Servicios Personalizados
Revista
Articulo
Indicadores
-
Citado por SciELO
-
Accesos
Links relacionados
-
Similares en SciELO
Compartir
Problemas del desarrollo
versión impresa ISSN 0301-7036
Prob. Des vol.49 no.195 Ciudad de México oct./dic. 2018
https://doi.org/10.22201/iiec.20078951e.2018.195.64001
Articles
The Impact of New Technologies on Jobs in Mexico
1 Institute for Economic Research at the UNAM, Mexico. E-mail addresses: minian.economia@gmail.com and amonroy@unam.mx, respectively.
This study evaluates job vulnerability to the advent of new technologies. Using the Frey and Osborne (2013) method, the estimate is that 63% of all jobs and 64.5% of manufacturing jobs in Mexico are at risk of being automated, making the least skilled workers the most vulnerable. Only the technical feasibility of automation was taken into account in this estimate; estimating the number of jobs that would truly be displaced would require analyzing economic, political, and social factors. Historically, the main consequence of automation has consisted of major changes to the job structure and widespread unemployment. Market mechanisms and public policy have been able to offset the impact of technology shocks, so that even as technological change displaces workers, it also creates new jobs by furnishing investment opportunities.
Key Words: Manufacturing jobs; technological change; investment; government policy
En el presente estudio se evalúa la vulnerabilidad del empleo ante el avance de las nuevas tecnologías. Con la metodología de Frey y Osborne (2013), se estima que 63% del empleo total y 64.5% del empleo manufacturero en México está en riesgo de ser automatizado, ocasionando que sean los trabajadores menos calificados los más vulnerables. Para ello se estimó exclusivamente la factibilidad técnica de automatización; el empleo que realmente sería desplazado requeriría analizar factores económicos, políticos y sociales. Históricamente, la principal consecuencia de la automatización han sido los cambios significativos en la estructura laboral y no el desempleo masivo. Los mecanismos de mercado y las políticas públicas han compensado los impactos de los shocks tecnológicos. De tal manera que aunque el cambio tecnológico desplaza trabajadores, también genera nuevos empleos al crear oportunidades de inversión.
Palabras clave: Empleo manufacturero; cambio tecnológico; automatización; inversiones; políticas gubernamentales
Clasificación JEL: E24; J08; J31; O33; O38
1. INTRODUCTION
Fear of technological dependence has been around for several centuries, and it has been documented to get worse in times of radical technological change (Mokyr et al., 2015). Analysis of the effects of technological change on employment dates back to the classic economists, and although a widely known and amply studied topic,2 continues to be controversial in light of the heterogeneity of the conclusions drawn in the diverse theoretical schools of thought and empirical evidence.
In broad strokes, theorists agree that technology tends to replace human labor, and that there are mechanisms that can offset or mitigate the adverse fallout of technological change on the employment level, even though they differ significantly in terms of the ability and speed of these offsetting mechanisms to restore full employment in the labor market. On the one hand, some hold that technology-related unemployment is fleeting, as the labor market balance will be "automatically" restored to full employment; on the other, some assert that due to the rigidities of the prices of factors, limits on the feasibility of the substitution of factors, the industrial structure, and the behavior of demand, the return to full employment in the labor market can require very long time periods and in the worst-case scenario, can even become structural unemployment (Standing, 1984).
Proponents of the “automatic” offsetting stance highlight the neoclassical school, which, following the Jean-Baptiste Say vision, says the following:
...Say's law of markets excludes technological unemployment. If machines displace labor an alternative opens up: either workers will be absorbed, because as unit prices fall, so do prices, and demand for the product rises, thereby driving up the demand for labor, or the displaced workers will accept lower wages, bringing with it the substitution of capital for labor in other sectors and a trend toward production in the capital-product ratio that will be lower (Standing, 1984, p. 160).
In neoclassical theory, production growth is endogenous and depends-in the long term-on the rise in productivity and the expansion of the economically active population. In that context, technical change entails an increase in income due to the rise in productivity and the quality of the goods, given a certain level of the workforce (driven by demographic conditions). Here, a portion of the income increase, derived from technical change, is distribution across the economic agents (workers and capitalists) at the innovative companies; another portion is transferred to the rest of society via lower prices, which increase real wages and, therefore, the demand for goods and services. In other words, greater productivity generates income and, therefore, an equivalent increase in demand, although note necessarily for the goods made by the innovative companies.
Pursuant to the predominant school, technical change activates market mechanisms that make it possible to efficiently reassign labor to companies that are increasing their production, offsetting job cuts at other companies. To guarantee that these offsetting mechanisms are effective, the neoclassicals stance holds that the following is required: 1) absolute flexibility in the labor and capital market; 2) that the workforce be homogeneous; and 3) that there are no barriers to the mobility of productive factors, so their transfer from one sector to another is immediate.
