is known as the "something better" trap (Ahlstrom, 2015), i.e., investing too many resources in an attempt to surpass the innovations of the most technologically advanced countries, when products and processes can be generated that endogenously improve the productivity of local markets (Ahlstrom, 2015).
The road to an innovative economy can be paved by the creation of new products and processes, or even by encouraging their creation, an area of opportunity presented by the country's economic activities.
The road to an innovative economy can be complex. The results indicate the usefulness of designing public policy instruments that support and give continuity to programs that are already underway with a long-term vision. The effects of innovation tend to be more important in regions with larger secondary and tertiary sectors. The contribution of innovation within more specific economic activities could be investigated, for example, to direct public policies to support innovation with a greater effect on economic growth. It also seems advantageous to concentrate innovation efforts on more technologically intensive industries, in accordance with the categorization that the OECD (2003) has been constructing and updating.
Despite the advantages of the number of patents as an indicator of innovation, including its simplicity and ease of application, as well as the quality of allowing homogeneous comparisons in space and time, it should not be forgotten that the concept of innovation is much broader than simply patents, as it also consists of new processes and organizational designs directly linked to the production of goods and services and that are often difficult to concentrate in a single indicator. Therefore, the patenting parameter used is only an approximation of the actual behavior it is intended to measure. Even with these limitations, it is possible to conclude that the growth-innovation relationship is positive and, moreover, can be enhanced through public policies that take into account the country's regional diversity.
REFERENCES
Acemoglu, D. y Robinson, J. (eds.) (2012). Why nations fail: The origins of power, prosperity and poverty. Crown Books. https://doi.org/10.30541/v51i3pp.276-278. [ Links ]
Acs, Z. y Varga, A. (2002). Geography, endogenous growth, and innovation. International Regional Science Review, 25(1). https://doi.org/10.1177/016001702762039484. [ Links ]
Agénor, P. R. y Neanidis, K. C. (2015). Innovation, public capital, and growth. Journal of Macroeconomics, 44(C). https://doi.org/10.1016/j.jmacro.2015.03.003 [ Links ]
Aghion, P. y Howitt, P. (1992). A model of growth through creative destruction. Econometrica, 60(2). https://doi.org/10.2307/2951599. [ Links ]
______, Akcigit, U. y Howitt, P. (2015). The schumpeterian growth paradigm. Annual Review of Economics, 7(1). https://doi.org/10.1146/annurev-economics-080614-115412 [ Links ]
Ahlstrom, D. (2015). Innovation and growth in emerging economies. En Austrian Council for Research and Technology Development (ed.). Designing the future: Economic, societal, and political dimensions of innovation (pp. 353-387). Echomedia. [ Links ]
Antonelli, C. (2017). Endogenous innovation. The economics of an emergent system property. Edward Elgar Publishing. [ Links ]
Arellano, M. (1993). On the testing of correlates effects with panel data. Journal of Econometrics, 59(1-2 ). https://doi.org/10.1016/0304-4076(93)90040-C [ Links ]
Arellano, M. y Bond, S. (1991). Some tests of specification for panel data: Monte Carlo evidence and an application to employment equations. Review of Economic Studies, 8(2). https://doi.org/10.2307/2297968 [ Links ]
