Servicios Personalizados
Revista
Articulo
Indicadores
-
Citado por SciELO
-
Accesos
Links relacionados
-
Similares en SciELO
Compartir
Problemas del desarrollo
versión impresa ISSN 0301-7036
Prob. Des vol.51 no.202 Ciudad de México jul./sep. 2020 Epub 09-Nov-2020
https://doi.org/10.22201/iiec.20078951e.2020.202.69516
Articles
Benefits of belonging to a coffee organization: The case of Santa María Yucuhiti, Oaxaca
a Rosario Castellanos Institute, Mexico; Email address: gustavogarduno@cipactli.org
b Doctoral student in Sustainability Sciences at the National Autonomous University of Mexico (UNAM), Mexico. Email address: aramirezl@comunidad.unam.mx.
This article uses cross-sectional data to analyze whether coffee producers in the municipality of Santa María Yucuhiti, Oaxaca -whose farmland is characterized by heterogeneous biophysical and socioeconomic conditions- have greater possibilities of accessing credit and improved productive management practices in an organic production context. Better access allows them to raise their prices, increase their production, and reduce the presence of diseases by belonging to an organization affiliated with a consolidated organization. The results indicate that producers belonging to these organizations have increased their production capabilities, as well as their income. However, it was also found that there are various socioeconomic and biophysical conditions that may well prevent such possibilities from materializing.
Key Words: coffee; productive management; latent method; producer organizations; rust
Este artículo analiza con datos de corte transversal, si productores de café con tierras de cultivo en condiciones biofísicas heterogéneas y características socioeconómicas distintas, en el municipio de Santa María Yucuhiti, Oaxaca, tienen mayores posibilidades de acceder a crédito y a mejores prácticas de manejo productivo en un contexto de producción orgánica que les permita obtener precios más altos, incrementar su producción y reducir la presencia de enfermedades, al pertenecer a una organización afiliada a una organización consolidada. Los resultados indican que los productores pertenecientes a dichas organizaciones han incrementado sus posibilidades productivas, así como de sus ingresos. Sin embargo, también se encontró que existen diferentes condiciones socioeconómicas y biofísicas que bien pueden impedir que estas posibilidades se concreten.
Palabras clave: café; manejo productivo; método latente; organizaciones de productores; roya
Clasificación JEL: O13; O18; Q13
1. INTRODUCTION
Coffee produced in Mexico mostly comes from small producers-with varying socioeconomic and biophysical characteristics-who tend to own less than two hectares of land and operate under marginalizing conditions. Furthermore, they face high production costs, volatile coffee prices, substantial difficulties accessing the market, little bargaining power, a lack of training and a lack of financing (Bernard and Spielman, 2009; Robles Berlanga, 2011). Under these conditions, the attainable benefits of coffee bean production are limited; such conditions also limit the adoption of production practices that improve productivity and quality and prevent diseases and pests (Barham et al., 2011; Beuchelt and Zeller, 2011; Barham and Weber, 2012; Bravo-Monroy et al., 2016). These circumstances have been a determining factor in the reduction of coffee production and cultivation in Mexico, as compared to the 1980s (CEDRSSA, 2018).
The present article seeks to determine whether more consolidated coffee organizations actually increase producers' benefits, regardless of their socioeconomic conditions and the biophysical characteristics of their agricultural land. To do this, the case of the Santa María Yucuhiti municipality, one of the most important coffee production regions of the state of Oaxaca, Mexico, was studied.
In this case, possible benefits include increased access to credit and improved management practices, as well as entering an organic market context that allows producers to obtain higher prices, thereby increasing their production and reducing the presence of diseases. This study focuses specifically on the presence of rust1 as a way to capture the vulnerability of coffee plantations to disease, given that in 2017-the year in which data was collected-the amount of rust recorded in the area of study was quite severe.
The hypothesis presented here is based on neo-institutional theory, which establishes that individuals socialize and organize in order to undertake shared actions and function as collective actors in the market. This is done with the aim of obtaining goods and services at a lower cost by exploiting economies of scale, managing with incomplete markets or internalizing other market failures (Téllez-Iregui and Cubillos-González, 2009; Narváez-Rodríguez, 2014; Caldentey, 2003). All of the above is translated to the case of the coffee sector, under the assumption that consolidated coffee organizations can effectively offer more benefits to their affiliates because they have the scale and organizational structure to reduce transaction costs, improve their bargaining position vis-à-vis buyers, and exploit economies of scale (Bray et al., 2002; Bernard and Spielman, 2009; Wossen et al., 2017; Ma and Abdulai, 2016; Milford, 2012; Abebaw and Haile, 2013). In the event that such an organization does not grant more benefits to certain types of producers, they can abandon it and join another which does.
Various studies indicate that small producers in general, and coffee growers in particular, have found in rural producer organizations an alternative to high production costs, volatility of coffee prices, substantial difficulties in accessing the market, poor bargaining power, and a lack of training and financing (Bray et al., 2002; Bernard and Spielman, 2009; Ito et al., 2012; Bravo-Monroy et al., 2016; Ma and Abdulai, 2016; Verhofstadt and Maertens, 2016). Such organizations offer various financial, commercial and productive strategies, such as obtaining a certification in organic or fair-trade production (Perfecto et al., 1996; Bray et al., 2002; Barham and Weber, 2012; Haggar et al., 2017; Wossen et al., 2017).
There is also evidence that these strategies improve prices and net income in particular (Valkila and Nygren, 2009; Barham et al., 2011; Beuchelt and Zeller, 2011; Barham and Weber, 2012; Ito et al., 2012; May Abdulai, 2016; Haggar et al., 2017), and that through them producers have more access to credit (Bernard and Spielman, 2009; Donovan and Poole, 2014; Wossen et al., 2017), in addition to improving certain social conditions (Barham et al., 2011; Donovan and Poole, 2014; Bravo-Monroy et al., 2016; Ibañez and Blackman, 2016; Wossen et al., 2017).
However, other studies imply that there are no benefits to belonging to an organization (Attwood and Baviskar, 1988) and that certified organizations do not improve the conditions of most producers or certain groups of producers (Beuchelt and Zeller, 2011; Lyngbæk et al., 2001).
To test the hypothesis, coffee producers were first grouped according to their biophysical characteristics and production management strategies, and in terms of access to credit and the income they receive from the sale of coffee beans.