The speed and ability of these offsetting mechanisms to “automatically” restore full employment in the labor market have been seriously challenged throughout history. Specifically, Ricardo (1959, p. 294) asserted that “the demand for labor will continue to rise as capital increases, but not in proportion to that increase; the ratio shall, necessarily, be decreasing.” In the same sense, Marx (2010, p. 368) maintained that “the increase in the labor required to produce the instruments of labor themselves, the machinery, coal, etc., must be less than the reduction in labor achieved by the employment of machinery.” Moreover, the speed at which technology replaces human labor is greater than the capacity of the economy to create new jobs for the displaced workers. In the words of Keynes (1986, p. 330), technological unemployment will arise "...due to our discovery of means of economizing the use of labor outrunning the pace at which we can find new uses for labor.” Finally, there are factors that can put the brakes on compensation mechanisms. For example, a low value for the marginal efficiency of capital can undermine the effect of the effective demand on creating new jobs (Keynes, 2003) and, given that innovation is an ongoing process, a delay in the offsetting can lead to permanent structural unemployment (Piva and Vivarelli, 2017).
In spite of the adverse impacts of technological change on the level of employment and the labor structure, economists agree that its net socioeconomic effects are positive, so governments should not try to interfere. In this regard, Ricardo (1959, p. 295) wrote:
The State should never discourage the use of machinery, because if capital is not permitted to obtain higher net income than the use of machinery will yield within the country, it will be carried abroad, and with that, it will for labor be even more disheartening than the maximum and widespread use of machinery; in effect, as long as the capital is employed within the kingdom, it will create a certain demand for labor; the machinery cannot operate without the assistance of man, nor can it be manufactured without the contribution of his labor.
Finally, critics of this “automatic” offsetting note that although during transition periods the offsetting mechanisms do struggle indeed to restore full employment to the labor market, in the long term, they acknowledge that it is likely that they will counteract unemployment, because with technological change "entirely new branches of production, creating new fields of labor, are also formed, as the direct result either of machinery or of the general industrial changes brought about by it” (Marx, 2010, p. 370), which drives up the demand for labor, and eventually offsets the negative impact of technological change on employment. In the same sense, Schumpeter (1983, p. 120), claimed that “the fundamental drive that starts up the capitalist machine and keeps it running comes from the new consumer goods, the new production and transport methods, the new markets, the new forms of industrial organization, which capitalist entrepreneurship creates." To Schumpeter (1983), technical progress is an essential cornerstone of creative destruction and innovation-which entail the construction of new plants or the refurbishment of old ones-and which are creators of benefits and investment opportunities, which, when taken advantage of by dynamic companies, lead to the creation of new jobs.
Although the empirical evidence on the impact of technological change on the employment level is abundant, studies differ from one another significantly in the following, among other factors: period of study, countries analyzed, level of analysis (macroeconomic, regional, sectoral, or microeconomic), and the diversity of variables and indicators used to measure technological change.
At the macroeconomic level, much of the empirical evidence suggests that in the long term, there is a negative correlation between productivity growth3 and the unemployment rate (Muscatelli and Tirelli, 2001; Benigno et al., 2015; Gallegati et al., 2014). Although the negative correlation between productivity and unemployment seems to be the rule, it has been documented that in certain time periods in certain regions of Western Europe, productivity gains have happened at the expense of jobs (Enflo, 2010). Gallegati et al. (2014) furnished empirical evidence suggesting that in the short and medium term, productivity gains spur unemployment.
Up until now, in the long term and very imperfectly, these offsetting mechanisms have managed to counteract the effects of technological change on the employment level, because neither technology in general nor computers in particular lead to permanent widespread unemployment (Frey and Osborne, 2013). Nevertheless, during transition periods (short and medium term), economies bear high unemployment rates due to, among other factors, the employment structure of the labor market, which changes faster than workers are able to change their skills (Levy and Murnane, 2004).
Among the major historical changes in the labor structure, changes due to economic activity sector and skill level stand out. At the sector level, employees have been displaced from agriculture and craftsmanship to manufacturing and office jobs, and subsequently, to service and management positions (Frey and Osborne, 2013). When it comes to skill level, there have been several patterns over time: 1) in the nineteenth century, technological change boosted the demand for unskilled labor (Levy and Murnane, 2004); 2) throughout much of the twentieth century, technology complemented skilled labor (Acemoglu, 2002); and 3) in recent decades, technology applications have been replacing medium-skilled labor (Autor, Levy, and Murnane, 2003; Levy and Murnane, 2004; Goos and Manning, 2007).
2. RECENT TECHNOLOGICAL BREAKTHROUGHS
Recent advances in artificial intelligence, machine learning, and robotics, alongside the constant decline in the costs of automation, derived from falling computer prices (which dropped 64% a year between 1980 and 2006, according to Nordhaus (2007)), and industrial robots (which dropped 10% annually, according to the International Robots Federation (2012, cited in MGI, 2013)), have created strong economic incentives for employers to replace human labor with computerized number control equipment (Frey and Osborne, 2013). The possibility that we are witnessing a radical technological change (once again) set off the alarm bells for technological unemployment, so in recent years, studies have multiplied as to the potential effects of automation on employment (Brynjolfsson and McAfee, 2011; MGI, 2017; Frey and Osborne, 2013; Chui et al., 2016; Jäger et al., 2016).
