Introduction
The Brazilian free-tailed bat Tadarida brasiliensis is a small-size insectivorous bat with one of the widest distributions in the western hemisphere (Wilkins 1989). It occurs in an extensive variety of habitats, ranging from deserts to montane humid forest (Eger 2007), with the ability to forage from the ground up to 3,000 m (McCracken et al. 2008). In South America, only the subspecies T. b. brasiliensis is recognized (Schwartz 1955). Based on individuals from the east versant of the Andes and the subantarctic sub-region, Morales et al. (2018) suggest it apparently conforms to one genetic group. The potential distribution of the species showed it is strongly associated to the Andes (Escobar et al. 2015; Romero 2018), with few records in much of Amazonia (Eger 2007), and presumably absent in lowland forest ecosystems (Wilkins 1989).
In the Peru, this species has been reported in the Equatorial dry forest, the coastal desert, the steppe, the Yungas and the lowland forests ecoregions (Pachecoet al.2009). Due to the variety of occurrence records (Acha and Zapatel 1957; Solari et al. 2001; Zeballos et al. 2001; Mena and Williams 2002; Zelada et al. 2014; Aragón and Aguirre 2014), it is believed that this species presents a continuous distribution in this country (Eger 2007; Barquez 2018). However, the Andean Cordillera, is recognized as a great biogeographical barrier that limits the dispersal of many mammalian species, particularly in bats, between the Pacific and Amazonian versants and could drastically affect T.brasiliensis perceived distribution in the country (Koopman 1978; Patterson et al.2012). To date, no analysis of the influence of the Andes, or how environmental conditions, influence the distribution patterns of T. brasiliensis in Perú has been conducted.
Species distribution models (SDMs) are frequently used for assessing the potential distribution of species (Razgour et al. 2016), based on occurrence records and the environmental characteristics of these localities (Franklin 2010). The main assumption of the models is that the climate of these localities represents part of the environmental component of the species’ niche (Peterson and Soberón 2012), and therefore, it is possible to predict suitable areas for species presence. Determining most suitable areas could help us better understand possible geographical and environmental barriers for the species’ distributions, and also provide information on its likely dispersal limitations (Barve et al. 2011).
The aim of this study is to describe the distribution patterns of T. brasiliensis in Perú using species distribution models and explore the possible influence of the Andes. Here, we test the hypothesis that T. brasiliensis has a continuous distribution in Perú, including the Andes mountain chain. Additionally, we report novel records in the territory and highlight priority research locations for this species.
Materials and Methods
Occurrence data. Occurrence data were selected from 139 records gathered from the following sources: the collection of mammals, Museo de Historia Natural de San Marcos (MUSM; n = 51) in Lima, bat acoustic surveys (this work, n= 26), literature (Acha and Zapatel 1957; Zeballos et al. 2001; Pari et al. 2015; Morales et al. 2018; Portugal 2018; n = 30), the Global Biodiversity Information Facility (GBIF 2019; download on July 8, 2019; n = 11), and Vertnet (Constable et al. 2010; download on August 7, 2019; n = 22). In order to avoid sampling bias caused by spatial correlation (Razgour et al. 2016), localities used as occurrence data were manually chosen such that no two localities were separated less than 25 km, which is the approximate mean displacement of T. brasiliensis for foraging flights from their roost (Allen et al. 2009). For GBIF and Vertnet sources, only records from scientific collections were considered to avoid spatial bias in the distribution (Beck et al. 2014). The majority of the occurrence records (74 %) were dated after 1960. All records covered a temporal range from 1900 to 2019. See selected locality details in Appendix 1.