Producers were grouped using a latent model that statistically identifies the optimal number of groups which can be obtained from a sample (Lo et al., 2001). After applying this model, it became evident that there are three groups of producers, each with different features. One group is distinguished by having the highest production, procuring the highest prices, registering the most access to credit and having the lowest proportion of rust cases. Meanwhile, the second group is defined by having the lowest production and lowest prices, the least access to credit and the highest proportion of rust cases. Finally, the third group registers average levels for these variables.
Next, the relationships between different variables (both socioeconomic and biophysical) that characterize the producers and the groups obtained from the latent model were examined using a multinomial logistic regression. One of these variables is membership in a consolidated coffee organization. This allowed for an exploration of how certain variables-in particular, belonging to a consolidated organization-influence the possibility of becoming part of the group with higher production, higher prices, better access to credit and less presence of rust.
This study uses representative data from 2017 for producers belonging to various coffee organizations as well as unaffiliated producers in the municipality of Santa María Yucuhiti, Oaxaca. In particular, consolidated organizations were considered those belonging to the State Coffee Producer Coordinator of the State of Oaxaca civil association (SCPCO) because, as explained in the following section, this is the state's largest coffee producer organization and one of its most experienced.
The area of study is a coffee production region with multiple community organizations that have organic certifications, and which is characterized by producing high quality coffee (e.g., in recent years various producers in the region have won the Cup of Excellence)2 . Yet it is also distinguished by high levels of social marginalization and limited options for the development of other agricultural activities.
The results of this study indicate that the strategies followed by SCPCO increase the possibility that all of the producer groups in consideration increase their production, average prices, access to credit and probability of not presenting rust. However, the benefits producers receive are inequitable, due partly to pre-existing conditions.
The details and points discussed in this article as a whole have not been explored in the existing literature. In particular, the presence of rust in coffee plantations, the heterogeneous biophysical conditions of the farmland, the adoption of production practices and results of their implementation in the municipality of Santa María Yucuhiti have not been considered.
The article is divided into five sections. The following section provides a brief description of environmental and socioeconomic characteristics of the area of study, and the third section presents the methods used for data collection and analysis. In the fourth section, the results of latent models and the multinomial logistic regression are provided. The last section presents a discussion of the results and conclusions.
2. AREA OF STUDY
The area of study is located in the municipality of Santa María Yucuhiti, part of the Sierra Madre del Sur (see Map 1); this region has temperate wet (79.11%) and temperate sub-humid (20.89%) climates. The municipality is comprised of 68.53% forest and 3.43% induced grassland; 98% of the soil is not suitable for agriculture, only 0.06% is used for year-round manual agriculture and 2.06% is used for seasonal manual agriculture (INEGI, 2010).
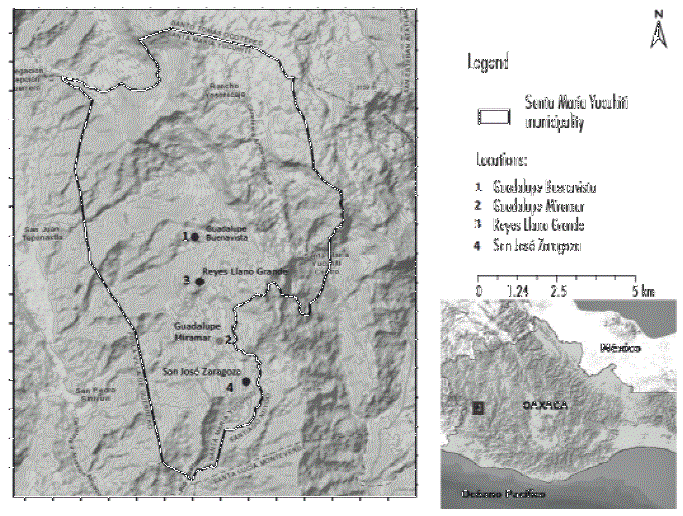
Source: prepared by the authors.
Map 1 Chosen locations in the Santa María Yucuhiti municipality, Oaxaca
In Yucuhiti, most coffee producers are organized through cooperative organizations and marketing networks that make up farmer enterprises. These organizations differ from each other in terms of their administrative complexity. Additionally, all of them have organic certifications issued by the Ministry of Agriculture and Rural Development (MARD); several have other international certifications, such as that of the United States Department of Agriculture or the European Union’s Commissioner for Agriculture.
SCPCO is the largest coffee commercialization organization in the state of Oaxaca and specializes in providing technical assistance, marketing services and low-cost credit to its members; it covers all areas of the state dedicated to coffee bean production. It has been recognized for its experience in integrating the production chain and for influencing public policies in the coffee sector. Its marketing strategies promote fair trade and it is one of the pioneers in access to specialized markets and certification seals (Calo and Wise, 2005).3 The organization exerts an important influence in the region of study through the Tee Ne Nuu and Café Yucuhiti organizations (see Table 3).
3. METHODS
To determine whether producers belonging to SCPCO affiliated organizations have better production management, producers were divided into homogeneous groups using a latent model (Muthén, 2002) according to their observed behavior regarding established production practices, prices obtained, production development and the presence of rust. The latent model was calculated using MPLUS version 7 software.
Using a multinomial logistic regression and STATA version 15 software, the question of whether belonging to a more consolidated organization is associated with more productive groups and better management was examined.
Defining grouping variables
Since most of the producers under study belong to organizations certified as organic, and independent producers assure that they cultivate their coffee according to those same criteria, the study focused on the producers’ observed behavior in relation to organic practices.
To group the producers, certain variables were established-shown in Table 1-based on organic practices at the producer level from the Rainforest Alliance and Bird Friendly certifications (based on Philpott et al. (2007)), as well as the Sustainable and Biodiverse Productive Systems project [Spanish acronym SPSB] (SPSB, 2017).4 Interviews were also conducted with Rainforest Alliance personnel in Mexico, the Mexican Certifier of Ecological Products and Processes (CERTIMEX) and the SPSB to help establish these variables.