This burgeoning concern is in large part because until a little more than a decade ago, it was that that technology could only replace human labor in routine tasks (manual and cognitive) but not (yet) replace it in non-routine tasks (Autor, Levy, and Murnane, 2003; Goos and Manning, 2007). Even so, rapid technological change, as well as the fragmentation of productive processes, has been on the upswing with respect to the ability of technology to automate tasks, blurring the line between what can and cannot be automated. For example, Frey and Osborne (2013) signaled that in 2003, driving a car or reading a manuscript were considered non-routine tasks, but ten years later, they no longer were. Likewise, technology is moving forward in giant steps in automating non-routine cognitive tasks, like diagnosing cancer, which is now starting to be done by a computational algorithm developed by IBM (2017).
It is likely that as has happened in the past, in the long term, those offsetting mechanisms will manage to restore the employment level in the labor market. According to Levy and Murnane (2004), the main consequence of automation does not seem to be widespread unemployment, but rather a significant change in the employment structure. This is because although the impact of technological change on the employment level tends to be mitigated as time goes on, its effect on the labor structure is persistent, as even when employment opportunities continue to grow, they grow faster in skilled jobs, where computers complement human labor.
In recent years, two methods have been proposed to try to quantify the potential impact of technological progress on employment. The first, proposed by Frey and Osborne (2013) makes it possible to quantify the impact of technology by occupation, and to estimate the proportion of employment that could potentially be replaced by capital and new knowledge. This method exclusively looks at technical capabilities (technical feasibility, as of 2013). In other words, the technical capacity for automation already existed in those jobs.
Even so, it is important to clarify that this method does not pretend to estimate how many jobs will really be automated, which is to say, it does not estimate the real risk, because there are other economic, political, social, legal, etc. factors that limit the implementation of technological innovations, even when they are technically feasible. Nor is it possible to predict how quickly the automation will come about.
With this method, the authors estimate that approximately 47% of all jobs in the United States are at high risk of being automated. Following this method, a team from the World Bank (2016) studied the share of jobs susceptible to automation in over 40 developing economies; Bowles (2014) did the same for 28 European countries.
The McKinsey Global Institute (MGI) (2017) proposed a method breaking down occupations into activities. These authors believe it can be used to estimate the potential impact of automation on over two thousand activities. Comparing the estimates made by the two methods, it emerges that in several countries, like the United States, Sweden, and Czech Republic, the results are very similar. Nevertheless, in the vast majority of countries, the impact estimated with the Frey and Osborne methodology is higher, with the gap particularly wide in developing economies (MGI, cited in Chui et al., 2017; Bowles, 2014; BM, 2016).
In the case of Mexico, using the MGI method, Chui et al. (2016) estimated that 52% of total jobs and 64% of all manufacturing jobs are at risk of being automated. However, it is unclear how many of these jobs are at risk of being automated by industry and what the skill level is of the workers whose jobs are in jeopardy in the manufacturing sector, as well as which occupations are most vulnerable and which local factors might accelerate or slow down the adoption of automated solutions in Mexican manufacturing.
With the likelihood of automation by occupation calculated by Frey and Osborne (2013), we estimated the potential impact of technological change on employment in the manufacturing industries; identified the jobs most vulnerable to technology breakthroughs; and developed a skill level vs. automation risk matrix. Finally, we analyzed the main factors that can influence the speed at which the Mexican manufacturing industry adopts automated processes.
Although the estimate of the impact of technology on employment level by activity could be more precise, the Frey and Osborne method was chosen thanks to the availability of estimates of the likelihood/risk of automation in 702 jobs, because up until now these occupations were the greatest level of disaggregation available in statistical information on employment in Mexico.
3. DATA AND METHODOLOGY
The statistical information used in this study was fundamentally taken from the Frey and Osborne (2013) paper and the National Occupation and Employment Survey (ENOE) from third quarter 2016 (INEGI, 2016). In the appendix to their study, Frey and Osborne published a table listing 72 occupations, and the likelihood they would be computerized,4 even though the jobs are listed based on the Standard Occupational Classification (SOC), while Mexico’s employment statistics use the National Occupations Classification System (SINCO). In order to assign an automation probability to the occupations in Mexico, it was necessary to find matches between the two classification systems. As such, we used the SOC-SINCO comparison table provided by the National Statistics and Geography Institute (INEGI, 2012). Because this table uses the SOC system disaggregated to five digits and Frey and Osborne (2013) go to six, employment statistics from the United States (BLS, 2016) were used to weigh the sum of the probabilities.
To validate that the sum of the likelihoods would not significantly modify the results, the Frey and Osborne (2013) exercise was replicated with data from 2016 with the occupations disaggregated to 6 and 5 digits. It was found that with the information at 6 (5) digits, 51% (52%) of total employment was at high risk of being automated, 20% (19%) was at medium risk, and 29% (29%) was at low risk.
Because the two classification systems do not match up perfectly, to estimate the likelihood for the occupations that do not have a one-to-one match, the following methods were used: 1) a weighted probability was estimated using the United States employment statistics (assuming that the Mexican labor structure is similar to the American) and 2) the probability was estimated based on a simple mean (see Appendix 1). Comparing the estimates made with the two methods, a difference was found of approximately 3% in the share of jobs at a high risk of being automated in the total economy, and at least 1% in the manufacturing industry. For this paper, the difference between the values estimated with the two models did not prove to be significant in the case of the manufacturing sector (64.5 with the weighted probability and 65.3 with the simple probability), so the following sections of this paper report only the estimates made using the former method.5
According to the ENOE,6 the total employed population in Mexico as of the third quarter 2016 was approximately 52 million, with 8.5 million in the manufacturing industry. To perform the estimates for the total economy, we only considered 47.9 million people, and 7.4 million for the manufacturing industry, because 1) for some occupations, Frey and Osborne (2013) did not estimate the likelihood of automation and 2) the equivalence between the American and Mexican occupation classification system is not exact, so there are some jobs that simply do not match up.