The acoustic surveys were performed in 23 localities distributed across eight departments of Perú: Ayacucho, Cajamarca, Ica, La Libertad, Lima, Piura, Puno and Tacna, from 2014 to 2019. Recordings were obtained by performing active monitoring in acoustic transects, using Pettersson ultrasonic detectors D240x and M500-384 plugged into digital recorders or mobile phone devices. Recording sessions were performed between 18:00 and 23:00 hrs, in rainless conditions, for one night per site (Kingston 2016). We selected pulses in which the search phase consists of a downward quasi-constant frequency component ranging from 30 kHz to 26 kHz, described as characteristic for this species (Gillam and McCracken 2007; Arias-Aguilar et al. 2018). The sound files are stored in the MUSM for further studies involving the development of an acoustic library for Perú.
Novel localities are reported using the political departments of Perú. For the biogeographical analyzes, we used the terrestrial ecoregions of the world (Olson et al. 2001) and the biogeographic regionalization of the Neotropical region (Morrone 2014).
Study area. Two types of geographical spaces were delimited: the background and the projection areas. The background area must offer a good coverage of the conditions within the species environmental tolerance (Anderson 2013), and at regional scales, using a specific area to model partial-niche produce more sensitive models (Razgour et al. 2016). Thus, we calculated a minimum-convex polygon based on our occurrence data (Brown 2014) and applied a buffer of 50 km. As projection area, we used the layer of the Peruvian territory obtained from Instituto Geográfico Nacional (IGN, www.idep.gob.pe), assuming that it represents an accessible area for this species (Barveet al.2011) and considering that climatic conditions within this area were well represented by the background area (Anderson and Raza 2010). All the geospatial operations were done using ArcMap 10.5.1 (Esri 2016).
Environmental data. Bioclimatic data from the global interpolated climate database WorldClim 1.4 were used (Hijmans et al. 2005) with a grid resolution of 30 arc-seconds (~1 km). We downloaded 15 variables representing current climate, but removed Bio 8, Bio 9, Bio 18 and Bio 19 because they can have spatial abnormalities (Escobaret al.2014). We masked these climatic rasters to the extent of the background area to generate background point. To avoid multicollinearity (Elith et al. 2011), a Spearman’s correlation test between variables were performed using the R package fuzzySim 3.0, to select variables with correlation values lower than 0.85 (Syfert et al. 2013). Also, we selected variables considering their relevance to the representative Peruvian climate (Schwarb et al. 2011), as well as their relationships with the occurrence of T. brasiliensis (Duff and Morrell 2007; Hristov et al. 2010).
Distribution model. We constructed an SDM using the maximum entropy algorithm Maxent 3.4.1 (Phillips et al. 2018). The background area was used to calibrate the model, and then projected to the Peruvian territory. For calibration, different models were built with 10,000 random background points and evaluated with spatial cross validation. We explored different complexity settings for Maxent: regularization multipliers in intervals of 0.5, ranging from 1.0 to 5.0, and feature class combinations L, Q, H, LQ, LH, QH, LQH, LQHP, and LQHPT, where “L” is linear, “P” is product, “Q” is quadratic, “H” is hinge and “T” stands for threshold (Radosavljevic and Anderson 2014). We performed the evaluation process with the spatial cross validation procedure “checkerboard2” (aggregation factor = 4) using the R package ENMeval 0.3.0 (Muscarella et al. 2014) with R 3.5.3 (R Core Team 2019). We evaluated variable importance with Maxent´s variable jackknife test (Phillips et al. 2006). Following Warren and Seifert (2011), the model selection was made based on the Akaike information criteria corrected for small samples (AICc) that provide a comparison of the most informative and parsimonious model (Razgouret al.2016). We use the metrics of the model with lowest AICc value and projected it using the Maxent cloglog transformation. Model evaluation details can be found in Appendix 2 and model response curves in Appendix 3.
Results
We found that T. brasiliensis is found from sea level (MUSM 4,793, 5,368; Lima department) to 4105 m (ROM 93863, 93868; Junín department) in Perú. In the Pacific versant, the species was found up to 3,149 m (via ultrasonic record), whereas on the Amazonian versant most records are in the Andean piedmont (>1047 m), and only two records are reported in the lowland eastern humid forest of Perú at 360 m (MUSM 6045 194441; San Martín department). With acoustic surveys, we reported for the first time its presence in Cajamarca, Piura and Ica departments. We recognized the occurrence of T. brasiliensis in the Desert and the Puna provinces of the South America transition zone, in the Ecuadorian province of the Pacific Dominion, and in the Yungas, the Ucayali and the Rondônia provinces of the South Brazilian dominion.