Table 1 Variables used to constitute producer groups
Variables | Description of variables |
Environmental effect variables (measure the effects of implementing management practices) | |
Tree diversity per hectare* | The number of tree species and their relative abundance, measured with the Shannon index. |
Soil index | This index measures the quality of the soil in the coffee plot. Higher values are preferred. |
Number of trees per hectare* | An estimation of the number of trees in one hectare of the coffee plot. The Rainforest Alliance indicates that more than 70 trees are required per hectare. |
Presence of rust in coffee plantations | Indicates the presence of rust reported by producers in 2017. |
Dichotomous variable | (1 if there is rust and 0 if not) |
Shade Percentage | EThis is the percentage of shade in the coffee plot that the foliage of the trees reached. According to the certifications referred to above, more than 40% is required. |
Tree heterogeneity per hectare* | The number of tree species per hectare. The Rainforest Alliance indicates that more than 12 tree species are required. |
Production management variables (measures the implementation of management practices that improve productivity) | |
Pruning of coffee plantations | Indicates whether pruning is done on the coffee plantations on a regular basis. |
Dichotomous variable | (1 if they prune, 0 if they do not). |
Tree pruning | Indicates whether tree pruning is performed regularly. |
Dichotomous variable | (1 if they prune, 0 if they do not). |
Natural pesticides | Indicates whether natural pesticides are used on a regular basis. |
Dichotomous variable | (1 if pesticides are used, 0 if they are not). |
Use of copper oxychloride | Use of copper oxychloride on a regular basis. |
Dichotomous variable | (1 if it is used, 0 if it is not). |
Organic fertilizers | Indicates whether organic fertilizers are applied regularly. |
Dichotomous variable | (1 if they are applied, 0 if they are not). |
Practices to avoid soil erosion | The use of different management practices to avoid soil erosion during the year of the study. |
Dichotomous variable | (1 if they are used, 0 if are not). |
Coffee plantation renewal | Percentage of coffee plantations renewed at the time of the year of study. |
Environmental management variables (measure the implementation of practices that improve environmental conditions) | |
Management of epiphytes | An epiphyte management plan. |
Dichotomous variable | (1 if they have one, 0 if they do not). |
Use of dead trees | The presence of dead tres on the coffee plantation during production management. |
Dichotomous variable | (1 if there are, 0 if there are not). |
Use of centennial trees | The presence of centennial trees during production management. |
Dichotomous variable | (1 if there are, 0 if there are not). |
Income and access to credit variables | |
Access to credit | If the producer used credit the year before the study. |
Dichotomous variable | (1 if they had credit, 0 if they did not). |
Coffee price | The coffee price per kilo reported in 2017 (in parchment). |
Coffee production | The coffee production in kilos reported in 2017. |
Notes: *For these variables the results obtained from the samples are scaled to one hectare; ** Copper oxychloride is separated from the others, since according to the results of interviews with coffee growers and leaders, it is suitable for preventing rust.
Source: prepared by the authors.
The selected practices are divided into variables of environmental effects, production management, environmental management and income and access to credit. The first of these groups is made up of variables that measure the effects of implementing organic production practices (e.g.,Beer et al., 1998; Philpott et al., 2007; Haggar et al., 2017; Meylan et al., 2017).5 High values for these variables negatively affect productivity, though in the case of variables related to the soil, high values also contribute to productivity.
The second group is divided into variables which show whether the producer implements production management practices and fosters increased production-if they follow a management plan (Beer, 1987; Beer et al., 1998; Meylan et al., 2017; Barham and Weber, 2012). The third is comprised of variables which measure the adoption of management practices that favor biodiversity conservation, but do not necessarily increase productivity (Beer, 1987; Beer et al., 1998; Perfecto et al., 1996). Finally, the last group reflects efforts made by producers to achieve greater production, access to credit and higher prices; these are clearly related to the above selected variables.
Defining variables that influence grouping types
Table 2 presents variables that are assumed to be associated with the four proposed groups. They are divided into biophysical and productive characteristics of the coffee plantations and sociodemographic characteristics of household members. Additionally, membership in a consolidated organization and receipt of support from government programs are included. These variables follow those used in other articles examining the role of certifications and producer organizations in producer productivity and net income (Lyngbæk et al., 2001; Barham and Weber, 2012; Donovan and Poole, 2014; Haggar et al., 2017; Wossen et al., 2017).
Table 2 Variables that influence production performance
Variables | Description of variables |
Biophysical characteristics | |
Altitude of coffee plot | Altitude in meters of the main coffee plot. |
Slope of main coffee plot | Average slope in sexagesimal degrees of the main coffee plot. |
External revenue | |
PROCAFÉ and Coffee Production Boost * | Producers who are beneficiaries of PROCAFÉ and Coffee Production Boost. |
Dichotomous variable | (1 if household members is a beneficiary and 0 if no one is a beneficiary). |
Productive characteristics | |
Hectares designated for coffee production | Number of hectares designated for coffee production per producer. |
Household members | Number of household members per producer (over 15 years old). |
Number of productive activities of household members | Number of productive activities carried out by household members. |
Cultivated coffee varieties | Number ot coffee varieties grown on the main coffee plot. |
Sociodemographic characteristics | |
Distance to a paved road | Measured distance from the main plot to a paved road. |
Head of household´s education | Number of years of schooling attended by the head of household |
Head of household´s age | How old the head of household is. |
Head of household´s gender | This variable indicates the gender of the head of the productive household. |
Dichotomous variable | (1 if they are male, 0 if they are female) |
SCPCO membership | This variable indicates whether a producer belongs to SCPCO. |
Dichotomous variable | (1 if the head of household belongs to SCPCO, 0 if they do not). |
Notes: Standard error in parentheses. *For these variables, the SEDAR and CONAFOR beneficiary registries were compared with responses obtained from producers to corroborate the information. **For this variable, the list of SEDAR beneficiaries was compared with responses obtained from the producers to corroborate the information.
Source: prepared by the authors.
In particular, the federal programs PROCAFÉ, Coffee Production Boost and PROAGRO that were operated by MARD in the period of 2012-2018 are included. The former two programs aimed to support coffee producers through various public mechanisms-such as subsidies-to improve productivity. Meanwhile, the third program assigned subsidies to agricultural activities, to increase producers’ productivity.
As established in the hypothesis, membership in SCPCO affiliated organizations is expected to be associated with the groups that have more production management-characterized by higher production, higher prices, more access to credit and less presence of rust. This is because SCPCO has the scale and organizational structure to reduce transaction costs, improve their bargaining position vis-à-vis buyers and exploit economies of scale (Bray et al., 2002; Bernard and Spielman, 2009; Wossen et al., 2017; Ma and Abdulai, 2016; Milford, 2012; Abebaw and Haile, 2013).
Data collection
The data used here comes from the National Commission for the Knowledge and Use of Biodiversity (NCKUB). A representative and random sample of coffee growers was obtained from a list of producers belonging to different organizations present in the area of study, which included independent producers. This list was prepared in conjunction with local authorities, and a total of 144 producers belonging to five organizations and from the following localities were surveyed: Guadalupe Buenavista, Reyes Llano Grande, Guadalupe Miramar and San José Zaragoza (see Table 3 and Map 1).