Following the Frey and Osborne (2013) proposal,7 occupations were grouped by the level of risk that they would be automated: 1) high, for occupations with an automation likelihood higher than 0.7, 2) medium, for occupations with an automation likelihood between 0.3 and 0.7, and 3) low, for occupations with a likelihood less than or equal to 0.3.
4. RESULTS
According to our own estimates, 63% of total employment is at high risk of being automated (see Table 1), which according to Frey and Osborne (2013) means that with existing technology (as of 2013), there is a high likelihood (greater than 0.7) that the tasks involved in occupations at a high risk of automation can be done by computer-controlled equipment. The proportion of Mexican jobs at a high risk of being automated is higher than it is in the United States (47%), the European Union (54%), and the average of the Organization for Economic Cooperation and Development (OECD) countries (57%), but below that of Asian countries like China (77%) and India (69%) (BM, 2016; Bowles, 2014).
Table 1 Total Employment by Risk of Automation, Third Quarter 2016.
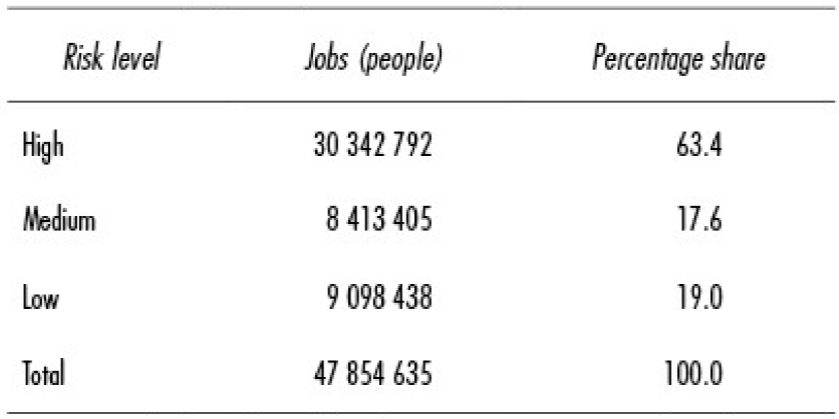
Source: Created by the authors with data from ENOE (INEGI, 2016), considering the automation likelihoods by occupation estimated by Frey and Osborne (2013).
On another note, estimates for the manufacturing industry suggest that approximately 64.5% of Mexican manufacturing jobs are at a high risk of being automated (see Table 2).
Table 2 Manufacturing Jobs by Industry and Level of Automation Risk, Third Quarter 2016.

aHousehold version.
Source: Created by the authors using data from ENOE (INEGI, 2016), considering the occupation automation likelihoods estimated by Frey and Osborne (2013).
Analyzing the potential impact of technology by industry, we found that there are three industries (timber, furnishings, and apparel) in which over 85% of jobs are highly likely to be automated. The timber and furniture manufacturing industries together represent just 5.8% of manufacturing employment, so the impact of automation there would be moderate. By contrast, advances in automation in other industries, like the food, transport equipment, apparel, and metal product manufacturing industries, would indeed entail a serious threat to employment, as together they account for over 50% of manufacturing jobs. Meanwhile, the greater adoption of automated processes in the transport equipment and electronics industries, in both Mexico and abroad, represents a major risk to Mexican manufacturing, as together, these two industries concentrate 17.8% of employment and over 60% of manufacturing exports.
Estimating the potential impact of technology on occupations in the manufacturing industry, it emerged that 117 of the 255 occupations comprising this industry are facing a high risk of automation. However, what is alarming is that 15 of the 117 account for over 70% of the jobs at risk of being automated.
The high concentration of workers in just a few jobs makes Mexican manufacturing employment particularly vulnerable to the march of automation (see Table 3).
Table 3 Occupations with the Greatest Number of Employees at a High Risk of Automation, Third Quarter 2016.

Source: Created by the authors using data from ENOE (INEGI, 2016), considering the occupation automation likelihoods estimated by Frey and Osborne (2013).
5. MITIGATION AND ADAPTATION MECHANISMS
Although the estimated potential impact of technological change on manufacturing employment is alarming, it emerged that market mechanisms, governmental policies, the speed and reach of technological change, and economic and social factors can all contribute significantly to mitigating technological unemployment, and help aid adaptation to the new employment structure.
Market Mechanisms and Investment Opportunities
Throughout history, during transition periods (short and medium term), economies have dealt with technology shocks via high unemployment rates. However, in the long term, market mechanisms have managed to restore the employment level in the labor market. As previously noted, technical progress not only destroys jobs but also creates opportunities for benefits and, therefore, investment. Moreover, technological progress renders capital goods, intermediate inputs, services, and workforce skills obsolete, opening up opportunities for new investments and new locations for that investment.8 If investments are taken by companies, new jobs will be created not only in the innovative sector but also in other sectors supplying them (goods and intermediate services), as well as complementary goods and services, and traditional sectors, thanks to rising income.