______y Bond, S. (1998). Dynamic panel data estimation using DPD98 for Gauss: A guide for users. CEMFI, December 1998. https://www.cemfi.es/~arellano/#WPapers. [ Links ]
Arrow, K. J. (1962). The economic implications of learning by Doing. The Review of Economic Studies , 29(3). https://doi.org/10.2307/2295952 [ Links ]
Avila-Lopez, L., Chaofeng, L. y Lopez-Leyva, S. (2019). Innovation and growth: Evidence from Latin American countries. Journal of Applied Economics, 22(1). https://doi.org/10.1080/02102412.2019.1610624. [ Links ]
Banco Mundial (2018). Indicadores del desarrollo mundial. https://datos.bancomundial.org/indicador. [ Links ]
Barro, R. (1990). Government spending in a simple model of endogeneous growth. Journal of Political Economy, 98(5).https://www.journals.uchica-go.edu/doi/abs/10.1086/261726. [ Links ]
Beltrán-Morales, L., Almendarez-Hernández, M. y Jefferson, D. (2018). El efecto de la innovación en el desarrollo y crecimiento de México: una aproximación usando las patentes. Problemas del Desarrollo. Revista Latinoamericana de Economía, 195(49 ). https://doi.org/10.22201/iiec.20078951e.2018.195.63191. [ Links ]
Bilbao-Osorio, B. y Rodríguez-Pose, A. (2004). From R&D to innovation and economic growth in the EU. Growth and Change, 35(4). https://doi.org/10.1111/j.1468-2257.2004.00256.x. [ Links ]
Blundell, R. y Bond, S. (1998). Initial conditions and moment restrictions in dynamic panel data models. Journal of Econometrics , 87(1). https://doi.org/10.1016/s0304-4076(98)00009-8 [ Links ]
Christensen, C., Ojomo, E. y Dillon, K. (eds.) (2019). The prosperity paradox: How innovation can lift nations out of poverty. Harper Business. [ Links ]
Dosi, G. y Nelson, R. (1994). An introduction to evolutionary theories in economics. Journal of Evolutionary Economics, 4(3). https://doi.org/10.1007/BF01236366. [ Links ]
Duranton, G. y Puga, D. (2004). Micro-foundations of urban agglomeration economies. En J. V. Henderson y J. F. Thisse (ed.). Handbook of regional and urban economics, vol. 4 (pp. 2063-2117). Elsevier. https://doi.org/10.1016/s1574-0080(04)80005-1 [ Links ]
Fagerberg, J., Srholec, M. y Verspagen, B. (2010). Innovation and economic development. En B. Hall y N. Rosenberg (eds.). Handbook of economics of innovation, vol. 2 (pp. 833-872). Elsevier. https://doi.org/10.1016/s0169-7218(10)02004-6. [ Links ]
Feki, C. y Mnif, S. (2016). Entrepreneurship, technological innovation, and economic growth: Empirical analysis of panel data. Journal of the Knowledge Economy, 7(4). https://doi.org/10.1007/s13132-016-0413-5 . [ Links ]
Germán-Soto, V. (2008). El stock de capital industrial medido a través de la relación inversión-empleo: estimaciones para los estados mexicanos. Ensayos. Revista de Economía, 27(1).http://ensayos.uanl.mx/index.php/ensayos/article/view/105 [ Links ]
Gould, D. y Gruben, W (1995). El papel de los derechos de propiedad intelectual en el crecimiento económico. Economía Mexicana, Nueva Época, 4(2). https://doi.org/10.2307/j.ctv10rr99j.11 [ Links ]
Greene, W. H. (2008). Econometric analysis. Pearson Prentice Hall. (Original publicado en 1990). [ Links ]
Griliches, Z. (1990). Patent statistics as economic indicators: A survey. Journal of Economic Literature, 28(4).https://www.jstor.org/stable/2727442 [ Links ]
Grossman, G. y Helpman, E. (eds.) (1991). Innovation and growth in the global economy. MIT Press. [ Links ]
Guzmán, A. y Gómez, H. (2010). Brechas tecnológicas y procesos de convergencia entre países emergentes e industrializados en la industria biofarmacéutica, 1980-2005. En J. Guillén (coord.). Una década de estudios sobre economía social (pp. 38-65). Juan Pablos. [ Links ]
______, López-Herrera, F. y Venegas Martínez, F. (2012). Un análisis de cointegración entre patentes y crecimiento económico en México, 1980-2008. Investigación Económica, 71(281). https://doi.org/10.22201/fe.01851667p.2012.281.37352. [ Links ]
Hasan, I. y Tucci, Ch. (2010). The innovation-economic growth nexus: Global evidence. Research Policy, 39(10 ). https://doi.org/10.1016/j.respol.2010.07.005. [ Links ]