Table 3 Coffee producer organizations
Organization | Producers | Percentage | Type of organization according to legal form | Organizations belonging to SCPCO |
Mixteca Alta | 35 | 25 | First level (cooperative society) | Not in SCPCO |
Café Yucuhiti | 34 | 23 | First level (cooperative society) | SCPCO |
Tee Ne Nuu | 29 | 20 | First level (cooperative society) | SCPCO |
Sierra Mixteca | 12 | 8 | First level (cooperative society) | Not in SCPCO |
Yeni Navan | 4 | 3 | First level (limited liability rural production company) | Not in SCPCO |
Independent | 30 | 21 | - | - |
Total producers | 144 | 100 | - | - |
Source: prepared by the authors.
A questionnaire with socioeconomic and production-related questions was applied to each of the producers selected. In addition, measurements were made of each producer’s main coffee plot to obtain various biophysical indicators. Data collection was carried out in 2016 and 2017. Parallel to this process, interviews were carried out with leaders in coffee cultivation in order to have a better understanding of the production landscape in the region of study.
In particular, to quantify the characteristics of the vegetation in each plot, a sampling polygon of 20 ×20 m was established in which each tree with a Diameter at Breast Height (DBH) greater than or equal to 10 cm was identified. Some variables were scaled to one hectare, as shown in table 1.
The above was performed with the support of identification guides which had been previously developed with the support of local producers and NCKUB experts. Height, DBH, and number of epiphytes were measured for each tree. Additionally, the slope and altitude of each plot were recorded.
Shade coverage was measured using a spherical concave densiometer at five points for each plot, and leaf litter depth was measured using a graduated metal ruler. A soil sample was taken in the center of the polygon, extracting a 20 ×20× 30 cm deep block, and determining its structure, porosity, level of erosion, color, odor, organic matter present, dead matter and biological activity to generate a soil quality index, based on Tovar et al. (2013). Higher values are associated with better soil quality.
Table 3 demonstrates the number of producers sampled and the organizations with which they are affiliated, as well as what type of organization they are, according to their legal status. The Café Yucuhiti and Tee Ne Nuu organizations are first level (cooperative societies) and belong to SCPCO, which is a second level organization (an organization made up of cooperatives).
4. RESULTS
Identified groups
Table 4 shows the results of statistical tests carried out to select the optimal number of groups. According to the sample, we examined whether two or three groups would better represent its heterogeneity.
Table 4 Statical tests for latent models
Statistical tests | Model with two groups | Model with three groups |
Akaike Information Criterion (AIC) | 9492.462 | 9370.079 |
Bayesian Information Criterion (BIC) | 9614.224 | 9542.328 |
Sample-size adjusted information Criterion (ABIC) | 9484.489 | 9358.801 |
Entropy | 0.812 | 0.898 |
Pearson Chi-square* | 369.857 | 360.027 |
(P-Value 0.0) | (P-Value 0.0) | |
Likelihood-ratio Chi-square* | 304.639 | 300.871 |
(P-Value 0.002) | (P-Value 0.001) | |
Boostrap likelihood ratio (BLR),a | 218.836 | 156.383 |
*Difference between groups | (P-Value 0.0) | (P-Value 0.0) |
Vuong-Lo-Mendell-Rubin (VLMR),a | 218.836 | 152.963 |
*Difference between groups | (P-Value 0.24) | (P-Value 0.063) |
Lo-Mendell-Rubin Adjuted (LMRA), a | 214.051 | 152.963 |
*Difference between groups | (P-Value 0.25) | (P-Value 0.063) |
Notes: a For the two-group model, these statistical tests compare a one-group model against a two-group model. For the three-group model, these statistical tests compare a two-group model against a three-group model; * P-values in parentheses.
Source: prepared by the authors.
For the BLR test, a model with two groups is rejected, but a model with three groups is not rejected. This same conclusion is reached for the VLMR and LMRA tests (see Table 4). For the AIC, BIC and ABIC information criteria, the three-group model is preferred to the two-group model because it has lower values (Nylund et al., 2007). The Entropy Index also favors the model with three groups, since values closer to 1 indicate better accuracy in classifying the model.
For the Pearson and Likelihood Ratio tests, the p-value indicates that they are significant for both models, so the two cases should be rejected. Based on this information, there are elements to support selecting either model; however, the model with three groups was selected for this study, since according to Nylund et al. (2007) the information criteria and BLR are the most important tests for the selection of a model. Furthermore, following Collins and Lanza (2009), the three-group model provides more information about the coffee producers’ characteristics.
Table 5 shows the results of division into three groups with respect to the selected variables (see table 1). It is important to highlight that the variables which reflect the adoption of practices to avoid soil erosion and management of epiphytes were not included because all of the producers indicated that they use such practices.
Table 5 Characteristics of the selected groupsa
Variables |
Group 1 (number of producers 28) |
Group 2 (number of producers: 75) |
Group 3 (number of producers: 41) |
Environmental effect variables | |||
Tree diversity per hectare | 0.506 *** | 1.324 *** | 1.879*** |
(0.094) | (0.054) | (0.063) | |
Soil index | 0.744*** | 0.708 *** | 0.684 *** |
(0.023) | (0.015) | (0.020) | |
Number of trees per hectare | 123.916*** | 220.908*** | 379.588*** |
(20.166) | (14.906) | (27.807) | |
Presence of rust (dichotomous variable) | 0.464 *** | 0.823 *** | 0.843 *** |
(0.101) | (0.051) | (0.059) | |
Shade percentage | 56.055*** | 70.485 *** | 74.795 *** |
(3.048) | (1.543) | (1.475) | |
Tree heterogeneity per hectare | 50.15 *** | 112.25*** | 195.5 *** |
(0.193) | (0.249) | (0.438) | |
Production management variables | |||
Pruning of coffee plantations | .471*** | 0.414*** | 0.455*** |
(0.097) | (0.051) | (0.083) | |
Pruning of trees | .530*** | 0.377*** | 0.407*** |
(0.101) | (0.060) | (0.082) | |
Naural pesticides | 0.698*** | 0.547*** | 0.520*** |
(0.110) | (0.069) | (0.091) | |
Use of copper oxychloride | 0.594*** | 0.420*** | 0.407*** |
(0.111) | (0.070) | (0.095) | |
Organic fertilizers | 0.773*** | .421*** | 0.403*** |
(0.079) | (0.064) | (0.095) | |
Coffee plantation renewal | 48.477*** | 38.791*** | 44.144*** |
(7.696) | (4.766) | (5.223) | |
Environmental management variables | |||
Use of dead trees | 0.242*** | 0.480*** | 0.356*** |
(0.083) | (0.062) | (0.077) | |
Use of centennial trees | 0.671*** | 0.757*** | 0.839*** |
(0.092) | (0.054) | (0.067) | |
Income and access to credit variables | |||
Credit | 0.608*** | 0.559*** | 0.372*** |
(0.095) | (0.065) | (0.084) | |
Coffee price | 56.875 *** | 54.881 *** | 52.538 *** |
(3.2441) | (2.423) | (1.825) | |
Coffee production per hectare | 161.434 *** | 120.052*** | 63.035 *** |
(35.972) | (17.005) | (11.815) |
Notes: a These results indicate the mean of each variable; *Significance level of 0.10; **Significance level of 0.05; ***Significance level of 0.01; Standard error in parentheses.