In recent decades, new products and services have arisen that have driven up output and labor productivity, generating new sources of jobs. Some examples of that include semiconductors, computing equipment, cell phones, ATMs, drones, industrial and service robots, cloud storage services, big data, social media, etc. According to the MGI (2017, p. 101), "one third of new jobs created in the United States in the past 25 years did not exist, or barely existed, just 24 years ago." Furthermore, several traditional industries are being reinvented, with a major impact expected on productivity, energy efficiency, quality, and employment.
Government Policies
Starting in the nineteen-thirties, the role of the state in creating jobs became vital, either via intervention in the so-called “traditional” areas, like education, healthcare, social security, and macroeconomic stability, or via industrial policy, wielding instruments like subsidies, trade protectionism, coordinated complementary and competing investments, policies to guarantee economies of scale, regulations on importing technology and foreign direct investment, venture capital and incubating for high-tech firms, export promotion, and foreign currency allocations (Chang, 2009) to foster the emergence and development of new and/or strategic industries. According to Shafaeddin (1998), with the exception of Hong Kong, no other country has developed its industry without governmental intervention, specifically, without policies designed to protect nascent industries.
Speed and Scope of Technological Change
The speed at which technological change appears is a key factor to determining its impact on the employment level. Fast and profound technological change challenges the economy’s ability to create new jobs for displaced workers, and the ability of workers to change their skills and adapt to the new labor structure. There are two opposing visions of the speed at which new technologies are being introduced nowadays: the first holds that the technological change of the present, compared to that of the past, is less profound and slower moving (Gordon, 2012), leading to low productivity, low growth in the product, and low employment growth. Hansen (1939) characterized this situation as "secular stagnation." The second maintains that the transformations derived from the incorporation of current digital technologies are profound, far-reaching, and fast-moving. As such, recent decades gave rise to very rapid productivity growth, ending millions of jobs, which suggests that we could be facing radical technological change whose adverse impacts are barely becoming apparent, as noted by Brynjolfsson and McAfee (2011).
Economic and Social Factors
Among the factors that affect the speed at which a new technology is adopted, once its technical feasibility has been proven, are the following: labor market conditions, the economic benefits derived from its adoption, and the industrial structure (MGI, 2017; BM, 2016).
Labor market conditions. The relative cost of human labor versus automated solutions affects the speed and degree to which new technology applications are adopted. In countries where labor is abundant and wages are low, it is likely that the high competitiveness of labor-intensive processes will delay the adoption of automation. By contrast, in countries with high wages, where the percentage of the population in working age is waning, the labor market conditions oblige them to pioneer the development and implementation of new technology applications (BM, 2016; MGI, 2017).
Having abundant labor and low wages is what has allowed Mexico to compete with production processes intensive in unskilled labor, which could potentially hold back the speed at which automated processes are adopted. Nevertheless, as will be seen later on, the economic benefits of automation are not limited to merely reducing labor costs, so in spite of low wages, the country may have industries (like the automotive industry) in which automation has made significant inroads.
It is also important to bear in mind that there is empirical evidence (Graetz and Michaels, 2015) suggesting that the negative impact of automated processes on employment (read, industrial robots) in the manufacturing industries is particularly adverse for less-skilled workers, and less so for medium-skilled employees. The high share of low-skill workers in total employment (58%) means that the Mexican manufacturing industry is extremely sensitive to the march of automation. According to our own estimates, 70% of low-skilled jobs (and 66% of medium-skilled jobs) are at a high risk of being automated; 28% (29%) are facing a medium risk, and only 2% (5%) have a low risk (see Table 4).
Tabla 4 Manufacturing Jobs by Skill Level and Automation Risk, Third Quarter 2016.

Note: Low-skilled is considered to be employees whit secondary school or less; medium-skilled refers to those who have completed high school or a technical vacational track; and highly-skilled means those with professional education or more.
Source: Created by the authors with data from ENOE (INEGI, 2016), considering the likelihoods of automation by occupation estimated by Frey and Osborne (2013).
Finally, implementing automated processes on a regular basis requires having specialized and highly-skilled technical personnel. But, in recent years, Mexican companies have signaled that they are seriously struggling to find skilled staff, which has led to a lot of open vacancies (Hays, 2013, cited in Forbes, 2014). Some of the top vacancies that employers have had difficulties to cover include: engineers, technicians, managers, and information technology staff (Manpowergroup, 2015). Failing to find enough people to fill these jobs restricts the country's capacity to adopt new technologies, putting it at a disadvantage with respect to its competitors.
The economic benefits of automation. Beyond reducing labor costs, automation generates economies of scale, so it boosts production, productivity, and gains for companies, reduces errors and increases the quality of products, diminishes technical shutdowns, and improves occupational safety. Thanks to all of that, analyzing the economic feasibility of adopting automated processes requires taking a look at all of its potential economic benefits and not limiting the scope to merely the effect on labor costs (MGI, 2017).