Hu, A. (2015). Innovation and economic growth in east Asia: An overview. Asian Economic Policy Review, 10(1). https://doi.org/10.1111/aepr.12078. [ Links ]
Instituto Mexicano de la Propiedad Industrial (IMPI) (2018). Informe anual. https://www.impi.gob.mx [ Links ]
Instituto Nacional de Estadística y Geografía (INEGI) (2018). Sistema de cuentas nacionales. http://www.inegi.org.mx [ Links ]
Kacprzyk, A. y Doryn, W. (2017). Innovation and economic growth in old and new member states of the European Union. Economic Research-Ekonomska Istrazivanja, 30(1). https://doi.org/10.1080/1331677x.2017.1383176. [ Links ]
Krugman, P. (1993). On the number and location of cities. European Economic Review, 37(2-3 ). https://doi.org/10.1016/0014-2921(93)90017-5 [ Links ]
______(1995). Development, geography and economic theory. MIT Press. [ Links ]
Lucas, R. (1988). On the mechanics of economic development. Journal of Monetary Economics, 22(1). https://doi.org/10.1016/0304-3932(88)90168-7 [ Links ]
Maradana, R., Pradhan, R., Dash, S., Gaurav, K., Jayakumar, M. y Chatterjee, D. (2017). Does innovation promote economic growth? Evidence from European countries. Journal of Innovation and Entrepreneurship, 6(1). https://doi.org/10.1186/s13731-016-0061-9 [ Links ]
Maradana, R., Zaki, D., Pradhan, R., Gaurav, K., Dash, S., Jayakumar, M. y Sarangi, A. (2019). Innovation and economic growth in European economic area countries: The Granger Causality Approach. IIMB Management Review, 31(3). https://doi.org/10.1016/j.iimb.2019.03.002 [ Links ]
Mendoza, J., Torres, V. y Polanco, M. (2008). Desigualdad del crecimiento económico regional e innovación tecnológica en México. Comercio Exterior, 58(7).http://revistas.bancomext.gob.mx/rce/magazines/117/2/507_Mendoza_et-al.pdf. [ Links ]
Mungaray, A., Ríos, J., Aguilar, J. y Ramírez, M. (2015). La capacidad innovadora de la economía mexicana. Economía: Teoría y Práctica, 43(2). https://doi.org/10.24275/etypuam/ne/432015/mungaray [ Links ]
Nelson, R. y Winter, S. (eds.) (1982). An evolutionary theory of economic change. Harvard University Press. [ Links ]
OCDE (2015). Estudios económicos de la OCDE México. OCDE. https://doi.org/10.1787/9789264218734-es [ Links ]
OECD (2003). OECD Science, technology and industry scoreboard 2003. OECD. https://doi.org/10.1787/sti_scoreboard-2003-en [ Links ]
Pece, A., Oros, O. y Salisteanu, F. (2015). Innovation and economic growth: An empirical analysis for CEE countries. Procedia Economics and Finance, 26(1). https://doi.org/10.1016/s2212-5671(15)00874-6 [ Links ]
Peng, M., Ahlstrom, D., Carraher, S. y Shi, W (2017). An institution-based view of global IPR history. Journal of International Business Studies, 48(7). https://doi.org/10.1057/s41267-016-0061-9 [ Links ]
Ríos, J. y Castillo, M. (2015). Efectos de la capacidad innovadora en el crecimiento económico. Análisis comparativo entre países desarrollados y en desarrollo. Región y Sociedad, 64(27 ). https://doi.org/10.22198/rys. 2015.64.a312. [ Links ]
Ríos-Flores, J. y Ocegueda, J. (2018). Efectos de la capacidad innovadora en el crecimiento económico de las entidades federativas en México 2018. Estudios Fronterizos, 19. https://doi.org/10.21670/ref.1813013. [ Links ]
Rodríguez-Pose, A. y Crescenzi, R. (2008). Research and development, spillovers, innovation systems, and the genesis of regional growth in Europe. Regional Studies, 42(1). https://doi.org/10.1080/00343400701654186 [ Links ]
Rodrik, D., Subramanian, A. y Trebbi, F. (2004). Institutions rule: The primacy of institutions over geography and integration in economic development. Journal of Economic Growth, 9(2). https://doi.org/10.3386/w9305 [ Links ]