Source: prepared by the authors.
With regard to the set of environmental effect variables, important differences are recorded between the groups identified; for example, group 1 has a lower shade index and number of trees. Furthermore, the diversity and heterogeneity of trees is the lowest for this group. In contrast, group 3 has the highest values for these variables.
Producers in group 1 have a higher soil index as compared to the other groups, and producers that belong in group 3 have the lowest. The results also show that producers in the second and third groups have more than 80% rust; on the other hand, producers in group 1 have 46% of rust cases.
Another important observation is the gap that exists between the groups in terms of prices, production and credit. Group 1 is distinguished by achieving higher prices, production and credit as compared to the other groups. By contrast, producers in group 3 have the lowest values for these variables. In particular, the difference in production between the third group and the other groups is more than 90%. It is important to highlight that these results are influenced by the worst rust crisis that this has region suffered (during the period of 2015-2017, the year in which data was collected) according to coffee producers and leaders who were interviewed (all obtained extraordinarily low results compared to other years).
The results obtained for shade, heterogeneity, and number of trees in groups 2 and 3 indicate that they are characterized by a rustic management style with low production levels (Lyngbæk et al., 2001; Haggar et al., 2017; Meylan et al., 2017).
The proportional access to credit that the groups have is another aspect to highlight. For example, producers in group 3 have a rate of 37% access, which is higher than the rate of 2.5% seen at the national level (Robles Berlanga, 2011). This can be explained by the fact that a substantial number of producers receive credit not only from their organizations, but also from intermediaries.
Regarding management practices, it can be observed that less than 50% prune their coffee plantations on a regular basis. Group 1 producers have the highest compliance rate, but the difference between groups is very small. Regular tree pruning is complied with by just over 50% of producers in the first group, while producers in the other groups are less than 41% compliant. This then becomes one of the factors that explains the difference in degrees of shade between the identified groups.
The most important difference between producers in group 1 and the other groups is seen in the regular use of pesticides and fertilizers. This can also help explain differences in the presence of rust and production between the groups in question.
Finally, group 1 producers have the highest average for renewal of their coffee plantations, while those with the lowest average are in group 2. This indicates that all of the producers have changed a significant proportion of their coffee plantations-either due to rust or to increase their productivity-because their coffee plantations were already older than recommended.
Influence of selected variables on groups identified
Table 6 shows the results of a multinomial logistic regression that examines the relationship between the identified groups and the chosen or covariate variables (see Table 2), using group 3 as a base.
Table 6 Results of multinomial logistic regression.
Dependent variable: groups identified | |||
Base group 3 | Variables | Group 1 coefficients | Group 2 coefficients |
Biophysical characteristics | Altitude of the main coffee plot | .0040*** | .0025** |
(.0014) | (.0012) | ||
Slope of the main coffee plot | -.0771*** | -.0531** | |
(.0288) | (.0248) | ||
External revenue | Coffee promotion (dichotomous variable) | -.1438 | .157 |
(.9783) | (.7548) | ||
PROAGRO (dichotomous variable) | .033 | .4838 | |
(.8655) | (.6523) | ||
Productive characteristics | Hectares designated for coffee production | .6700*** | .5574** |
(.257) | (.221) | ||
Number of productive activities done by household members | 1.037*** | .1402 | |
(.380) | (.2872) | ||
Coffee varieties per hectare | -.4648 * | -.2208 | |
(.265) | (.1736) | ||
Sociodemographic characteristics | Distance to a paved road | -1.128 | -.57 |
(1.55) | (1.174) | ||
Head of household education (years of schooling) | -.4215 | -.0387 | |
(.3490) | (.253) | ||
Head of household age (years) | -.0507* | -.0505** | |
(.0286) | (.0200) | ||
Head of household age (years) squared | -.000387 | -.0000312 | |
(.00021 3) | (.0001482) | ||
Head of the household gender (dichotomous variable) | .9821 | .3944 | |
(.7415) | (.5411) | ||
Members of the household | -.2126 | -.213 | |
(.2476) | (.1858) | ||
SCPCO membership | 1.4308** | .966* | |
(.665) | (.560) | ||
Constant | -2.432 | .30 | |
(3.642) | (2.373) |
Notes: Wald chi-squared (24) = 40.87 (Probability > chi-squared=0.0172); Standard error in parentheses; *Significance level of 0.10; ** Significance level of 0.05; *** Significance level of 0.01; The possibility of multicollinearity is taken into account in this regression; Group 3 is taken as the basis for the estimations.
Source: prepared by the authors.
Since the Coffee promotion and PROAGRO variables could be endogenous-due to their probable correlation with the ability to obtain resources-Hausman (1978) tests were used to detect endogeneity. The results found that none of them are endogenous.
The results in Table 6 show that the altitude of the main coffee plot and the number of hectares designated for coffee production are statistically significant and increase the possibility of belonging to groups 1 and 2, compared to group 3. Additionally, the number of productive activities done by members households increase the possibility of belonging to group 1 rather than group 3. On the other hand, the land slope and head of household’s age reduce the possibility of belonging to groups 1 and 2, compared to group 3. The varieties of coffee bean per hectare also decrease the possibility of belonging to group 1 rather than group 3. It is important to note that the relationship between the number of coffee varieties and least productive producer group contrasts with the findings of Benítez-García et al. (2015), in that they report that the most productive producers have more varieties and less productive ones have less.