We selected five variables as most ecologically relevant and not highly correlated (Table 1). Then, from 81 computed models using these variables, we chose the model with the lowest AICc obtained with following parameters: LQ as features class combination and 1 as regularization multiplier (AUCtrain: 0.7836, AUCtest: 0.7211, OR10: 0.0912, AICc: 1073.82, ΔAICc: 0). Based on the evaluation results (Appendix 2 and 4), we observed that in models with more parameters, the average AUCtest increase, which means that these models are stricter than the others. But we also observed that AUCdiff and the OR10 increase with more complexity, what is typically related to overfitting in these more complex models. Our selected model presents less complexity but also the less overfitting in comparison with other models.
Table 1 Selected variables contribution. Selected bioclimatic variables and their contribution in the selected model.
Variables Contribution | ||
---|---|---|
Potential Distribution Model | Percent contribution | Permutation importance |
Mean Diurnal Range (Bio 2) | 57.4731 | 64.8406 |
Annual Mean Temperature (Bio 1) | 18.4447 | 28.2832 |
Temperature Annual Range (Bio 7) | 6.6362 | 6.8761 |
Precipitation Seasonality (Bio 15) | 3.3811 | 0.000 |
Annual Precipitation (Bio 12) | 14. 0648 | 0.000 |
Bioclimatic variables with the greatest influence in the distribution of T. brasiliensis were mean diurnal range of temperature (Bio 02) and annual mean temperature (Bio 01), which contribute to 64.8 % and 28.3 % to the model, respectively. Response curves of each variable (Appendix 3) showed that annual temperatures between 15 to 20°C present predicted values over 0.5, while temperatures over 10°C in mean diurnal ranges reduce significantly the predicted values of occurrence. The jackknife test showed that the variable with the highest gain when used in isolation was annual mean temperature, while the one which decreased the gain the most when omitted was mean diurnal temperature.
The SDM showed a slightly disjunct distribution for T. brasiliensis in Perú, where two different core areas with high suitability values (> 0.5) were obtained (Figure 1). The first area corresponded to the coastline ecosystems in the Pacific versant of the Andes, included in the Desert and Xeric Shrublands biome, where higher predictive values seem to be restricted to the Sechura desert and Tumbes-Piura dry forest ecoregions. The other area corresponded to Andean slopes ecosystems in the Amazonian versant, where higher predictive values were detected above 1000 m, in the Yungas and wet Puna ecoregions. In northern Perú, a moderately suitable area in the Huancabamba Depression region, connects the western and eastern suitable areas. The higher part of the Andes (above 4500 m elevation) exhibited low environmental suitability (< 0.2) for this species. Similarly, the lower Amazonian forest had low environmental suitability (< 0.4).
Discussion
It was previously reported that T. brasiliensis has a broad and continuous distribution in Perú (Wilkins 1989; Eger 2007; Barquez et al. 2015); however, based on our model, we reject that hypothesis. Our correlative model predicted that this species has a largely discontinuous distribution in the country, where two disjunct areas with high suitability values could be identified: the Pacific and Amazonian versants, separated by the Andes. Contrary to the model presented by Escobar et al. (2015), we identified highly suitable environmental conditions in the coastal and Andean slopes ecosystems. Furthermore, supporting Wilkins (1989), we found this species is mostly absent in lowland forest of the Amazonia.