Romer, P. (1986). Increasing returns and long-run growth. The Journal of Political Economy , 94(5). https://doi.org/10.1086/261420 [ Links ]
______ (1990). Endogenous technological change. The Journal of Political Economy, 98(5), S71-S102. https://doi.org/10.1086/261725 [ Links ]
Sala-i-Martin, X. (2000). Apuntes de crecimiento económico. Antoni Bosch. [ Links ]
Schumpeter, J. (1942). Capitalism, socialism and democracy. Harper & Brothers. [ Links ]
Sedgley, N. y Elmslie, B. (2001). Agglomeration and congestion in the economics of ideas and technological change. American Journal of Economics and Sociology, 60(1). https://doi.org/10.1111/1536-7150.00056 [ Links ]
______y Elmslie, B. (2004). The geographic concentration of knowledge: Scale, agglomeration, and congestion in innovation across U.S. states. International Regional Science Review, 27(2). https://doi.org/10.1177/0160017603262401 [ Links ]
Solow, R. M. (1956). A contribution to the theory of economic growth. Quarterly Journal of Economics, 70(1). https://doi.org/10.2307/1884513 [ Links ]
Stokey, N. (1995). R&D and economic growth. The Review of Economic Studies , 62(3). https://doi.org/10.2307/2298038 [ Links ]
Tomizawa, A., Zhao, L., Bassellier, G. y Ahlstrom, D. (2019). Economic growth, innovation, institutions, and the great enrichment. Asia Pacific Journal of Management, 37(1). https://doi.org/10.1007/s10490-019-09648-2 [ Links ]
Torres-Preciado, V., Polanco-Gaytán, M. y Tinoco-Zermeño, M. (2014). Technological innovation and regional economic growth in Mexico: A spatial perspective. The Annals of Regional Science, 52(1). https://doi.org/10.1007/s00168-013-0581-1 [ Links ]
Ulku, H. (2004). R&D, innovation, and economic growth: An empirical analysis. International Monetary Fund Working Papers, No. 04/185. https://www.imf.org/-/media/Websites/IMF/imported-full-text-df/external/pubs/ft/wp/2004/_wp04185.ashx [ Links ]
______(2007). R&D, innovation, and growth: Evidence from four manufacturing sectors in OECD countries. Oxford Economic Papers, 59(3). https:// doi.org/10.1093/oep/gpl022 [ Links ]
Unger, K. (2011). La política de estímulos fiscales a ID en México. Alcances limitados en el contexto de innovación de las empresas. El Trimestre Económico, 78(309 ). https://doi.org/10.20430/ete.v78i309.27 [ Links ]
Wu, Y., Guo, X. y Marinova, D. (2017). Productivity, innovation and China's economic growth. En L. Song, R. Garnaut, C. Fang y L. Johnston (eds.). China's new sources of economic growth: Human capital, innovation and technological change (pp. 213-227). ANU Press. https://doi.org/10.22459/cnseg.07.2017.09 [ Links ]
Yanhui, W., Huiying, Z. y Jing, W. (2015). Patent elasticity, R&D intensi ty and regional innovation capacity in China. World Patent Information, 43(1). https://doi.org/10.1016/j.wpi.2015.10.003 [ Links ]
Zhou, G. y Luo, S. (2018). Higher education input, technological innovation, and economic growth in China. Sustainability, 10(8). https://doi.org/10.3390/su10082615 [ Links ]
1Compiled by the authors using data from INEGI on Gross Domestic Product (GDP) updated to base year 2013.
2Compiled by the authors using patent data from the Mexican Institute of Industrial Property (IMPI) for the period 1995-2017.
3Since 2009, the PEI has been the country's main public policy instrument for promoting innovation in the business sector.
4Among the variables tested were schooling, college and high school enrollment, and life expectancy. Strong collinearity with agglomeration was obtained for all of them.
6GDP per capita data are constant and were taken from World Bank (2018)
7As mentioned by Arellano (1993), the two-stage procedure is preferable in case of heteroscedasticity.
Received: July 10, 2020; Accepted: November 30, 2020