In particular, the altitude, slope, and head of household’s age have very little effect (see Table 6), though it should be considered that there may be important differences between producers in terms of altitude.
Results indicate that the relative probability of belonging to group 1 or 2 instead of group 3 for producers belonging to SCPCO is more than double than that of producers not belonging to SCPCO (keeping the other variables constant). All other variables are not significantly associated with any group, which is a surprising factor in terms of government support for producers.
5. DISCUSSION AND CONCLUSIONS
The results obtained indicate that the heterogeneity which characterizes producers can be synthesized into three groups that share similar characteristics. The first group is distinguished by having higher production levels, more access to credit and a low level of tree diversity, heterogeneity and number compared to the other groups. The producers in the other groups have characteristics which oppose those favoring conservation, as indicated by Perfecto et al. (1996). Compliance with management practices is consistent with these characteristics. That is, the first group is more compliant in terms of production management whereas the other groups are more compliant with respect to environmental management.
All three groups had rust present, although the least productive groups with the most precarious management practices were most affected. This coincides with the argument of Avelino et al. (2015) regarding factors that led to the expansion of rust in Central America: lack of management, adverse economic conditions (e.g., low prices and high prices for inputs) and conducive environmental factors. In the present case, these factors are mostly identified with the least productive of the selected groups.
Meanwhile, the prices obtained by coffee growers in the least productive groups tend to be lower than those in the most productive group. Furthermore, the results indicate that producers who have organic certifications are in the most productive group (although there are some in the second and third groups). This is consistent with Calo and Wise (2005), who note that producers receive different prices if they have an organic certification.
Although the price difference is minimal, it is significant and indicates a positive relationship between management, production, quality and price-as indicated by Taugourdeau et al. (2014). The minimal price difference may be due, following Valkila and Nygren (2009), Weber (2011) and De Janvry et al. (2011), to the fact that a significant proportion of producers sell their beans to various buyers at different prices; this may be a consequence of the limited demand for certain types of certified coffee. Another probable cause is the presence of rust, which reduced the coffee supply in the region of study and Mexico overall.
The results obtained also indicate that SCPCO’s strategies increase the possibilities of all producers increasing their production, average prices, access to credit and the probability of not having rust. However, this does not mean that they necessarily improve their production management. It was also found that there are different conditions that influence the possibility of belonging to the most productive (or least productive) producer group. Examples of this include altitude and slope of the coffee plantation, in addition to the head of household’s age and the number of hectares dedicated to coffee production. The former three factors are pre-existing (the producer has no control over them) and the latter is determined by the total area that the producers have available (which also means that it is partially pre-existing).6 Less productive producers are more likely to have pre-existing conditions that prevent them from improving their production management, production, access to credit, and prices. Consequently, even if organizations provide goods and services to improve the least productive producers’ potential, the possibilities of achieving this are slim. The opposite is true for the most productive producers.
This finding coincides with other studies that point out the fundamental role that pre-existing conditions play in favoring or harming producers (Barham and Weber, 2012; Abebaw and Haile, 2013; Donovan and Poole, 2014; Ma and Abdulai, 2016; Verhofstadt and Maertens, 2016; Haggar et al., 2017; Wossen et al., 2017). For example, age, housing capacity, gender, distance between the plot and markets, productive land area and education are important factors that influence the benefits organizations provide. Institutional arrangements and land tenure also influence the strategies that producers adopt (Méndez et al., 2009).
On the other hand, public mechanisms of strengthening coffee production are not associated with better production management. This result coincides with Robles Berlanga (2011), who explains that state intervention-especially in coffee-growing municipalities-is minimal and does not generate positive effects. Furthermore, support meant to improve agricultural production does not impact coffee cultivation.
The prevailing conditions of the coffee sector (e.g., the aftermath of the rust epidemic) and decreasing coffee prices have further reduced the benefits of coffee bean production, and therefore, have increased the opportunity cost of investing in small producers’ production management. These conditions also affect the ability of organizations to provide goods and services to their members. In addition to the factors mentioned above, it is expected that climate change will contribute to conditions which favor diseases and pests, requiring producers to improve their production management.
The consequences of these conditions may be quite negative for producers’ well-being and the conservation of biodiversity, since they could replace coffee production with activities that involve changes in land use, such as agricultural activities (indicated most of the coffee leaders interviewed in the region). This sort of situation was previously experienced in the 1999-2004 period when coffee prices saw historical lows (Castro et al., 2004).
In this context, it is important to find public and private mechanisms that encourage the adoption of practices which improve productivity and quality-as well as prevent diseases and pests-by small producers who grow coffee under shade. This is particularly critical for the most disadvantaged producers, in biophysical and socioeconomic terms.
This will require changing the way governmental organizations and support programs work to promote economies of scale; also, it means avoiding the implementation of generalized production standards which assume biophysical and socioeconomic conditions that are not appropriate to local characteristics, as some certifications are known to do (Gonzalez and Nigh, 2005; Barham and Weber, 2012).
ACKNOWLEDGEMENTS
Thank you to the coffee growers and leaders of Santa María Yucuhiti, as well as to NCKUB, the SPSB project technicians, the Rainforest Alliance, and CERTIMEX for their support in carrying out this research. Finally, a special recognition to Jimena Deschamps Lomelí for her support in preparing the map of the area of study.