Based on the localities of occurrence and the predictions of the model, we identified two areas in the Peruvian territory that have not been sampled enough or remain unassessed for this species: the Huancabamba depression zone and the eastern slopes of the central Peruvian Andes. In the Huancabamba depression zone, although the SDM showed moderate suitability (0.4 to 0.6), no specimens are known to occur in this zone or in the vicinity (less than 25 km). Thus, we consider that the Huancabamba depression zone remains poorly studied and requires increased focus on bat research priorities. On the other hand, the eastern slopes of the central Peruvian Andes -specially the zone between Junín and Pasco departments- where our model indicated moderate suitability (~ 0.6) is one the better surveyed areas in the country (Mena 2010; Arias et al. 2016). We believe that T. brasiliensis has yet to be detected in this geographic area, because most surveys have only used mist nests, a methodology with only moderate efficiency when in collecting insectivorous bats (Rydell et al. 2002).
Despite the information gaps, we consider that our model efficiently represents the environmental niche of this species in Perú, in great part because our occurrence data covered a great part of the territory. We accomplished this, by incorporating ultrasonic records which increased data occurrence in about 41 % (Appendix 1); therefore, we support recent suggestions that acoustic surveys contribute and complement traditional methodologies in studying insectivorous bat species (Hintze et al. 2019).
Our model estimated only a moderate suitability index (~ 0.6) in the dry forests of the Huancabamba depression. Although this zone was proposed as an important biogeographical barrier for montane species (Weigend 2004), this region was also suggested as an east-west corridor that allow gene flow in Sturnira erythromos and likely other species (Pacheco and Patterson 1992). We currently lack occurrence data for T. brasiliensis in this region, but considering the low elevation of the Andean in this area (Pacheco 2002), we propose that the species likely uses the depression to connect populations on the eastern versant with those of the western versant of the Andes. Future biological inventories in the area will test this hypothesis.
On the other hand, in central and southern Perú, our model revealed broad separation in the suitable environmental conditions for the potential distribution of the species. Due to the topographic characteristics and the extreme climate conditions in the higher elevations (Garreaud 2009), the Andes are considered as important biogeographical barrier for several groups of mammals (Patterson et al. 2012). However, the role of the Andes as a biogeographic barrier for bats has seen little debate since the pioneering works of Koopman (1978) and Pacheco and Patterson (1992), but it has been argued that only few bat species are capable of inhabiting high elevations (Patterson et al. 1998). Although T. brasiliensis has the ability to fly up to 3,000 m above the ground to forage (McCracken et al. 2008), our model suggest that this species is limited by extreme climatic conditions on the highest parts of the central and southern Andes, likely due to cold weather and permanent snow (Garreaud 2009). Additionally, we believe that biological factors could also influence its distribution in the higher elevations of the Andes (Krauel et al. 2018). For example, the diet of T. brasiliensis includes coleopterans, lepidopterans, hemipterans and dipterans (Lee and McCracken 2002; Alurralde and Díaz 2018), but the diversity and abundance of these insects declines with increasing elevation (Hodkinson 2005). Therefore, reduced prey availability could make it difficult for this species to inhabit the higher Andes.
Furthermore, our model also revealed high suitable conditions restricted to the deserts and Lomas ecosystems of the Pacific versant (Figure 1). As in other western bat species in Perú, such as Artibeus fraterculus (Patterson et al.1992) and Glossophaga soricina (Webster 1993), the homogeneous climatic conditions of this ecoregion (Rundel et al.1991) could have allowed the latitudinal dispersal of T. brasiliensis close to the coastline (Wiens and Donoghue 2004). However, the biogeographical processes by which this species arrived to these ecoregions are still unknown (Lim 2009). We believe that the Huancabamba depression is closely related to the distributional patterns in South America, but population-level phylogenetic studies of the individuals from the central Andean mountains and Pacific versant are needed to test dispersal hypothesis.
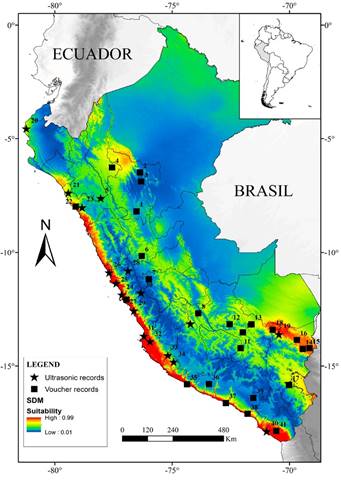
Figure 1 Selected localities and the potential distribution model (SDM) of T. brasiliensis in the Peruvian territory.