The Heckscher-Ohlin model states that if a country has a relative abundance of one factor, it can be more competitive in producing the goods that require a greater amount of that factor, so it is expected to specialize in producing and exporting that type of good. Pursuant to this model, with abundant labor and low wages, Mexico ought to specialize in labor-intensive goods and processes. Nevertheless, enormous gains in competitiveness, efficiency, and quality thanks to the automation of certain exhausting and repetitive tasks, especially in the automotive industry, have driven automation and digitization in the country in recent years, making it in 2016 ranked fourth on the list of users of industrial robots worldwide (Guthrie, 2017; El Financiero, 2017).
The industrial structure. Even as the degree of automation is vastly heterogeneous across industries, once the technical feasibility is overcome, it is very likely that automated processes will be incorporated even more rapidly in industries with highly concentrated markets. According to Jäger et al. (2016), this is because the largest companies have the most experience in introducing advanced production technologies and have larger economies of scale to make efficient use of industrial robot systems. Moreover, these companies have access to the large amounts of capital required to develop and implement new technology applications.
The high degree of Mexican manufacturing concentration could favor the rapid advance of automation. In 2013, 92% of manufacturing production was done by medium and large companies (see Table 5). Analyzing production by industry, the outlook does not change, as in 15 of the 21 manufacturing industries, medium-sized and large companies accounted for over 75% of production (see Table 5).
Table 5 Manufacturing Production by Industry and Company Size, 2013.

Sorce: Created by the authors based on information from INEGI (2013). Economic censuses.
The rapid march of automation, with a paucity of policies to mitigate its adverse effects, could be painful for the Mexican labor market, as over 50% of manufacturing jobs are found in just four highly concentrated industries (food, transportation equipment, metal products, and plastics and rubber), which-except in the case of the food industry-are some of the most robotized worldwide (IFR, 2015; Jäger et al., 2016).
6. CONCLUSIONS
Technological change boosts productivity and competitiveness, redefining production models and displacing human labor in activities where machines can get the job done more efficiently. Until a little over a decade ago, it was thought that technology would only be able to replace human labor in routine tasks, but (still) would be unable to supplant it in non-routine tasks (Autor, Levy, and Murnane, 2003; Goos and Manning, 2007). Nevertheless, rapid technological change and the advance of the fragmentation of productive processes have increased technology’s ability to automate tasks, blurring the border between what is and is not automatable.
This paper posed questions such as: what is the potential impact of technology change on manufacturing industry employment? What are the most vulnerable jobs? And, what is the relationship between skill level and automation risk? To answer these questions, we turned to the automation likelihoods per occupation estimated by Frey and Osborne (2013) and the ENOE from the third quarter of 2016 (INEGI, 2016). According to data obtained in the research, without looking at estimates of timeframes, approximately 64.5% of manufacturing jobs are at a high risk of being automated. The analysis by industry found that over 50% of manufacturing jobs are found in just four industries, three of which are robotized around the world (IFR, 2015; Jäger et al., 2016). Finally, a negative correlation was found between skill level and automation risk, as 70% of low-skilled jobs face a high risk of automation, while just 40% of high-skilled jobs are at high risk of automation.
Although the estimated potential impact of technological change on manufacturing jobs might seem terrifying, historically, imperfectly and at a very high human cost, industrial revolutions ushered in market mechanisms that have managed to offset the displacement of labor. Is it perchance possible that in this day and age, the offset would be different, given the expulsion of physical labor and the new demand for fundamentally cognitive work?
Recent decades have witnessed the rise of new companies with new products and services that have boosted labor productivity and production, thereby generating new sources of jobs, although primarily cognitive. In addition to new products and services, several traditional industries are being reinvented (like the automotive and pharmaceutical industries), with major impacts expected for productivity, energy efficiency, quality, and employment. Thus, the main consequence of automation seems to be a significant change in the occupational structure, with a constant decline in the demand for low- and medium-skilled labor, with a shift toward widespread unemployment as a consequence (Levy and Murnane, 2004).
ACKNOWLEDGMENTS
We would like to thank the research assistants Denhy Rodríguez Bautista, Alonso Lozano Tena, Carlos Briseño Larios, and Luis Amezquita Bobadilla for their contribution.