REFERENCES
Abebaw, D. y Haile, M. G. (2013), "The impact of cooperatives on agricultural technology adoption: Empirical evidence from Ethiopia", Food Policy, vol. 38, núm. 1, DOI <10.1016/j.foodpol.2012.10.003> [ Links ]
Attwood, D. y Baviskar, B. (1988), Who shares? Cooperatives and rural development, India, Oxford University Press. [ Links ]
Avelino, J., Cristancho, M., Georgiou, S., Imbach, P., Aguilar, L., Bornemann, P. L., Anzueto, F. y Hruska, C. M. (2015), "The coffee rust crises in Colombia and Central America (2008-2013): Impacts, plausible causes and proposed solutions", Food Security, vol. 7, núm. 2, DOI <10.1007/ s12571-015-0446-9> [ Links ]
Barham, B. L. y Weber, J. G. (2012), "The economic sustainability of certified coffee: Recent evidence from Mexico and Peru", World Development, vol. 40, núm. 6, DOI <10.1016/j.worlddev.2011.11.005> [ Links ]
______, Callenes, M., Lewis, J., Gitter, S. y Weber, J. (2011), "Fair trade/organic coffee, rural livelihoods, and the 'agrarian question': Southern mexican coffee families in transition", World Development, vol. 39, núm. 1, DOI <10.1016/j.worlddev.2010.08.005> [ Links ]
Beer, J. (1987), "Advantages, disadvantages and desirable characteristics of shade trees for coffee, cocoa and tea", Agroforestal Systems, vol. 5, núm. 3, DOI <10.1007/BF00046410> [ Links ]
Beer, J., Muschler, R., Somarriba, E. y Kass, D. (1998), "Shade management in coffee and cacao plantations-a review", Agroforestal Systems , vol. 38, núm. 1, DOI <https://doi.org/10.1023/A:1005956528316> [ Links ]
Benítez-García, E., Jaramillo-Villanueva, J. L., Escobedo-Garrido, S. y Mora-Flores, S. (2015), "Caracterización de la producción y del comercio de café en el municipio de Cuetzalan, Puebla", Agricultura Sociedad y Desarrollo, vol. 12, núm. 2, DOI <10.22231/asyd.v12i2.147> [ Links ]
______y Spielman, D. J. (2009), "Reaching the rural poor through rural producer organizations? A study of agricultural marketing cooperatives in Ethiopia", Food Policy, vol. 34, núm. 1, DOI <10.1016/j.foodpol.2008.08.001> [ Links ]
Beuchelt, T. D. y Zeller, M. (2011), "Profits and poverty: Certification's troubled link for Nicaragua's organic and fairtrade coffee producers", Ecological Economics, vol. 70, núm. 7, DOI <10.1016/j.ecolecon.2011.01.005> [ Links ]
Blundell, R. y Smith, R. J. (1986), "An exogeneity test for a simultaneous equation tobit model with an application to labor supply", Econometrica, vol. 54, núm. 3, DOI <10.2307/1911314> [ Links ]
Bravo-Monroy, L., Potts, S. G. y Tzanopoulos, J. (2016), "Drivers influencing farmer decisions for adopting organic or conventional coffee management practices", Food Policy , vol. 58, DOI <10.1016/j.foodpol.2015.11.003> [ Links ]
Bray, D. B., Sánchez, J. L. P. y Murphy, E. C. (2002), "Social dimensions of organic coffee production in Mexico: Lessons for eco-labe-ling initiatives", Society and Natural Resources, vol. 15, núm. 5, DOI <10.1080/08941920252866783> [ Links ]
Caldentey, P. (2003), "Neoinstitucionalismo y economía agroalimentaria", Contribuciones a la Economía, vol. 2, núm. 2. Recuperado de <http://www.eumed.net/ce/pca-agroali.htm> [ Links ]
Calo, B. M. y Wise, T. A. (2005), Revaluing peasant coffee production: Organic and fair trade markets in Mexico, USA, Global Development and Environment Institute, Tufus University. Recuperado de <https://www.iatp.org/news/revaluing-peasant-coffee-production-organic-and-fair-trade-markets-in-mexico> [ Links ]
Castro, F., Montes, E. y Raine, M. (2004), Centroamérica, la crisis cafetalera: efectos y estrategias para hacerle frente, World Bank, Washington, DC. Recuperado de <http://documentos.bancomundial.org/curated/es/573051468743167658/Centroam-233-rica-la-crisis-cafetalera-Efectos-y-estrategias-para-hacerle-frente> [ Links ]
Centro de Estudios para el Desarrollo Rural Sustentable y la Soberanía Alimentaria (CEDRSSA) (2018), El café en México: diagnóstico y perspectiva. Recuperado de <http://www.cedrssa.gob.mx/files/10/30El%20caf%C3%A9%20en%20M%C3%A9xico:%20diagn%C3%B3stico%20y%20perspectiva.pdf> [ Links ]
Collins, M. L. y Lanza, T. S. (2009), Latent class and latent transition analysis: with applications in the social, behavioral, and health sciences, Estados Unidos, John Wiley & Sons. [ Links ]
De Janvry, A., Mcintosh, C. y Sadoulet, E. (2011), Fair trade and free entry: The dissipation of producer benefits in a disequilibrium market, Documento de trabajo, Goldman School of Public Policy, Universidad de California Berkeley. Recuperado de <https://gspp.berkeley.edu/research/working-paper-series/fair-trade-and-free-entry-the-dissipation-of-producer-benefits-in-a-disequi> [ Links ]
Donovan, J. y Poole, N. (2014), "Changing asset endowments and smallholder participation in higher value markets: Evidence from certified coffee producers in Nicaragua", Food Policy , vol. 44, DOI <https://doi.org/10.1016/j.foodpol.2013.09.010> [ Links ]
González, A. A. y Nigh, R. (2005), "Smallholder participation and certification of organic products in Mexico", Journal of Rural Studies, vol. 21, núm. 4, <https://doi.org/10.1016/j.jrurstud.2005.08.004> [ Links ]
Haggar, J., Soto, G., Casanoves, F. y Virginio, E. M. (2017), "Environmental-economic benefits and trade-offs on sustainably certified coffee farms", Ecological Indicators, vol. 79, DOI <https://doi.org/10.1016/j.eco-lind.2017.04.023> [ Links ]
Hausman, J. (1978), "Specification tests in econometrics", Econometrica , vol. 46, núm. 6, DOI <10.2307/1913827> [ Links ]
Ibañez, M. y Blackman, A. (2016), "Is eco-certification a win-win for developing country agriculture? Organic coffee certification in Colombia", World Development , vol. 82, DOI <10.1016/j.worlddev.2016.01.004> [ Links ]
Instituto Nacional de Estadística y Geografía (INEGI) (2010), Compendio de información geográfica municipal Santa María Yucuhiti, Oaxaca. Recuperado de <http://www3.inegi.org.mx/contenidos/app/mexicocifras/datos_geograficos/20/20446.pdf> [ Links ]