Based on our model, it could be expected that individuals from the eastern and western versant have accumulated some degree of genetic variation. Genetic differentiation between individuals from different versants has been reported for other Neotropical bats, resulting in some cases in the validation or description of new species, such as Lophostoma occidentalis (Velazco and Cadenillas 2011), Sturnira bakeri (Velazco and Patterson 2014), and Artibeus aequatorialis (Larsen et al. 2010). In North America, studies on intraspecific genetic variation for T. brasiliensis suggested that genetic structure is correlated with niche differentiation (Morales et al. 2016) and not with the currently recognized subspecies boundaries (Russell and McCracken 2005; Morales et al. 2016). Morales et al. (2018) found that individuals of T. brasiliensis from South America formed a unique population group based on the combined analysis of molecular and morphometric data; however, their study did not include representative data for populations from the Pacific versant of Perú or Ecuador. Thus, we highlight that a genetic survey for T. brasiliensis considering samples from both versants is badly needed.
In the Amazonian versant, our model reveals that lowland humid forests ecosystems present few suitable areas for the species (Figure 1). Although the distribution map of Wilkins (1989) seems to include a small part of Amazonia with this ecosystem, and Pacheco et al. (2009; 2015) stated the species was present in the lowland forest ecoregion (sensuBrack-Egg 1986), we found only two records in lowland forests (San Martin Department). In the apparent absence of east geographical barriers for bats in Perú (Proches 2006) and considering the ability of this bat to fly long distances (McCracken et al. 2008), the limitation of dispersal for T. brasiliensis in this ecosystem is probably related with climate conditions and/or biotic interactions (Peterson and Soberón 2012). The climate in lowland Amazonian forests is characterized by high precipitation and temperature (Vieira et al. 2004), with remarkable seasonal variation in the southeast (Jenkins 2009). Since our models revealed that the potential distribution of T. brasiliensis is highly influenced by mean diurnal range and annual mean temperature, and considering that ambient temperatures affect metabolic rates in bats (Reichard et al. 2010), it is likely that high temperatures in this ecosystem are one of the most important limiting factors for the species. Although it was documented that T. brasiliensis prefers temperatures between 22 to 32 °C to develop optimally (Herreid 1967), response curves of our model showed that suitable values of temperature vary between 15 to 20 °C in the Perú; higher temperatures may generate hyperthermia in this bat, limiting its dispersal and supporting what we stated.
Additionally, in the Amazonian forests, there are other possible limiting factors. The high plant diversity (Cardoso et al. 2017) and dense coverage that characterize the lowland tropical forest (Nogueira et al. 2005; Nabe-Nielsen 2001) could limit the open-space flight of insectivorous bats such as T. brasiliensis (Fenton 1990; Kalko 1997). In addition, it is reported that this species usually roosts in caves or human dwellings (Allen et al. 2009), which are scarce in this type of forest (García-Rosell 1965; Kvist and Nebel 2000); thus, potential roosting sites may represent another factor that restricts the niche of T. brasiliensis in this territory.
In general, our model suggests T. brasiliensis is mainly associated with deserts and Andean slopes ecosystems (Figure 1). Further, a great number of locality records in South America and previous work on potential distributions support this pattern (Escobar et al. 2015). However, due to the difficulties in capturing T. brasiliensis with conventional methods (Rydell et al. 2002), we highlight the importance of incorporating acoustic records proceeding from reliable sources to increase the number of records and therefore to improve modelling precision, especially when certain Andean and Amazonian regions remain poorly studied. Notably, the ecological mechanisms associated with geographical dispersal in South America and potential speciation in widespread species, such as T. brasiliensis, has yet to be completely investigated (Pyron and Burbrink 2009).