REFERENCES
Acemoglu, D. (2002), "Technical Change, Inequality, and the Labor Market", Journal of Economic Literature, vol. 40, num. 1, United States, American Economic Association. [ Links ]
Autor, D. H., Levy, F. y Murnane, R. J. (2003), "The Skill Content of Recent Technological Change: An Empirical Exploration", Quarterly Journal of Economics, vol. 118, num. 4, United Kingdom, Oxford University. [ Links ]
Banco Mundial (BM) (2016), "Digital Dividends", World Development Report. Recuperado de <http://documents.worldbank.org/curated/en/896971468194972881/pdf/102725-PUB-Replacement-PUBLIC.pdf> [ Links ]
Benigno, P., Ricci, L. A. y Surico, P. (2015), "Unemployment and Productivity in The Long Run; The Role of Macroeconomic Volatility", The Review of Economics and Statistics, vol. 97, num. 3, United States, Harvard University. [ Links ]
Bowles, J. (2014), "Chart of the Week: 54% of EU Jobs at Risk of Compu terization", Bruegel. Recuperado de <http://bruegel.org/2014/07/chart-of-the-week-54-of-eu-jobs-at-risk-of-computerisation/> [ Links ]
Brynjolfsson, E. y McAfee, A. (2011), Race against the Machine: How the Digital Revolution is accelerating Innovation, driving Productivity, and irreversibly transforming Employment and The Economy, United States, Digital Frontier Press. [ Links ]
Bureau of Labor Statistics (BLS) (2016), National Occupational Employment and Wage Estimates: United States. Recuperado de <https://www.bls. gov/> [ Links ]
Chang, H. J. (2009), ABCDE (Annual World Bank Conference on Development Economics). Recuperado de <http://siteresources.worldbank.org/INTAB-CDESK2009/Resources/Ha-Joon-Chang.pdf> [ Links ]
Chui, M., Manyika, J. y Miremadi, M. (2017), "The Countries Most (and Least) Likely to be Affected by Automation", Harvard Business Review. Recuperado de <https://hbr.org/2017/04/the-countries-most-and-least-likely-to-be-affected-by-automation> [ Links ]
______, Manyika, J. y Miremadi, M. (2016), "Where Machines could Replace Humans-and Where they can't (yet)", Mckinsey Quarterly [Anexo]. Recuperado de https://public.tableau.com/profile/mckinsey.analytics#!/vizhome/InternationalAutomation/WhereMachinesCanReplaceHumans [ Links ]
El Financiero (30 de julio de 2017), "México, cuarto lugar mundial en importación de robots industriales: OMC", El Financiero. Recuperado de <http://www.elfinanciero.com.mx/tech/mexico-cuarto-lugar-mundial-en-impor-tacion-de-robots-industriales.html> [ Links ]
Enflo, K. (2010), "Productivity and Employment-Is there a Trade-off? Comparing Western European Regions and American States 1950-2000", The Annals of Regional Science, vol. 45, num. 2, United States, Western Regional Science Association. [ Links ]
Forbes (2014), "¿Por qué a México le falta talento?", Forbes, marzo. Recuperado de: <https://www.forbes.com.mx/por-que-mexico-le-falta-talento/> [ Links ]
Frey, C. B. y Osborne, M. A. (2013), "The Future of Employment: How Susceptible are Jobs to Computerisation?", Working Paper: Oxford Martin Programme on Technology and Employment. Recuperado de <https://www.oxfordmartin.ox.ac.uk/downloads/academic/The_Future_of_Employment.pdf> [ Links ]
Gallegati, M., Gallegati, M., Ramsey, J. B., y Semmler, W. (2014), "Does Productivity Affect Unemployment? A Time-frequency Analysis for the US", en M. Gallegati y W. Semmler (eds.), Wavelet Applications in Economics and Finance, Germany, Springer. [ Links ]
Goos, M. y Manning, A. (2007), "Lousy and Lovely Jobs: The Rising Polarization of Work in Britain", The Review of Economics and Statistics , vol. 89, num. 1, February, United States, Harvard Kennedy School. [ Links ]
Gordon, R. J. (2012), "Is U.S. Economic Growth Over? Faltering Innovation Confronts the Six Headwinds", National Bureau of Economic Research, Working Paper 18315. DOI <10.3386/w18315> [ Links ]
Graetz, G. y Michaels, G. (2015), "Robots at Work", Center for Economic Performance. Discussion Paper No. 1335. Recuperado de <http://cep.lse.ac.uk/pubs/download/dp1335.pdf> [ Links ]
Guthrie, A. (12 de julio de 2017), "Trump Talk Aside, Auto Manufacturing and Automation are Booming in Mexico", MIT Technology Review. Recuperado de <https://www.technologyreview.com/s/608220/trump-talk-aside-auto-manufacturing-and-automation-are-booming-in-mexico/> [ Links ]
Hansen, A. H. (1939), "Economic Progress and Declining Population Growth", The American Economic Review, 29 (1). Recuperado de <https://www.jstor.org/stable/1806983?seq=1#page_scan_tab_contents> [ Links ]
IBM (2017), IBM Watson Healt: Watson for Oncology. Recuperado de <https://www.ibm.com/watson/health/oncology-and-genomics/oncology/> [ Links ]
International Federation of Robotics (IFR) (2015), World Robotics Industrial Robots 2014, International Federation of Robotics Statistical Department, Germany. Recuperado de <https://ifr.org/worldrobotics/> [ Links ]
Instituto Nacional de Geografía y Estadística (INEGI) (2016), Encuesta Nacional de Ocupación y Empleo (ENOE), tercer trimestre de 2016. Consultada en mayo de 2017. Recuperado de <http://www.inegi.org.mx > [ Links ]