Ito, J., Bao, Z. y Su, Q. (2012), "Distributional effects of agricultural cooperatives in China: Exclusion of smallholders and potential gains on participation", Food Policy , vol. 37, núm. 6, DOI <10.1016/j.foodpol.2012.07.009> [ Links ]
Lo, Y., Mendell, N. R y Rubin, D. B. (2001), "Testing the number of compo nents in a normal mixture", Biometrika, vol. 88, núm. 3. Recuperado de <http://www.jstor.org/stable/2673445> [ Links ]
Lyngbæk, A. E., Muschler, R. G. y Sinclair, F. L. (2001), "Productivity and profitability of multistrata organic versus conventional coffee farms in Costa Rica", Agroforestry Systems, vol. 53, núm. 2, DOI <10.1023/A:1013332722014> [ Links ]
Ma, W y Abdulai, A. (2016), "Does cooperative membership improve household welfare? Evidence from apple farmers in China", Food Policy , vol. 58, DOI <10.1016/j.foodpol.2015.12.002> [ Links ]
Méndez, V. E., Shapiro, E. N. y Gilbert, G. S. (2009), "Cooperative management and its effects on shade tree diversity, soil properties and ecosystem services of coffee plantations in western El Salvador", Agroforestry Systems , vol. 76. Recuperado de <https://link.springer.com/article/10.1007/s10457-009-9220-3> [ Links ]
Meylan, L., Gary, C., Allinne, C., Ortiz, J., Jackson, L. y Rapidel, B. (2017), "Evaluating the effect of shade trees on provision of ecosystem services in intensively managed coffee plantations", Agriculture, Ecosystems and Environment, vol. 245, DOI <10.1016/j.agee.2017.05.005> [ Links ]
Milford, A. B. (2012), "The pro-competitive effect of coffee cooperatives in Chiapas, Mexico", Journal of Agricultural & Food Industrial Organization, vol. 10, núm. 1. Recuperado de <https://www.degruyter.com/view/jour-nals/jafio/jafio-overview.xml> [ Links ]
MPLUS (2015) (Versión 7) [software]. Los Angeles, CA, USA, Muthén & Muthén. [ Links ]
Muthén, B. O. (2002), "Beyond SEM: General latent variable modeling", Behaviormetrika, vol. 29, núm. 1, DOI <https://psycnet.apa.org/doi/10.2333/bhmk.29.81"\t"_blank> [ Links ]
Narváez-Rodríguez, C. C. (2014), "Asociaciones y cooperativas rurales: factores internos y externos que influyen en su estabilidad y eficiencia. Una reflexión sobre el caso de Viotá, Cundinamarca", Cooperativismo & Desarrollo, vol. 22, núm. 04. Recuperado de <http://dialnet.unirioja.es/descarga/articulo/5243237.pdf> [ Links ]
Nylund, K., Asparouhov, T. y Muthen, B. O. (2007), "Deciding on the number of classes in latent class analysis and growth mixture modeling: A Monte Carlo simulation study", Structural Equation Modeling, vol. 14, núm. 4, DOI <10.1080/10705510701575396> [ Links ]
Perfecto, I., Rice, R. A., Greenberg, R. y van der Voort, M. E. (1996), "Shade coffee: A disappearing refuge for biodiversity", BioScience, vol. 46, núm. 8, DOI <10.2307/1312989> [ Links ]
Philpott, M. S., Bichier, P., Rice, R. y Greenberg, R. (2007), "Field-testing ecological and economic benefits of coffee certification programs", Conservation Biology, vol. 21, núm. 4. Recuperado de <http://www.jstor.org/stable/4620911> [ Links ]
Robles Berlanga, H. M. (2011), Los productores de café en México: problemática y ejercicio del presupuesto, Mexican Rural Development Research Reports. Recuperado de <https://www.wilsoncenter.org/sites/default/files/Hector_Robles_Cafe_Monografia_14.pdf> [ Links ]
Sistemas Productivos Sostenibles y Biodiversidad (SPSB) (2017), Prácticas amigables con la Biodiversidad. Recuperado de <https://www.biodiversidad.gob.mx/SPSB/pdf/TablaCafe_publicada.pdf> [ Links ]
Taugourdeau, S., Le-Maire, G., Avelino, J., Jones, J., Ramirez, L. G., Jara-Quesada, M., Charbonnier, F., Gómez-Delgado, F., Harmand, J. M., Rapidel, B., Vaast, P. y Roupsar, O. (2014), "Leaf área index as an indicator of ecosystem services and management practices: An application for coffee agroforestry", Agriculture, Ecosystems & Environment, vol. 192, DOI <https://doi.org/10.1016/j.agee.2014.03.042> [ Links ]
Téllez-Iregui, G. y Cubillos-González, A. (2009), "Relaciones entre el enfoque neoinstitucional, desarrollo y medio ambiente", Opera, vol. 9, núm. 9. Recuperado de <https://revistas.uexternado.edu.co/index.php/opera/article/view/696/658> [ Links ]
Tovar, E., Calderón-Mandujano, R., Toledo-Espinoza, A. y Estrada-Santos Y. (2013), Técnicas de monitoreo y evaluación de servicios ecosistémicos y biodiversidad para la zona Sierra y Costa de Chiapas. Documento de trabajo. Recuperado de <https://www.biodiversidad.gob.mx/corredor/SPSB/pdf/bazar_12.pdf> [ Links ]
Valkila, J. y Nygren, A. (2009), "Impacts of fair trade certification on coffee farmers, cooperatives, and laborers in Nicaragua", Agriculture and Human Values, vol. 27, DOI <https://doi.org/10.1007/s10460-009-9208-7> [ Links ]
Verhofstadt, E. y Maertens, M. (2016), "Can agricultural cooperatives reduce poverty? Heterogeneous impact of cooperative membership on farmers' welfare in Rwanda", Applied Economic Perspectives and Policy, vol. 37, núm. 1, DOI <10.1093/aepp/ppu021> [ Links ]
Weber, J. (2011), "How much more do growers receive for fair trade organic coffee?", Food Policy , 36, núm. 5, DOI <https://doi.org/10.1016/j.foodpol.2011.05.007> [ Links ]
Wossen, T., Abdoulaye, T., Alena, A., Haile, M. G., Feleke, S., Olanrewaju, A. y Manyong, V. (2017), "Impacts of extension access and cooperative membership on technology adoption and household welfare", Journal of Rural Studies , vol. 54, DOI <https://doi.org/10.1016/j.jrurstud.2017.06.022> [ Links ]
1This is a disease caused by the Hemileia vastatrix fungus. Infection by this fungus causes leaves to fall prematurely and damages coffee production by up to 90%.
2Event organized by Alliance for Coffee Excellence and the Mexican Association of the Coffee Production Chain, that seeks to identify the best coffee in Mexico.
4This was a project executed by the National Commission for the Knowledge and Use of Biodiversity (NCKUB) from 2013 to 2018, with the support of the Global Environment Facility.
Received: November 20, 2019; Accepted: April 06, 2020