______(2013), Censos económicos. Recuperado de <http://www.inegi.org.mx/est/contenidos/proyectos/ce/ce2014/default.aspx> [ Links ]
______(2012), Sistema Nacional de Clasificación de Ocupaciones (SINCO) (2011). Tablas comparativas. Consultado en mayo de 2017. Recuperado de <http://www.inegi.org.mx/est/contenidos/proyectos/aspectosmetodo-logicos/clasificadoresycatalogos/sinco.aspx> [ Links ]
Jäger, A., Moll, C., Som, O. y Zanker, C. (2016), "Analysis of The Impact of Robotic Systems on Employment in the European Union", Publications Office of the European Union 2012 Data Update. DOI <10.2759/516348> [ Links ]
Keynes, J. M. (2003) [1936], Teoría general de la ocupación, el interés y el dinero, México, Fondo de Cultura Económica. [ Links ]
______(1986) [1930], Ensayos de persuasión, las posibilidades económicas de nuestros nietos, España, Editorial Crítica S.A. [ Links ]
Levy, F. y Murnane, R.J. (2004), The New Division of Labor: How Computers Are Creating the Next Job Market, United States, Princeton University Press. [ Links ]
Manpowergroup (2015), Talent Shortage Survey, Manpowergroup. Recuperado de <https://www.manpowergroup.com/wps/wcm/connect/db23c560-08b6-485f-9bf6-f5f38a43c76a/2015_Talent_Shortage_Survey_US-lo_res.pdf?MOD=AJPERES> [ Links ]
Marx, K. (2010) [1867], El capital I. Crítica de la Economía Política, México, Fondo de Cultura Económica . [ Links ]
McKinsey Global Institute (MGI) (2017), "A Future That Works: Automation, Employment, and Productivity", McKinsey & Company. Recuperado de <https://www.mckinsey.com/mgi/overview/2017-in-review/automation-and-the-future-of-work/a-future-that-works-automation-employment-and-productivity> [ Links ]
______(2013), "Disruptive Technologies: Advances that will Transform Life, Business, and The Global Economy", McKinsey & Company. Recuperado de <https://www.mckinsey.com/business-functions/digital-mckinsey/our-insights/disruptive-technologies> [ Links ]
Minian, I. (1981), Progreso técnico e internacionalización del proceso productivo: el caso de la industria maquiladora de tipo electrónico, México, Ensayos CIDE. [ Links ]
Mokyr, J., Vickers, C. y Ziebarth, N. (2015), "The History of Technological Anxiety and the Future of Economic Growth: Is This Time Different?", The Journal of Economic Perspectives, vol. 29, num. 3, United States, American Economic Association. [ Links ]
Muscatelli, V. A. y Tirelli, P. (2001), "Unemployment and Growth: Some Empirical Evidence from Structural Time Series Models", Applied Economics, vol. 33, num. 8, United Kingdom, Routledge. [ Links ]
Nordhaus, W.D. (2007), "Two Centuries of Productivity Growth in Computing", The Journal of Economic History, vol. 67, num. 1, United Kingdom, Cambridge University. [ Links ]
Piva, M. y Vivarelli, M. (2017), "Technological Change and Employment: Were Ricardo and Marx Right?", Discussion Paper Series, num. 10471, Germany, Institute of Labor Economics (IZA). [ Links ]
Ricardo, D. (1959) [1817], Principios de economía política y tributación, México, Fondo de Cultura Económica . [ Links ]
Schumpeter, J. A. (1983) [1950], Capitalismo, socialismo y democracia I, España, Ediciones Orbis. [ Links ]
Shafaeddin, M. (1998), "How did Developed Countries Industrialize? The History of Trade and Industrial Policy: The Cases of Great Britain and the USA", United Nations Conference on Trade and Development (UNCTAD). Recuperado de <http://unctad.org/en/Docs/dp_139.en.pdf> [ Links ]
Standing, G. (1984), "La noción de desempleo tecnológico", Revista Internacional del Trabajo, vol. 103, num. 2, abril-junio, United States, Organización Internacional del Trabajo. [ Links ]
2Frey and Osborne (2013) conducted an extensive literature review of the historical relationship between technological progress and employment; on another note, Levy and Murnane (2004) did the same on the impact of technology on the labor structure.
3Productivity growth is taken as the explanatory variable, as diverse studies hold that productivity is the channel through which technological change impacts the job market.
4To estimate these probabilities, Frey and Osborne, alongside a group of machine learning researchers at Oxford University, subjectively assigned labels to 70 occupations pursuant to automation possibility: “0” (not automatable) or “1" (not automatable). Later, they developed an algorithm that, considering the labels assigned to the aforementioned 70 occupations, helped them predict the likelihood of automation for 702 occupations. A detailed description of the methodology is found in the original Frey and Osborne (2013) study.
6The ENOE is a survey administered every quarter to households, nationwide, and its results are limited to the population aged 15 to 98 years old.
7The Frey and Osborne (2013) method was implemented in the case of Mexico by Ángel Martínez Monroy, co-author of this paper.
8An earlier paper developed the theme of obsolescence in high-tech industries as a driver of new investments, the fragmentation of productive processes and the relocation of production abroad (Minian, 1981).
*We would like to thank DGAPA for the grant funding provided through PAPIIT project IN300117, which made this work possible.
Appendix 1

*n is the number of SINCO jobs that match the SOC occupations. In the first example, there is a 1:1 correlation, but in the second, occupation 19-305 matches up with two SINCO classifications, so for occupation 2132, the probability is only weighted with 50% of the 19-305 jobs.
Source: Created by the authors.
Received: March 20, 2018; Accepted: June 01, 2018